November 4, 2023
Free Edition
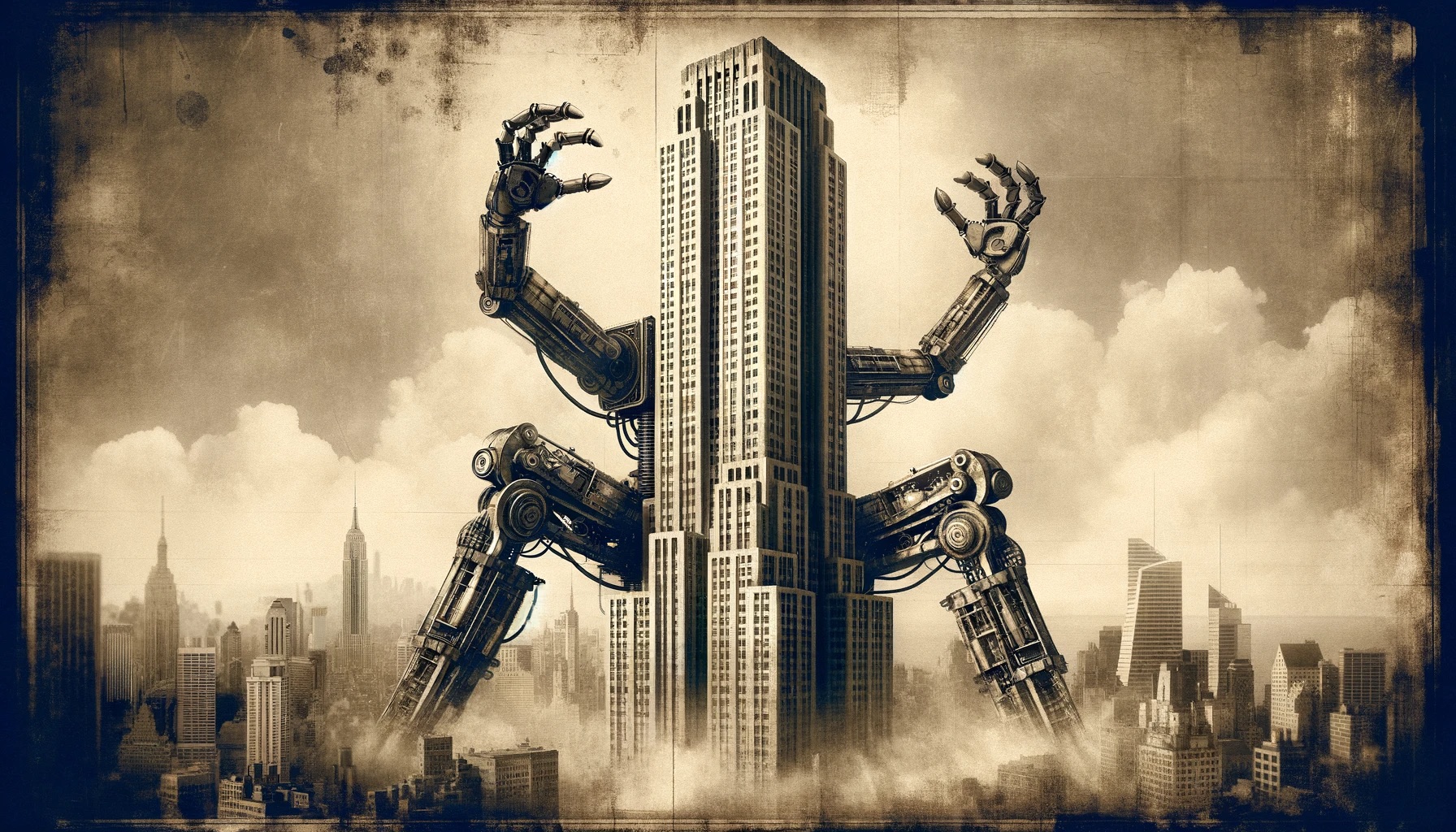
In This Issue
- Intro
- Synthetic Work reaches an important milestone.
- What Caught My Attention This Week
- Creating synthetic advisors vs synthetic analysts, and the legality of AI-led corporations.
- Goldman Sachs lifts US GDP forecast to account for the impact of generative AI.
- Can AI threaten the job of the field researcher? Can it at least decode animal language?
You need a paid membership to read this content.