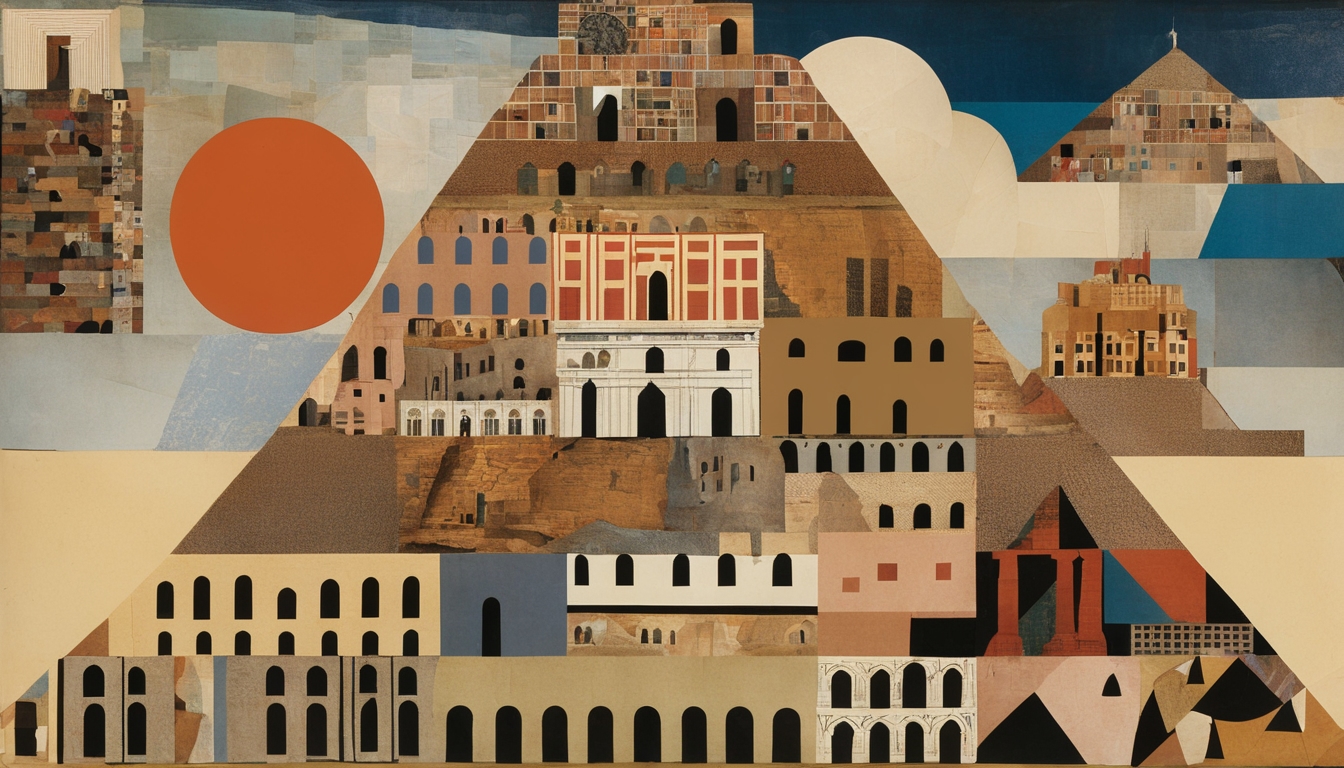
- Intro
- Should you bother learning how to use generative AI? What if it could change your career?
- What Caught My Attention This Week
- We might have real-time transcription of spoken audio before the end of 2024.
- AI algorithm was far better than a biopsy at correctly grading the aggressiveness of sarcomas.
- ServiceNow CEO suggests that AI will not displace software engineering jobs as the demand outstrips the supply.
- The Way We Work Now
- 39,000 volunteers on social media have started using face recognition AI to give a name to unidentified dead bodies.
Earlier this week, I released my AP Workflow 6.0 for ComfyUI.
Some context for the new readers of Synthetic Work: ComfyUI is a node system that allows you to automate the creation and manipulation of images, videos and, most recently, text, using generative AI models.
It started with a focus on image generation via the open access model Stable Diffusion, but now the community is greatly expanding the scope of the system. I’ve been personally pushing for the creation of new nodes supporting large language models (LLMs) like GPT-4, and I’m happy to see the community going in that direction.
As you can infer from the image below, ComfyUI is a very complicated system, but no different than other node systems professionals across industries use for 3D rendering, VFX, color management, music production, etc.
The AP Workflow is a large, moderately-complicated workflow I created, mantain, and distribute freely to the community, designed to generate or manipulate digital output at industrial scale. Its advanced pipeline can power both enterprise-focused and consumer-focused applications.
Why am I bothering with such a complicated technical tool?
Because there is no other free access, ready-to-use scaffolding to do things like:
- Attempting to build a commercial competitor to Midjourney
- Powering groundbreaking enterprise applications for fashion houses, animation studios, ad agencies, etc.
- Researching disinformation at scale (for example, to disrupt elections)
Now that this scaffolding has reached a certain level of maturity, I can fully focus on these use cases and their business economics with first-hand experience.
Of course, not everyone sees the potential of the AP Workflow and the opportunity it unlocks. So, every time I share the new version on Reddit, I get comments from people who don’t see the point of what I’m doing.
The one I got this week:
With all the respect that this hard work generates, I can only say that this is not the future. It is, aside from the differences, the same feeling that the prompts gave me, becoming more and more complex every week. That’s not the future, no matter how you look at it, sooner than later this will be obsolete.
This comment gave me the opportunity to talk about the choice that all of us need to make when facing generative AI.
Even if it seems otherwise, my reply is not about ComfyUI, Stable Diffusion, or even automation:
Divergence of opinions is welcome. But it would be better to contextualize your prediction: a node system for AI generation is not the future…for what use case? And in how much time?
– For what use case?
Re prompts: there is little doubt that prompt engineering will eventually go away for end users. In part, because these models will understand better what we want when we ask to generate X, in part because much of the prompt engineering effort will be moved to the backend, which is exactly what I’m showing in AP Workflow 6.0.The point of the Prompt Enricher function is to offload the end user from the burden of figuring out how to write an uber-complicated prompt. The integrated LLM will do the job. And this is not different from what Midjourney does behind the scenes.
If you spent some time reading the FAQ on my website, you know that my motivation for building this workflow, and my long-term goal, is to create an advanced, multi-modal automation pipeline that can enrich the user prompt and the generated output in a way akin to what commercial systems do.
The workflow cannot do anything to improve the quality of a model, but it can create an advanced scaffolding that automatically improves anything the model spits out.
Of course, the more the user is offloaded from the task of writing the prompt, the less amount of control it has over the final output look and feel. Eventually, we’ll fix this, too, with natural language-driven editing of existing images, but we are still far from a viable solution.
If your use case is “I want to just generate a good picture of subject X,” then you are absolutely right: prompt engineering will not be a concern of yours in the future.
But if your use case, for example, is “I want to compose a very complex picture in layers and regions, where every region and every layer is created in a different artistic style, and I want to capture that process in a way that is repeatable for my future artworks, as it will become my own unique artistic style,” then you need extreme control that might be achievable only with prompt engineering, even in the future.
Re node systems: if you look across industries, node systems are everywhere because they provide the most precise way to control a digital creation/transformation process, at industrial scale. We use them for 3D rendering, VFX, color management, music production, etc.
If a fashion house wants to test how a new garment from their new Summer Collection 24/25 looks on 500 models available in the roaster of their model agency, and then repeat the process for all the new clothes and accessories in the collection, A1111/SD Next/Stable Studio can’t help with that. They either take thousands and thousands of pictures, or they resort to an automation pipeline like the one the ComfyUI and AP Workflow offer.
Of course, if your use case is “I’m an individual artist and I have to generate max 10 images that don’t require any particular acrobatic.”, then ComfyUI is not the future. But I’d argue that it’s not even the present. It’s absolutely not worth the investment necessary to learn and maintain the system.
Could node systems be replaced by an LLM? Or at least, could an LLM generate a node diagram on behalf of the user so we don’t have to waste any time building the pipeline?
Probably. But then you go back to the problem with the prompts: more abstraction, less control. And if you spend too much time editing the digital output produced by an LLM, you might end up deciding that it’s not worth the effort.
– In how much time?
Let’s say that, despite everything I said above, both the need for prompt engineering and the need for node systems will go away in the future. It depends on when.If the prediction is that the need will go away in 10 years, then I’d argue that it would be a shame to waste 10 years of productive life by not learning a tool that could really make a difference in your profession and, potentially, elevate your profile to international fame.
If, instead, your prediction is that the need will go away next year, then you are right: we are all wasting time here and we would be better off by just waiting a little bit longer.
So, what’s your prediction? 1 year or 10? And how can you be sure? And what if you are wrong?
Each person interacting with generative AI out there has to ask these questions, come up with their own answers, and evaluate the consequences of being wrong. But it’s a conversation worth having.
What are you betting your career on?
Alessandro
Earlier this week, Vaibhav Srivastav released hard-to-believe numbers about the performance of the new OpenAI model Whisper 3.
For the ones that don’t know what it is: Whisper is an open AI model that can transcribe spoken audio in multiple languages. It’s remarkably accurate compared to previous approaches, and it’s now being used by a wide array of commercial applications, including Descript, the audio/video editing solution we discussed many times in the Splendid Edition of Synthetic Work.
Thanks to a new optimization, the new Whisper 3 model can transcribe 1h and 30min of spoken audio in 1min and 38sec.
Why do these numbers matter for Synthetic Work readers?
OpenAI released Whisper 2 in December 2022. Whisper 3 was released in November 2023.
In just eleven months, the transcription time fell dramatically, and if we continue at this pace, we might have real-time transcription of spoken audio before the end of next year.
This means that we are very close to real-time live streaming in hundreds of languages at the same time. And to a real-time universal language translator.
And while that is wonderful, that would have a massive impact on all the people that offer transcription and translation services today.
Now, to set the expectations right: even if we achieve real-time transcription of the English language next year, there’s still some work to do to reach parity in other languages. The performance of Whisper 3 is not uniform across languages.
Plus, occasionally, Whisper can make up words. Yes, even speech-to-text models can hallucinate.
So, it’s not that the industry of transcription and translation services will disappear overnight, but it’s clear that the workload for those professionals will be dramatically reduced very soon.
On this point, a keen reader of Synthetic Work asked me over LinkedIn about the accuracy of Whisper.
Is Whisper 100% accurate? No.
In my experience, Whisper v2 is remarkably accurate (I use it every week for my video podcast about AI), but still needs a human editor to polish the output before publishing.
Whisper v3 is even more accurate: OpenAI reports that Whisper v3 shows a 10% to 20% reduction of error compared to Whisper v2.
But the (rhetorical) real question is: is any human transcriber 100% accurate at the speed reported above?
Which, in turn, leads to another (rhetorical) question: will Whisper be more accurate than a human transcriber in a real-time transcription?
No human can transcribe in real-time. My mother used to be a stenographer, and she taught me that the best humans can do is transcribe in shorthand, and then expand the shorthand into full sentences later.
If your use case doesn’t need 100%, then Whisper v3 is already more efficient and cheaper than a human transcriber.
And if you think that there are not many use cases that require 100% accuracy, I invite you to watch any video published by Bloomberg. Those videos feature interviews with world-class CEOs and other world leaders, saying things that can have a material impact on the economy.
Yet, if you turn on their auto-generated captions, you’ll immediately see how poor the technology they are using is.
Now.
Before we move on, let’s go back to the mythical scenario of real-time live streaming in hundreds of languages at the same time for another important caveat.
As I said multiple times in this newsletter, you should expect that, in a not-too-distant future, YouTube will flip a switch allowing any video ever published to be available in any language.
However, the quality of the transcription is only half the problem. The other half, which I extensively discussed in the Splendid Edition of Synthetic Work, is the lip-syncing of the translated audio.
We’ve seen incredible progress made in the last twelve months by companies like ElevenLabs and HeyGen, but as you saw in Issue #29 – The AI That Whispered to Governments, we are far, far away from a level of quality that is acceptable for professional use.
So, when we say that “we are very close to real-time live streaming in hundreds of languages at the same time. And to a real-time universal language translator,” we are talking about the audio portion of this use case.
As researchers expand their exploration of AI applied to the medical field, more promising results are coming out. A new study suggests that AI is almost twice as accurate as a biopsy at understanding how quickly cancer is progressing.
Andrew Gregory, reporting for The Guardian:
A study by the Royal Marsden NHS foundation trust and the Institute of Cancer Research (ICR) found that an AI algorithm was far better than a biopsy at correctly grading the aggressiveness of sarcomas, a rare form of cancer that develops in the body’s connective tissues, such as fat, muscle and nerves.
…
The team specifically looked at retroperitoneal sarcoma, which develops at the back of the abdomen and is difficult to diagnose and treat due to its location.They used CT scans from 170 Royal Marsden patients with the two most common forms of retroperitoneal sarcoma – leiomyosarcoma and liposarcoma. Using data from the scans, they created an AI algorithm that was then tested on 89 patients in Europe and the US.
The technology accurately graded how aggressive the tumour was likely to be 82% of the time, while biopsies were accurate in 44% of cases. AI could also differentiate between leiomyosarcoma and liposarcoma in 84% of sarcomas tested, while radiologists were unable to tell the difference in 35% of cases.
…
“As patients with retroperitoneal sarcoma are routinely scanned with CT, we hope this tool will eventually be used globally, ensuring that not just specialist centres – who see sarcoma patients every day – can reliably identify and grade the disease.”Messiou added: “In the future, this approach may help characterise other types of cancer, not just retroperitoneal sarcoma. Our novel approach used features specific to this disease, but by refining the algorithm, this technology could one day improve the outcomes of thousands of patients each year.”
This is the point where I would go back to the archives of Synthetic Work to provide all of you a link or two to previous issues where we discussed the use of AI in cancer screening and or treatment.
That task can take anything between 10 minutes and 1 hour, depending on how far back I have to go to retrieve the information I vaguely remember publishing.
Instead, today, I’m using the new Synthetic Work search engine powered by OpenAI custom GPT that I created last week:
Every time I use this new tool, I smile.
But it is accurate? In describing how I created it, in Issue #37 – What is a search engine, really?, I warned you that, just like any other large language model, this incarnation of GPT-4 can hallucinate. So we need to double-check.
The answer is: mostly, but not completely.
The GPT is accurate in retrieving the information you see in the screenshot above, but not 100% accurate in associating that information with the correct issue of Synthetic Work. One out of two linked Issues was a made-up URL.
The problem is related to a temporary limitation of these custom GPTs, as I described in the tutorial. It will go away in the future, but for now, we need to live with an imperfect search engine.
Old school linking to past issues of Synthetic Work where you’ll find more information about the effectiveness of AI used to screen other types of cancer:
- Issue #25 – The Clean-Up Commando
- Issue #26 – I have a bridge to sell you
- Issue #4 – Medical AI to open new hospital in the metaverse to assist (human) ex doctors affected by severe depression
ServiceNow CEO Bill McDermott suggests that AI will not displace software engineering jobs because the demand outstrips the supply.
David Rubenstein interviews him for Bloomberg:
Q: Is AI going to reduce the amount of jobs that people have now?
A: I don’t believe so. I think if you look at the market today, we have a 17-year situation. It was 17 years ago when the job market was this tight, when the openings outpaced the opportunity for candidates to jump in those jobs because the candidates aren’t there. So we really are capitalizing on technology to fill that void.
For example, I talked to a CEO just yesterday of a major financial services company. He was interested in AI. But he said: “I just can’t get enough people to do what I need to have done.”
I said: “Well, how many engineers do you have?”
He said: “I have 7000.”
“What you need to do is AI-enable them because now you can text-to-code, text-to-workflow-automation or even text-to-new-application-development. So we can make your engineers 50% more productive than they are today.”
The demand for AI researchers and ML engineers has exploded. That is true.
At the same time, we always need to have a broader look at the job market and put the demand for AI experts in perspective. And, we have to look at the long-term implications of what’s happening today, not just the short-term signals.
Put these two things together and you might remember an analogy I’ve been using for a while:
While we wait for the utopia of a world where nobody has to walk thanks to cars, everybody might be asked to become a car mechanic. At least, as long as the cars don’t start fixing themselves.
Techno-optimists make three assumptions:
- Cars will not be able to fix themselves until we reach the utopia.
- Until then, there will always be a need for car mechanics.
- People will be happy in a world where there is hardly any other job than the car mechanic.
It’s possible, but I don’t think we have the data to make that assumption.
An alternative possibility, as I said many times, is that things might get much better before they get dramatically worse.
For more about the latter scenario, you might want to read the intro of Issue #15 – Well, worst case, I’ll take a job as cowboy
This is the material that will be greatly expanded in the Splendid Edition of the newsletter.
Believe it or not, 39,000 volunteers on social media have started using face recognition AI to identify dead bodies to help families find their missing loved ones.
Deidre Olsen, reporting for Wired:
There are an average of 4,400 unidentified new cadavers per year in the US, and a total of 600,000 missing people across the country. Some of these cases are collected on databases, such as the National Missing and Unidentified Persons System (NamUs), which helps medical examiners, coroners, law enforcement officers, and members of the public solve missing, unidentified, and unclaimed cases across the country. The true scale of the problem is unknown, as the data available for the average number of unidentified cadavers comes from a 2004 census. Just 10 states have laws requiring that cases be entered into NamUs, meaning that many reports are voluntary.
…
Lee noticed a pattern in which cases were solved and which weren’t. The decisive factor was often money. Funding from private donors, sponsorship, and public support meant that law enforcement agencies were able to access cutting-edge technology, such as Othram, a forensic genetics company, which has been pivotal in cracking several high-profile cases. Those that weren’t solved didn’t have resources behind them.
…
Lee set up a TikTok to try to raise awareness. After a few false starts, she went viral, attracting a following of 128,000. She set up a Facebook group—Thee Unidentified & Unsolved—which now has 39,000 members, many of whom work together to solve unidentified and unsolved cases. Thee Unidentified & Unsolved is one of several volunteer social media communities that are filling a gap left by the US state, a gap that is getting worse due to the overlapping crises of poverty, fentanyl, and shortfalls in public funding. Now, with AI image recognition more readily available, volunteers have new tools to help them identify the deceased. This brings with it new issues around privacy and consent, but those in the communities say their work brings closure to families.
…
There are around a dozen posts each day in the group, often unsolved cases from NamUs with pictures. Members scour the internet, looking for other images, comparing images with missing person sketches or social media profiles.Some of the identification groups work globally; others are region- or country-specific or dedicated to unique circumstances, such as missing and murdered Indigenous women and girls. Online detective groups often tread a delicate line between altruistic investigation and mob obsession.
…
One member of Thee Unidentified has recently began using a new tool, PimEyes, a controversial facial recognition search engine, as a means to identify the deceased via morgue photos. A quick upload produces search results in a matter of seconds. Photos from across the internet are organized in a single view, mugshots frequently among them. While this technology can accelerate the process of identifying the dead, it brings with it serious privacy concerns. In many cases, informed consent is obtained for neither the image uploaded nor the results that the technology returns, which can include the biometric data of private individuals. Thus far, a few members of the group have utilized this tool.
…
PimEyes has been criticized by privacy advocates for scraping the internet for images and giving users access to highly personal information about private individuals. PimEyes CEO Giorgi Gobronidze says that these threats are exaggerated, and that PimEyes doesn’t hold images but just directs users to the URLs where images are hosted. “The tool is designed to help people to find the sources that publish photos, and if they shouldn’t be there, apply to the website and initiate takedown.” Gobronidze says that PimEyes has many use cases, such as searching for missing people, including women and children in conflict areas, and actively cooperates with human rights organizations.
…
In other social media groups, PimEyes is slowly being introduced as an investigative tool for cases related to missing persons, cold cases, and human trafficking.
The article is fascinating and worth reading in full. But remember: facial recognition is not 100% accurate and the algorithms that PimEyes uses are not routinely vetted by a panel of experts for accuracy.
Of course, the counter-argument to this is that inaccurate facial recognition algorithms have been used by police forces all around the world for years. And, in this particular use case, the volunteers probably feel that something is better than nothing.
If this approach yields tangible results, eventually, it might be formally adopted by coroners and law enforcement agencies, enabling one of the most surreal use cases of applied AI we’ve seen so far.
This week’s Splendid Edition is titled How to do absolutely nothing to prepare the best presentation of your career.
In it:
- Intro
- We are just getting started.
- What’s AI Doing for Companies Like Mine?
- Learn what the Australian government, GE Appliances, and Warner Music Group are doing with AI.
- What Can AI Do for Me?
- Meet the new Synthetic Work’s Presentation Assistant.