August 12, 2023
Free Edition
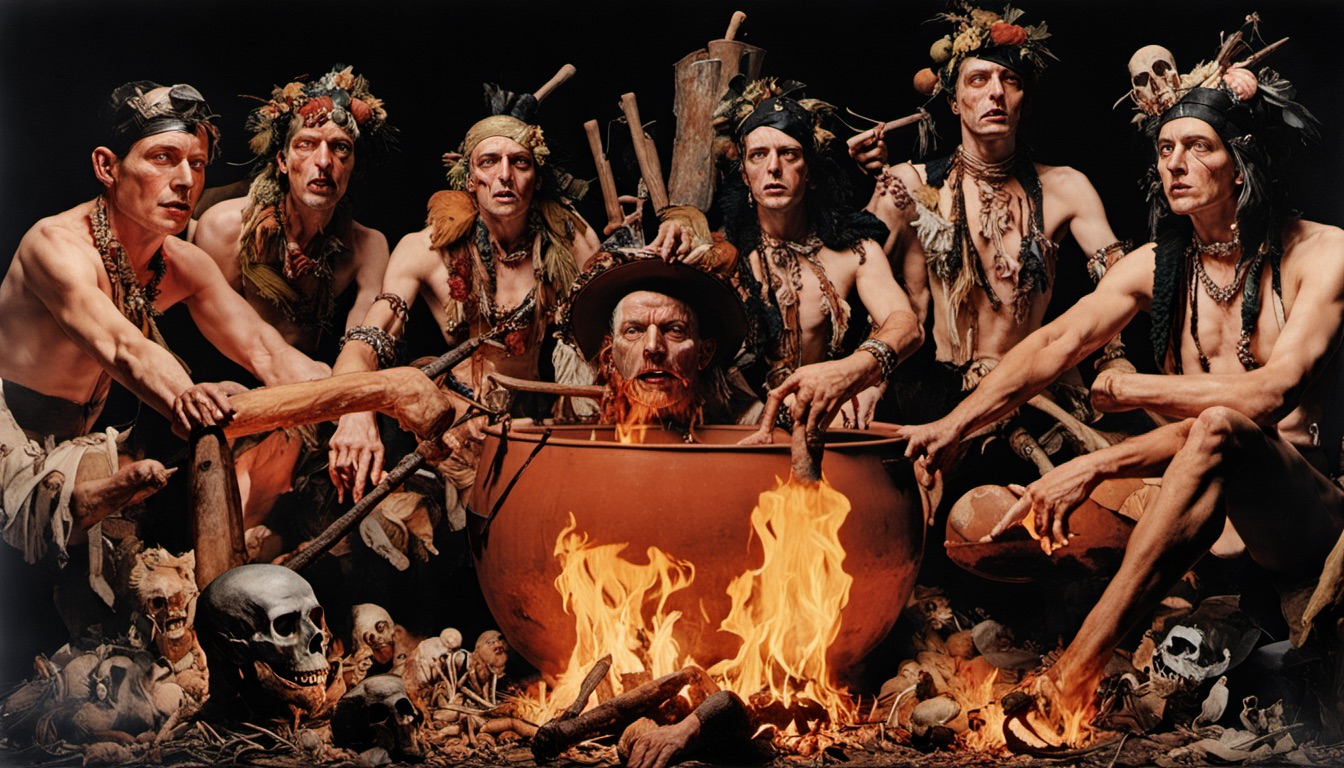
In This Issue
- A rare interview with the Anthropic CEO Dario Amodei and his view on the integration of AI in business environments.
- Billionaire investor Chamath Palihapitiya on the impact of AI on software development and the implications for public companies pressured by activist investors.
- A leaked conversation during an Adobe staff meeting about the risk of cannibalizing the company’s own business model with AI.
- The New England Journal Of Medicine published a very interesting article on the adoption of AI in the Health Care industry.
- The collaboration between a rapper, Lupe Fiasco, and Google is a great example of collaboration between humans and AI. For now.
- A new report from the UK House of Commons Committee touches on how workers might feel in a workplace where they are surveilled and judged by tech and AI.
P.s.: this week’s Splendid Edition of Synthetic Work is out, titled The Ideas Vending Machine.
In it, you’ll read what WPP, the London Stock Exchange Group, Tinder, and Australia’s Home Affairs Department are doing with AI.
Also, in the What Can AI Do for Me? section, you’ll read how GPT-4 can be used to generate perfectly legit business ideas. For real.
You need a paid membership to read this content.