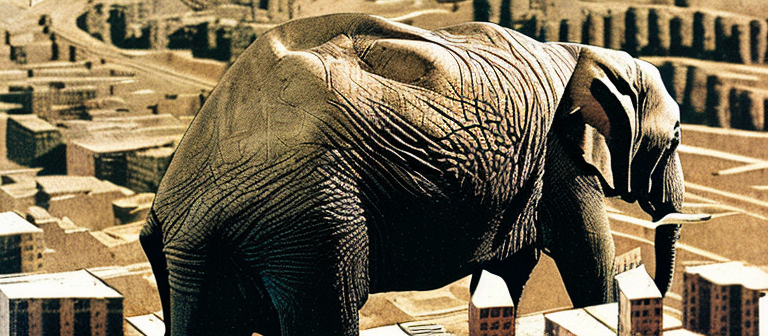
- New research might revolutionize how much AI can remember for every conversation. And that completely changes the things that we can do with it.
- Do you have a unique value proposition? If you don’t, your audience might just prefer AI-generated content
- Just Eat is having a great time using automated dismissal technology
- A Fortune company gave its customer service reps generative AI to help them answer customers’ calls. Performance increased noticeably.
- While Drake’s music label is busy suing to death his fans, Grimes proposes her fans to split the profit if they use her voice to make new songs
- People reveal secrets they don’t tell anybody else to their AI
- Why bother with dating apps when the AI can do it for you? Just show up to the restaurant that GPT-4 has booked for you with the partner of the day.
P.s.: This week’s Splendid Edition of Synthetic Work is titled The Fed Whisperer. It covers how Coca-Cola, Walmart, and JPMorgan Chase & Co are using AI for a very different range of use cases. It also reviews three powerful prompting techniques to reduce the number of tokens (and the bill) in your interaction with GPT-4.
As you all know, your Synthetic Work memberships are managed by a company called Memberful.
They reached out and asked if they could write an article about why I created Synthetic Work and what it’s all about.
We worked together and this project ended up becoming a two-part story. The first part is here:
https://memberful.com/meet-our-customers/synthetic-work/
Memberful has been one of the best business partners I ever had and it has been from day 1.
Alessandro
Without question, the most important thing that happened this week, equivalent to a tectonic shift in the AI world, is this: researchers have found a way to extend the “memory” of AIs like GPT-4 two orders of magnitude compared to what’s available today.
What does it mean in non-technical language and why does it matter so much to you?
The wonderful people that have read Synthetic Work since the beginning may remember that we already talked about this “memory” that AI experts call context window in Issue #2 – 61% of the office workers admit to having an affair with the AI inside Excel.
If you are too lazy to go there and read it, let’s refresh our memory here.
In a very loose analogy that could cost me imprisonment, the context window of an AI like ChatGPT or GPT-4 is like the short-term memory for us humans. It’s a place where we store information necessary to sustain a conversation (or perform an action) over a prolonged amount of time (for example a few minutes or one hour).
Without it, we’d forget what we were talking about at the beginning of the conversation or what we were supposed to accomplish when we decided to go to the kitchen.
The longer this short-term memory, this context window, the easier is for an AI to interact with people without repeating or contradicting itself after, say, ten messages.
Right now, the context window of GPT-4 is not very long, which is why extended chats on the OpenAI chat interface, the Bing interface inside Microsoft Edge, or any other application that connects with OpenAI, end up in very weird places.
Very soon, OpenAI will unlock the possibility to use a version of GPT-4 with a context window four times larger than what the current version sports.
Everybody is waiting for this moment as it will have huge implications in terms of productivity. With a short-term memory four times larger, at the beginning of each conversation you can, for example, feed the AI a corporate guideline PDF of multiple pages and then order with your prompt: “Write a blog post about topic X being sure that it follows all the rules in the document I just shared with you.”
There are many other examples, which we explore in the new Prompting section of the Splendid Edition of Synthetic Work, but throughout this story, I’ll stick with this particular example.
So, the thing is game-changing. And the few lucky ones that got preview access to this new version of GPT-4 are already raving about it (but people rave about everything AI-related these days, so we’ll see).
Now that you have this context in mind, here’s why it matters to understand the big news of the week.
The current GPT-4 context window is big enough to fit 8,000 tokens (approximately 6,000 words, as 100 tokens ~= 75 words).
The imminent GPT-4 model’s context window is big enough to fit 32,000 tokens (almost half a book of average size).
The new technique discovered by researchers allows AIs to have a context window as big as 2M tokens (the entire Harry Potter book collection is 2.5M).
If this works as intended, instead of a scenario where you will be able to ask GPT-x (or another AI) to write a long blog post that follows the corporate guidelines, you might be able to ask GPT-x, in a single request, to write enough corporate blog posts for 1 year, all related to each other, all consistent in terms of language, and all following an overarching corporate narrative defined by your prompt.
If this scenario becomes a reality, the implications on how we work and our jobs are deep and it’s really time we all start thinking about this seriously.
What does it mean for both companies and writers if with a push of a button, instantaneously, you can generate enough high-quality (coherent, consistent, engaging, funny, accurate, etc.) content to be satisfied for 12 months?
Sure, existing writers will still have to review what the AI has generated and correct and tweak it where necessary. But there’s an enormous difference in terms of workload between a company that needs to write content for 12 months and a company that only needs to review content for 12 months.
Are companies going to hire the same number of writers? If they do, and each and every one of them is armed with a GPT-x with a 2M token context window, they will generate content that nobody will be able to read. AI might be getting capable of infinite high-quality content production, but humans won’t be capable of infinite high-quality content consumption.
Even if want to invoke the Jevons paradox, the human brain has physical limits that cannot be overcome until we reach mass adoption of neural interfaces, which is many, many years into the future.
To me, it seems more likely that companies will work to increase content quality, not content production. But the former is about improving the data used to train the AI, not about retaining the same number of writers they have today to multiply their output.
On the other side of the equation, what happens to the writers?
For a growing number of jobs, AI can improve human labour in terms of quality and productivity beyond our wildest hopes. But passed a threshold, like the one in our example, the AI starts to become a competitor to humans.
People clearly cannot outproduce the AI. Nobody can write a year’s worth of high-quality content instantaneously. And even if the content is not always high quality, it will become good enough to require only a review, as we said.
So, if writers cannot write more, what value do they bring to the table to justify their salary? Will companies recognize that value and compensate for it as they do today?
After working the last 14 years in corporate environments, I have zero doubts that companies (which, never forget, are made of people) value quantity infinitely more than quality.
Attend more meetings.
Write more presentations.
Publish more papers.
Manage more direct reports.
And so what happens when “more” is a game humans cannot play anymore?
–
All of this leads us to the second thing that caught my attention this week: a comment by Aleksandr Tiulkanov, an AI and Data Policy lawyer.
The times where you could simply sit in an armchair and collect royalties are long over.
Your subscribers and customers need to clearly see what your unique value proposition is for them. And it better be so high as to make them never seriously compare your work to botwork.
— Aleksandr Tiulkanov (@shadbush) April 23, 2023
Aleksandr is referring to the news that streaming service providers are being urged to clamp down on AI-generated music, a topic we’ll explore in detail in a future issue of the Splendid Edition. However, his comment applies to practically everything we produce now that the competition with AI is looming.
To go back to the previous example, if a writer cannot demonstrate that his/her work is undeniably better than what an AI with a 2M token context window can do, then the writer has a ticking bomb with his/her name written on it.
And this, in turn, leads to at least two problems.
The first problem, I already mentioned: to go back to Aleksandr’s scenario, a lot of subscribers don’t care if the artist/creator/writer does better. They will only care if the artist/creator/writer can offer something of value that they cannot find anywhere else.
It’s not about how good this is. It’s about how useful this thing is to your audience right now.
Why is it a problem? Because figuring out if what you are doing is of value to your intended audience is one of the hardest things in the world. Ask the nine out of ten startups that go bust every year.
Many people don’t have an entrepreneurial mindset, the analytical mind to identify the most valuable thing they can offer, and the grit and perseverance to keep offering that thing until a big enough number of people notice it through all the noise.
The second problem: for many people, it’s incredibly stressful to operate in a work environment where you are constantly competing to keep your job.
How long can people sustain that stress without burning out?
What happens if they burn out?
What happens to the people that don’t want even try to sustain that stress?
–
The third thing that caught my attention this week: Worker Info Exchange published a 33-page report about the misuse of automated dismissal (or robo-firing as somebody calls it) by the company Just Eat.
Worker Info Exchange is a non-profit organisation dedicated to helping workers access and gain insight from data collected from them at work.
You give them the mandate, and they go collect the data on your behalf.
Like many other companies, Just Eat is exploring the use of AI and automation (two very different things that occasionally work together) to reduce the HR workload.
The AI identifies a fraudulent behaviour from a worker and, depending on the corporate policies, flags him/her for dismissal. At this point, an automation engine performs all the steps necessary to fire the worker. No human involved. All in the blink of an eye.
Obviously, if the AI model adopted by Just Eat or another company doesn’t do an exceptional job at identifying fraudulent behaviours, you end up with a bunch of so-called false positives, and a lot of people that lose their job without a chance to appeal.
From the report:
couriers are expected to update their status on the app five times after accepting an order: to confirm when they have arrived at the restaurant, when the food is collected, when they’re ready to deliver, when they have arrived at the delivery address, and when the delivery is complete. Any irregularities in the recording of these actions, whether the fault of couriers or not, can lead to immeate dismissal.
…
In the recent dismissals we investigated, couriers were initially sent a generic message notifying them of their contract terminations due to “unexplained instances in their accounts” where they had incorrectly updated their statuses, leading to financial gain from restaurant hold payments, in other words, waiting fees paid out for delays at restaurants. When the couriers pushed for further explanation, Just Eat stated:“These fraudulent activities are a serious breach of your courier agreement which we have to monitor and take actions against to protect our legitimate business interests. Seeing that you continued thesviolations after our
email and the company-wide announcement dated 24 September 2021, we decided to revoke your account’s network access permanently.”
…when we inquired about the reason for the dismissals, Just Eat confirmed that the couriers were marking themselves ‘parked at restaurant’ to indicate they were waiting, but then leaving the restauranwithout updating their delivery status as ‘order collected.’
Just Eat then offered the following explanation to couriers: “Our investigation team members manually observed and confirmed that during these deliveries, you left the restaurants for an extended perioof time after marking yourself as “Parked at Restaurant” to indicate you are waiting at the restaurant to pick up the order. Since you left these restaurants without updating your delivery status as order collected you received undeserved financial gains that are for compensating couriers who are held in their deliveries due to late functioning restaurants.”
Assuming that the system is working correctly, how much money did these horrible Just Eat drivers steal from the company? As much as £1.4
No, not One Million and Four Hundred Thousand British Pounds.
One Pound.
According to Just Eat, some of his drivers would risk their job to gain one pound.
So Worker Info Exchange investigated 11 cases after collecting the relevant GPS data and concluded:
There was no GPS data showing the courier’s movements during the waiting period either. The arrival and completion coordinates were presented as demonstrating that the courier had left the restaurant, however all we saw was the courier stepping outside of the restaurant, sometimes as little as three metres, or as briefly as eleven minutes.
In two of the cases, the order numbers identified in the request response email did not even match the order numbers referenced in the GPS data. Ultimately, we saw no data to verify that the couriers haleft the area of the restaurant in any of the data returns. The couriers were merely stepping several metres away so as not to crowd already busy restaurants, and sometimes being explicitly instructed to do so by restaurant staff.
Just Eat therefore did not provide any evidence that it was the couriers’ fault that the collection was delayed. In fact, this would not have been possible since there isn’t a function in the Just Eaapp that issues a notification for when the food is ready. Neither Just Eat, nor the couriers know when the order is ready for collection.
For all of the sophisticated surveillance technologies deployed across the platform, this has been left to the couriers to manually contend with. Couriers need to regularly go up to the counter to finout if the food is ready.
I won’t quote the rest of the report, which is incredibly interesting and worth a read. I’ll just say the following.
Earlier this week, MIT called for an interview about the Future of Life Institute (FLI) Open Letter that I signed last month together with a group of very high-profile AI researchers.
One of the questions I received was approximately this: “Don’t you think that future AI large language models will bring more benefits than downsides to people and, because of that, we shouldn’t pause their development?”
The answer I gave, which applies to the Just Eat situation as well, was approximately this: “I think that certain categories of people will benefit enormously from increasingly capable AI models while other categories will bear untold downsides because of them. And that’s because we have too little transparency in how these model work, and too little opportunity for the scientific community and the regulators to see how the models (and their data sets) comply with the regulations that exist, in the first place, to protect humans.”
Before closing, let’s hear from one of the drivers, courtesy of Heather Stewart, writing for The Guardian:
“I tried to explain to them: the restaurant is busy. I have so many orders from McDonald’s. I can’t park my car near the restaurant because the customers have only one McDonald’s in this city, it’s so busy. It’s a shopping centre there, and I should leave my car away and I should walk,” he said.
“They say: ‘Sorry, I can do nothing because this is the manager’s action.’ They don’t understand me. I tried to explain many times in email what happened. I tried to explain that I have a kid, this is my job.”
Of course, until algorithmic injustice reaches your line of work, it will be hard to see why AI transparency is a problem that should concern us all.
You won’t believe that people would fall for it, but they do. Boy, they do.
So this is a section dedicated to making me popular.
The US National Bureau Of Economic Research (NBER) published a very interesting new paper titled Generative AI at Work:
We study the staggered introduction of a generative AI-based conversational assistant using data from 5,179 customer support agents. Access to the tool increases productivity, as measured by issues resolved per hour, by 14 percent on average, with the greatest impact on novice and low skilled workers, and minimal impact on experienced and highly skilled workers. We provide suggestive evidence that the AI model disseminates the potentially tacit knowledge of more able workers and helps newer workers move down the experience curve. In addition, we show that AI assistance improves customer sentiment, reduces requests for managerial intervention, and improves employee retention.
These charts say a lot more:
The average handle time significantly decreases for a lot of agents and, as a consequence, the number of chats the customer service representatives can have in one hour increases.
But the most significant insight is this:
We see that all agents begin with around 2.0 resolutions per hour. Workers who are never treated slowly improve their productivity with experience, reaching approximately 2.5 resolutions 8 to 10 months later. In contrast, workers who begin with access to AI assistance rapidly increase their productivity to 2.5 resolutions only two months in. Furthermore, they continue to improve at a rapid rate until they are resolving more than 3 calls an hour after five months of tenure.
As Jo Constantz, writing for Bloomberg, puts it:
The most highly skilled workers saw little to no benefit from the introduction of AI into their work. These top performers were likely already giving the responses at the same caliber that the AI was recommending, so there was less room for improvement — if anything, the prompts may have been a distraction, the researchers said.
If AI does ultimately narrow the gap between low- and high-skilled workers, however, companies may need to fundamentally rethink the logic underpinning compensation choices.
…
Top customer service agents had Excel spreadsheets where they collected phrases that they used often and that worked well, MIT’s Raymond said. If the AI tool is indeed taking this tacit knowledge and distributing it to others, she said, “then these high-skilled workers are doing the additional service for the firm by providing these examples for the AI, but they’re not being compensated for it.” In fact, they may be worse off because their incentives were based on performance relative to their peers, which introduces a host of weighty policy questions about how workers should be compensated for the value of their data.
Notice two final things.
The first one: this is not a test in a controlled lab environment. From the paper:
We work with a company that provides AI-based customer service support software (hereafter, the “AI firm”) to study the deployment of their tool at one of their client firms, (hereafter, the “data firm”).
Our data firm is a Fortune 500 enterprise software company that specializes in business process software for small and medium-sized businesses in the United States. It employs a variety of chat-based technical support agents, both directly and through third-party firms. The majority of agents in our sample work from offices located in the Philippines, with a smaller group working in the United States and in other countries. Across locations, agents are engaged in a fairly uniform job: answering technical support questions from US-based small business owners.
The second thing to notice: it’s unlikely this is GPT-4 as access to their API is still quite limited. In fact, the overwhelming majority of research papers published these days are based on ChatGTP (aka GPT-3.5 Turbo).
Again, from the paper:
The AI system we study uses a generative model system that combines a recent version of GPT with additional ML algorithms specifically fine-tuned to focus on customer service interactions. The system is further trained on a large set of customer-agent conversations that have been labeled with a variety of outcomes and characteristics: whether the call was successfully resolved, how long it took to handle the call, and whether the agent in charge of the call is considered a “top” performer by the data firm. The AI firm then uses these data to look for conversational patterns that are most predictive of call resolution and handle time.
It’s not unreasonable to assume that it will be possible to increase the performance by well over 14% once GPT-4 starts to be adopted.
Soon enough, situations like this might start to happen in every industry, for every line of work. Most skilled workers might unknowingly fine-tune the AI that the company adopted, and, in turn, that AI will spoonfeed the newer and less experienced workers. So that everybody will be a rockstar and the employees that actually are high achievers, as we said here on Synthetic Work multiple times, will be recognized and compensated way less, if at all.
This is the material that will be greatly expanded in the Splendid Edition of the newsletter.
Unless you live under a rock, you heard of a song titled Heart on My Sleeve by the musician Drake.
And since you don’t live under a rock, you know that this song is not really from Drake, but from a mysterious creator (like Jesus, but with a mask and a cape, like Batman) called ghostwriter977, who cloned Drake’s voice and published the song on every video and music streaming platform on the Internet.
And, of course, it went viral.
Immediately after, also of course, music labels, who are dying to have the chance to sue to death generative AI companies and their users, took action and started to pressure streaming companies to not publish AI-generated songs.
Notice that there’s a difference between AI-generated music and human-generated music with a synthetic voice. But if we go there, then we need to ponder the true meaning of “human-generated music” given that, for decades, musicians have used synthesizers and samples stolen from older songs to create their hits. So let’s not go there, shall we?
If you cannot find Heart on My Sleeve anywhere when you read this newsletter, you have to thank Universal Music Group.
Joe Coscarelli for The New York Times:
in a statement this week, the company spoke to the broader stakes, asking “which side of history all stakeholders in the music ecosystem want to be on: the side of artists, fans and human creative expression, or on the side of deep fakes, fraud and denying artists their due compensation.”
There would be much to say about who gets the due compensation here, but it would be beside the point.
The point is that we already know how this story ends. We have seen it unfold in the last few months, as thousands of AI and digital art enthusiasts have fine-tuned the Stable Diffusion model after the art style of their favourite artists, inundating social media with artistic output that is not different from fan art.
(If you don’t know what I’m talking about, search for Greg Rutkowski.)
Even if companies like Stability AI are making it harder to generate artistic output too similar to the original material that the AI has been trained with, it’s getting trivial to fine-tune the AI yourself and achieve the same identical result.
In other words, you can’t generate an image in the style of Greg Rutkowsky out of the box with the latest Stable Diffusion model, but you can take a bunch of original images made by Greg Rutkowsky, upload them online on a service, for a few dollars, have a fine-tuned version of Stable Diffusion that generates Greg Rutkowsky images.
The same will happen with synthetic voices.
While companies like ElevenLabs or Resemble AI or Coqui will make it harder to clone the voice of an artist or an actor, if you are determined enough, you’ll be able to do it yourself with almost no technical expertise.
To prove this point, in March, I sneak-peaked Fake Show, a podcast with synthetic voices that I’m creating using a state-of-the-art generative AI model for voices:
So, here, if you are an artist there are only three choices:
The first choice is that you start suing every single person out there that generates a synthetic clone of your voice and uses it to create fan art.
Possibly, you leverage music label money and influence to lobby governments into passing laws that classify deepfakes creation as a criminal offence, like in China.
Well done.
The second choice is that you wait for technology to be so accurate and reliable that your every artistic output deemed original is watermarked. You also have to wait for every video and music platform and social media network out there to agree to upload only things that pass the digital verification.
The first problem is that watermarking technology is unreliable according to the tests conducted by OpenAI on their own watermarking techniques.
The second problem is that, even if watermarking was reliable, you still need to have an industry-wide agreement on enforcing it.
The third problem is that, once you start deciding what is original and what is not, you’ll have to justify all the samples created from old songs that you used to compose your original hits and you didn’t pay for.
The third choice is the smart choice:
I'll split 50% royalties on any successful AI generated song that uses my voice. Same deal as I would with any artist i collab with. Feel free to use my voice without penalty. I have no label and no legal bindings. pic.twitter.com/KIY60B5uqt
— 𝔊𝔯𝔦𝔪𝔢𝔰 (@Grimezsz) April 24, 2023
This is not the first time that artists allows others to openly use their voices.
At TED, Holly Herndon, an artist based in Berlin, thanks to AI, lent her voice to the musician Pher for a live performance.
Holly Herndon, created the AI framework Holly+ to allow others to create artistic output with her voice.
Any profit made out of a similar endeavour ends up in a Decentralized Autonomous Organization (DAO), a social structure supported by Blockchain technologies.
The members of this DAO decide what to do with the profits.
Back to Grimes.
Rather than fight against something that is clearly unstoppable, Grimes decided to embrace technology and use it to amplify her reach.
That’s the only smart thing to do because, sooner than you think, an AI that doesn’t clone anybody’s voice but it’s completely original will steal the top spot in worldwide music ranks and you will slowly start to slip into oblivion.
For any new technology to be successfully adopted in a work environment or by society, people must feel good about it (before, during, and after its use). No business rollout plan will ever be successful before taking this into account.
I talk to ChatGPT about things that I honestly can’t talk to my friends or family about.
And that’s not a comment on my friends or family. I’ve been through a lot and a person on the other side is just sometimes too much for me.
I have cried literal tears talking to ChatGPT.
— Jenny AI (@jenny____ai) April 16, 2023
If you are wondering if Jenny AI is a real human being, the answer is yes.
If you have read Synthetic Work long enough, by now you should see that people are reacting to AI with a whole spectrum of emotions and there’s not one way to feel about it. Equally true, different types of AI trigger different emotions, too.
I’ve said multiple times that generative AI has huge potential to become the ultimate tool to help the elderly and people affected by depression. So much life and happiness and productivity could be recovered thanks to large language models.
Do you remember Issue #1 – When I grow up, I want to be a Prompt Engineer and Librarian?
In that historical first issue, in a section called Don’t., I described my business idea for a terrible dating app.
Well, somebody has done worse than me. Much, much worse.
Let me introduce you to CupidBot:
Somebody has allegedly devised a mechanism to scrape data from Tinder, Bumble and various other dating apps, and let a large language model carry lead the conversation with a match on their behalf.
The conversation goes on until the pair agrees on a date. At that point, some automation mechanism creates a calendar event with the name of the match, phone number, and other relevant information that must be studied before the date.
A briefing on the date you never bothered to talk to.
The homepage says “We’re a team of machine learning engineers dedicated to enhancing the dating lives of men.” Apparently former Tinder engineers.
Every cell of my body wants to believe that this is a joke to warn people about the dangers of pushing AI and automation too far. But there’s a non-insignificant group of those cells that harbours the doubt that Cupidbot is a real thing.
It doesn’t really matter.
Even if it’s not real, eventually, somebody will create a real version of this.
Also, it’s impossible to not think about the episode Hang the DJ from the acclaimed documentary series Black Mirror:
Last week, I mentioned a new perk for those members of Synthetic Work with a Sage, Innovator, and Amplifier subscription: the Best AI-Centric Tools for X (or Best For) database.
That tool is not finished yet because, first, I had to create another tool: the AI Adoption Tracker.
This is something I wanted to do for 20 years, and not just about AI but about any emerging technology I focused on during my career: a tool that tracks what organizations in what industries are using what type of AI (from what technology provider) for what use case.
Tracking the technology providers that offer a solution in a given market segment is easy. The biggest challenge is keeping up with the number during the market expansion phase, and figuring out a taxonomy that is comprehensive, easy to understand, and not prone to obsolescence after two minutes…