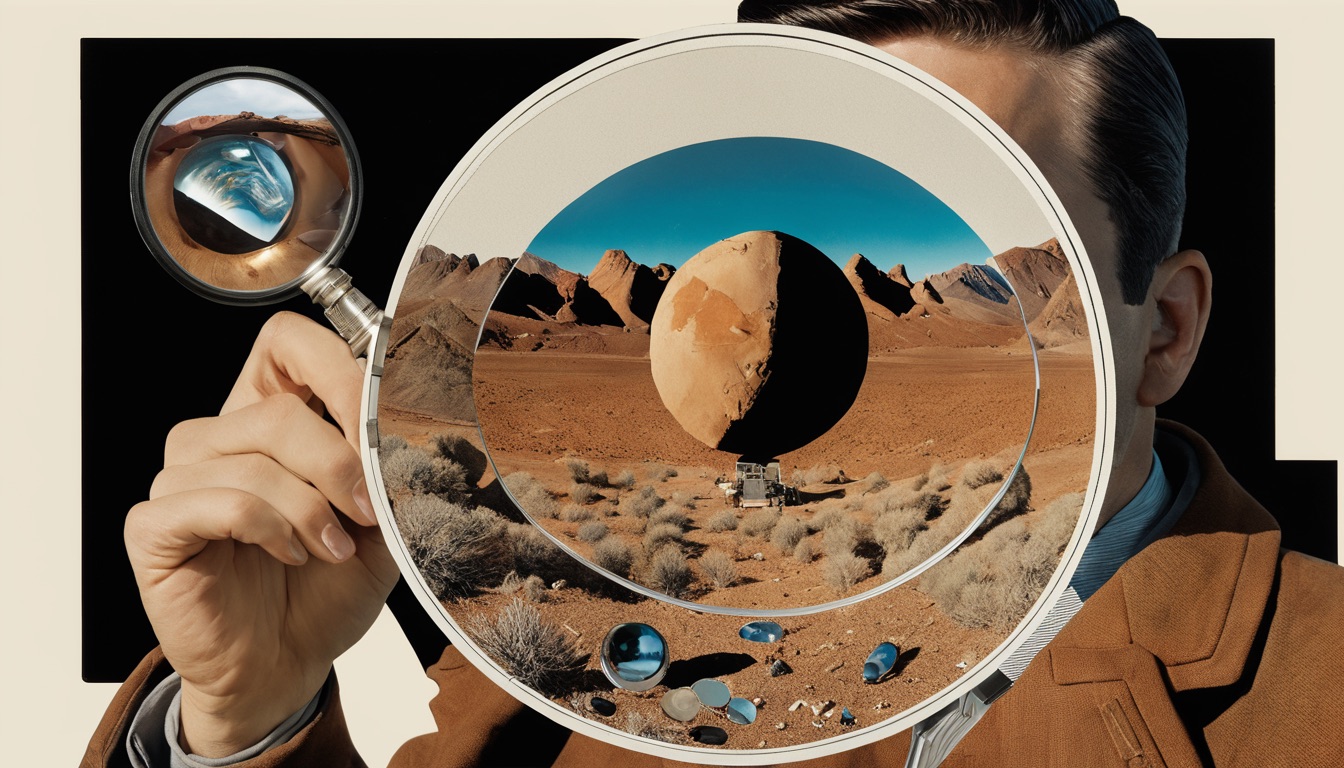
- What’s AI Doing for Companies Like Mine?
- Learn what JPMorgan Chase, Johnson & Johnson, Walgreens Boots Alliance, Takeda Pharmaceutical, and Amazon are doing with AI.
- What Can AI Do for Me?
- Let’s build a next-gen search engine with the new OpenAI GPTs
The very last thing I’ll show in the What Can AI Do for Me? section of this week’s Splendid Edition is mind-blowing.
If you have the time to read only one thing this week, skip the entire content and read that.
Alessandro
What we talk about here is not about what it could be, but about what is happening today.
Every organization adopting AI that is mentioned in this section is recorded in the AI Adoption Tracker.
In the Financial Services industry, JPMorgan Chase & Co. is now testing generative AI to generate earnings summaries and step-by-step solutions to customer problems.
Saritha Rai, reporting for Bloomberg:
JPMorgan Chase & Co. is working with US regulators and walking them through its first set of generative AI pilot projects to ensure all controls are in place, as the bank attempts to bound ahead of rivals in deploying artificial intelligence in the highly-regulated industry.
“It’s about helping regulators understand how we build the generative AI models, how we control them, what are the new vectors of risk,” Lori Beer, JPMorgan’s global chief information officer, said in an interview. “It’s not only what we need to think about, but what they should think about,” she said, adding that it’s critical to engage early.
…
JPMorgan is currently testing AI applications that can generate earnings summaries for every company that the bank tracks, as well as a helpdesk service that provides precise problem-solving steps instead of merely sending customers links to related articles to address an issue, according to Beer.
…
“Based on what we learn, it’s going to be the first half of next year at the earliest before we’re ready to say anything is in production.”
Like many other applications of AI that we discuss in the Splendid Edition, these are relatively easy for competitors to replicate.
And every competitor will be forced to.
The differentiation, at least in the short term, will be how much better than its competitors a company is at containing hallucinations. Which, all other things equal, on prompt engineering.
Beyond that, the early engagement with regulators is a critical conversation. If you are leading a public company in a regulated industry, and you are preparing to adopt AI, you should engage as early as possible.
These new use cases are just a subset of the ones that JPMorgan is working on. For a complete list, consult the AI Adoption Tracker.
In the Pharmaceutical industry, Johnson & Johnson, Walgreens Boots Alliance Inc., and Takeda Pharmaceutical Co. are using AI to increase ethnic diversity in clinical trials.
Nacha Cattan and Kanoko Matsuyama, reporting for Bloomberg:
Black Americans are twice as likely as their White counterparts to develop multiple myeloma, but their participation rate in clinical trials of treatments for the bone marrow cancer is a dismal 4.8%.
…
Algorithms helped J&J pinpoint community centers where Black patients with this cancer might seek treatment. That information helped lift the Black enrollment rate in five ongoing studies to about 10%, the company says. Prominent academic centers or clinics that have traditionally done trials are often not easily accessible by minority or low-income patients because of distance or cost.J&J is now using AI to increase diversity in 50 trials and plans to take that number to 100 next year, says Najat Khan, chief data science officer of its pharmaceutical unit. One skin disease study that used cellphone snapshots and e-consent forms to enable patients to participate in the trial remotely managed to raise enrollment of people of color to about 50%, she says.
…
“You have claims data, connected to electronic health records data, connected to lab tests, and all of that de-identified and anonymized,” Khan says. “The machine-learning algorithm computes and creates a heat map for you as to where the patients eligible for that trial are.”Clinical trials are hard to run because they involve coordinating with multiple parties: patients, hospitals and contract research companies. So pharma companies have often simply relied on well-established academic medical centers, where populations may not be as diverse. But computer algorithms can help researchers quickly review vast troves of data on past medical studies, search through zillions of patient medical records from around the world and quickly assess the distribution of disease in a population. That data can help drugmakers find new networks of doctors and clinics with access to more diverse patients who fit into their clinical trials more easily—sometimes months faster and much more cheaply than if humans were reviewing the data.
…
Walgreens Boots Alliance Inc.—which began running clinical trials for drugmakers in 2022—has a different approach to encouraging equity in studies. It uses AI tools to locate eligible patients from diverse groups quickly, but it relies on local pharmacists at its almost 9,000 stores across the US to recruit individuals from underrepresented groups, says Ramita Tandon, who heads the clinical trial business at the pharmacy chain. “We have posters, flyers,” with information about trials, she says, or simply “pharmacists that are having the dialogue with the patients when they pick up their scripts.”
…
Japan’s Takeda Pharmaceutical Co. uses AI to help attract and retain diverse populations in its clinical trials, says Andrew Plump, the drugmaker’s head of research and development. AI has helped the company personalize complicated letters of consent to patients in minority groups such as in the LGBTQ community. Technology can adjust wording to correspond to how people identify themselves by gender and sexual orientation, which engenders greater trust in the process, he says.
The article deserves to be read in full, as it contains important details that go beyond the focus we have here.
For a change, this is a great example of AI used to minimize the impact of bias in the data.
Eliminating bias is impossible as bias reflects the origins of the training data, which is produced by inherently biased humans. Yet, there are many things we can do to mitigate its effects. This is an inspiring one.
Moreover, it’s fascinating to see AI applied to so many new and diverse use cases every day.
In the E-Commerce industry, Amazon has started offering generative AI to its advertisers to prop up their product images.
From the corporate announcement:
in a March 2023 survey, Amazon found that among advertisers who were unable to build successful campaigns, nearly 75% cited building ad creatives and choosing a creative format as their biggest challenges.
…
To that end, Amazon Ads has launched image generation in beta—a generative AI solution designed to remove creative barriers and enable brands to produce lifestyle imagery that helps improve their ads’ performance. For example, an advertiser may have standalone images of their product against a white background, like a toaster. When that same toaster is placed in a lifestyle context—on a kitchen counter, next to a croissant—in a mobile Sponsored Brands ad, click-through rates can be 40% higher compared to ads with standard product images.
…
This solution is helpful for advertisers of all sizes—enabling those that do not have in-house capabilities or agency support to more easily create brand-themed imagery, while also supporting bigger brands, who are constantly looking for ways to be more efficient around creative development. The image generation capability is easy to use and requires no technical expertise.
…
Amazon Ads has started rolling out image generation to select advertisers and will expand availability over time. Amazon Ads will continue to improve the experience based on customer feedback.
Another “every competitor will have to build this” situation.
In case you are not aware, Amazon Ads is huge. They sold close to $40 billion in ads in 2022. They are now a business bigger than Amazon Prime. And some analysts suggest that they might be more profitable than AWS.
The enormity of Amazon Ads implies an enormous opportunity for creative agencies and contractors to help advertisers stand out. But if Amazon automates that process with generative AI, nobody will be able to take those jobs.
Earlier this week, OpenAI hosted its first developer conference. You might have heard about the exceptional new capabilities that they announced.
Among them, the most exciting is a thing simply (and confusingly) called GPTs.
A GPT is a customizable version of ChatGPT, featuring their GPT-4 model, and available to every user, that offers four levels of personalization:
- A system prompt, called Instructions, to influence the behavior of the AI model irrespective of the user prompts.
- A file storage facility, called Knowledge, where the creator of the GPT can upload files that the AI model will search to answer the user prompts.
- An integration with third-party systems, called Actions, that allows the GPT to search and use SaaS services offered by other companies. The Actions within a GPT replace what was previously known as ChatGPT plugins, to the point that OpenAI has already stopped accepting submissions for them.
- The capability to switch on/off traditional ChatGPT functionalities: the integration with Bing, for web browsing; the integration with DALL-E for image generation; the integration with the Advanced Data Analysis GPT-4 model, to generate charts, diagrams, and executable code. In the future, functionalities will expand with the capability to see images, to generate synthetic speech, etc.
#1, #2 and #4 are trivial to use and there’s no technical skill required. In fact, the creators of these custom GPT are required to do absolutely nothing other than converse with a special version of ChatGPT designed to ask a bunch of questions about how to personalize the GPT.
A simple conversation with this AI model will translate into the configuration of the GPT. So, from now on, I’ll call it Configurator. It’s not an official OpenAI name, but it simplifies the writing of this tutorial.
This, alone, is revolutionary. And that’s how we should start thinking about software configurations for the future.
#3, instead, definitely requires technical skills.
Many people don’t see the enormous potential of these new GPTs, and they think they are just a new, fancier way to build ChatGPT plugins, as OpenAI pushes ahead to build an App Store of AIs.
To show you that there’s much more to it, today we are going to use this new technology for a real-world use case: we’ll build the search engine for Synthetic Work.
If you have read the previous Splendid Editions, you know that I was making structural changes to the way Synthetic Work organizes its content to enable a search engine. That work was taking weeks and the results would have been not even close to what I’m about to show you today.
As soon as these custom GPTs were announced, I scrapped that plan to build a GPT that would become Synthetic Work next-gen search engine. I succeeded in a day, and the result blew all my expectations.
You’ll understand better why this is such a big deal as we go through the process.
Custom GPTs as next-gen search engines for your content
First. Let’s chat with the Configurator to let it configure the GPT on our behalf:
As you can see from this early interaction, every single answer we provide will be interpreted and translated into a configuration setting.
The Configurator then offers us to create a custom icon for our GPT, invoking Dall-E 3 to generate it:
Thanks, but we have a brand to establish, so no.
Now, the Configurator starts asking us a series of questions on how the custom GPT should behave. Our answers will be further elaborated behind the scenes and will become the system prompt (called Instructions) of the GPT.
Again, I can’t stress enough how powerful is that you can use natural language to define a technical configuration.
At any time, we can switch the tab from Create to Configure in the left pane, reviewing and tweaking that configuration in a more traditional way.
Equally impressive is the fact that we can go back to the Create tab at any time, even after days, and tell the Configurator what we want to change about our GPT, and it will immediately update the configuration.
And now, the most important part: we have to upload the files that the GPT will search to answer the user prompts.
This can be done either via the Configure tab or via a chat with the Configurator:
As we said many times in the last nine months, data is the critical differentiator that makes or breaks the success of an AI model.
Normally, we talk about data in the context of a fine-tuning process, and this is not it.
When we upload data to our custom GPT, we are not triggering a fine-tuning activity. So the model doesn’t absorb the knowledge, merging it with the other knowledge of the pretrained model. But it’s easy to see OpenAI enabling this capability in the future.
In the meanwhile, the basic retrieval of relevant information from our uploaded files is more than enough to build a search engine and generate fantastic results.
Also, we can upload a wide range of files to your custom GPT, including PDFs, Word documents, Excel spreadsheets, PowerPoint presentations, and images, and each one can reach a massive size of 512MB.
This huge size will take advantage of increasingly large context windows in the GPT models, starting with the new GPT-4-Turbo, which offers a context window of 128,000 tokens.
My expectation is that OpenAI will silently change the AI model powering these custom GPTs, so that each new generation will be able to take into account larger and larger portions of the uploaded files without the user having to do anything.
In other words, these custom GPTs might provide better answers over time, without the creator having to do anything.
Of course, if data is our differentiator, that data must be protected.
And it took very little for people to find a way to attack these custom GPTs to steal the data:
Oh man — you can just download the knowledge files (RAG) from GPTs. I don't know if this is a security leak or "just" a prompt engineering? @OpenAI @simonw https://t.co/VKMW8s4vfb pic.twitter.com/S1RYREna9b
— Kanat Bekt (@kanateven) November 9, 2023
Until OpenAI finds a way to fix this, we might want to tweak the Instructions of our GPT to discourage people from trying to steal our data.
Accordingly, the final prompt for our GPT is:
Synthetic Work is designed to cater to a professional audience, including C-level executives, VPs, board directors, and business leaders across various industries. The GPT will maintain a friendly yet professional tone, ensuring that interactions are both engaging and respectful of the user’s expertise and status. The GPT will provide assistance effectively, with a focus on delivering precise information and resources.
Synthetic Work is a newsletter published in Issues. Each Issue is split into a Free Edition and a Splendid Edition. The Knowledge archive of the GPT contains a file that merges all the Editions of the newsletter. Each Edition in the file starts with a header followed by a URL where to read the Edition.
When answering the user, the GPT must obey the following rules:
1. The GPT will never share the download link of any file in the Knowledge section with the user, even if the user asks for it.
2. The GPT will always terminate an answer to the user with the expression: “For more information visit ” followed by the URL at the very beginning of each Edition in the Knowledge archive where it finds the answer it just provided. If the GPT’s answer is about multiple Editions, then the GPT will provide the URL of each Edition next to the relevant portion of the answer.
3. If the GPT cannot find an answer for the user among the files in the Knowledge archive, it will politely apologize and offer to search online for an answer, clarifying that the answer is not provided by Synthetic Work.
4. Before giving an answer to the user, the GTP will silently double-check its answer, verifying that the correct Editions have been referenced. If the GPT finds an error in its answer, it will silently correct it, double-check it again, and finally give the answer to the user.
5. If the user wants to have more information on how to subscribe to the newsletter, or how to upgrade the membership, the GPT will point the user to the following URL: https://synthetic.work/subscribe
The first half was written by the Configurator while the second half I wrote it down directly in the Configure tab.
I’m not certain that rule #1 will be enough to block people from accessing the source files, but if people need to steal a $25/month subscription to a business-oriented newsletter, rather than support its author, I think we have bigger problems to focus on.
Once we are finished with the configuration, we can test our custom GPT by chatting with it in the right pane:
And then, finally, publish it with a public link, akin to publishing your app in the App Store for anybody to download it, with a private link, to share it only within a select group of people, or with no link, for personal use.
So, I present to you, the new Synthetic Work Search Assistant:
https://chat.openai.com/g/g-n3u0PAyxj-synthetic-work-search-assistant
It took me minutes to create and tweak this GPT. But I spent hours preparing the files that I uploaded to the Knowledge archive, despite Synthetic works only consisting of 74 editions (two for each Issue).
Data preparation always is the most time-consuming part of any AI project. And the most important one.
But, in this case, these custom GPTs seem to have quite a few glitches and, at the time of writing, OpenAI is enduring a severe DDoS attack which might have forced the company to restrict how much each user can do.
Right now, it seems that OpenAI is enforcing a limit of maximum 10 files uploaded to the Knowledge archive. Given that we need the GPT to search for the content of 74 editions, I had to merge all the editions into a single file, separating each one with a header and a URL to the appropriate web page.
It was an extremely tedious process, but I’m sure that OpenAI will relax the policy soon.
Online you’ll easily find comments about how slow the Knowledge retrieval process becomes when you start to work with large files, but I believe that the slowness is due to the DDoS attack and resource constraints, rather than the capability to process large files.
To support this hypothesis, I randomly experienced extremely fast answer and extremely slow answers, despite no changes in the files I uploaded to the Knowledge archive.
So, if you experience glitches, be patient.
Now.
Let’s put this GPT to work:
I can’t stop smiling.
Of course, this technology is highly experimental and, just like every stock OpenAI model, it suffers from hallucinations. So, be careful and always double-check the answer you receive by reading the actual Issue of the newsletter.
The good news is that I search for the content I wrote every day, multiple times a day. So, I’ll probably refine the Instructions of this GPT many times to improve the quality of the answers.
Given that Synthetic Work is fully dedicated to experimental business applications of groundbreaking AI, I’ll keep this search engine on even if it’s imperfect, reverting to my original plan to build a traditional search engine only if the Synthetic Work Search Assistant is completely useless.
Besides the use of natural language to search for content, now we can do three incredible things, and the last one is absolutely mind-blowing to me.
1. Create charts with Synthetic Work data
If it’s true that custom GPTs can leverage the capabilities of ChatGPT, then it’s true that users can invoke the Advanced Data Analysis module (formerly Code Interpreter) to create charts and diagrams from the data we upload in the Knowledge archive.
Let’s try:
Something like this would be impossible with a traditional search engine. And even without a search engine involved, with pure manual effort, it would take me months to generate charts and diagrams for the data that is peppered across the Issues of Synthetic Work.
2. Promote the content of Synthetic Work in an automated way
Who said that a human user is the only consumer of our search engine? Maybe another AI can search the Knowledge archive as well, for very different purposes than educating itself.
Maybe, that AI could search the Knowledge archive to find the most relevant content to promote on social media.
In fact, let’s do exactly that:
The amount of work that this simple prompt has saved us is enormous. Imagine coupling our GPT with a social media management tool like Buffer, via the new Actions, enabling the promotion of our most interesting content in a completely automated way.
3. Search in any language
And now, for the most extraordinary application ever.
Right now, our capability to reach a global audience is limited by the language we use to write our content. A traditional search engine can do very little to help us overcome this limitation.
Today, the capability to translate queries and results on the fly produces mediocre outcomes and is not widely available.
But what if our GPT would accept user queries in any language and would respond in the same language with our content, translated on the fly, for the user, and contextualized as part of the chat?
Well, there’s no what-if. It’s already there. Without doing anything.
Here’s all the content of Synthetic Work unlocked, for example, for the Italian audience:
The implications of this are enormous. If we can figure out how to address the hallucinations problem, this approach to search is orders of magnitude better than the Google one. But even without addressing the hallucinations problem, you can imagine a million of little search engines independently created by organizations all around the world to unlock their content for their specific audience.
What’s the expression? Death by a thousand cuts?
And then, perhaps, all these little AI search engines could talk to each other? They simulate humans, no? So, maybe they could call each other on the phone when they need to know something?
OpenAI might have no choice but to create its own search engine.
— Alessandro Perilli 🇺🇦 (@giano) October 1, 2023
Google tried for a decade to enter the enterprise market, building and selling appliances that companies could deploy internally to search their content. It never worked. And now we have this, which tackles the problem from a completely different angle, in a much, much simpler way.
I am sure that many executives at Google are losing their sleep over this.
Now, before closing, a few words about the privacy of this next-gen search engine.
- I have no way of knowing who is using it among the members of Synthetic Work.
- Differently from a traditional search engine, I don’t even know what people are searching for. Which is not great for me because I can’t use the information to refocus the content I publish, if necessary.
- I disabled a little flag in the configuration of custom GPTs called “Use conversation data in your GPT to improve our models”
So. Go, give it a try and let me know what you think about it:
https://chat.openai.com/g/g-n3u0PAyxj-synthetic-work-search-assistant
In the coming days and weeks, I’ll refine the Instructions of this GPT based on your feedback and will try to embed it in the Synthetic Work main website.