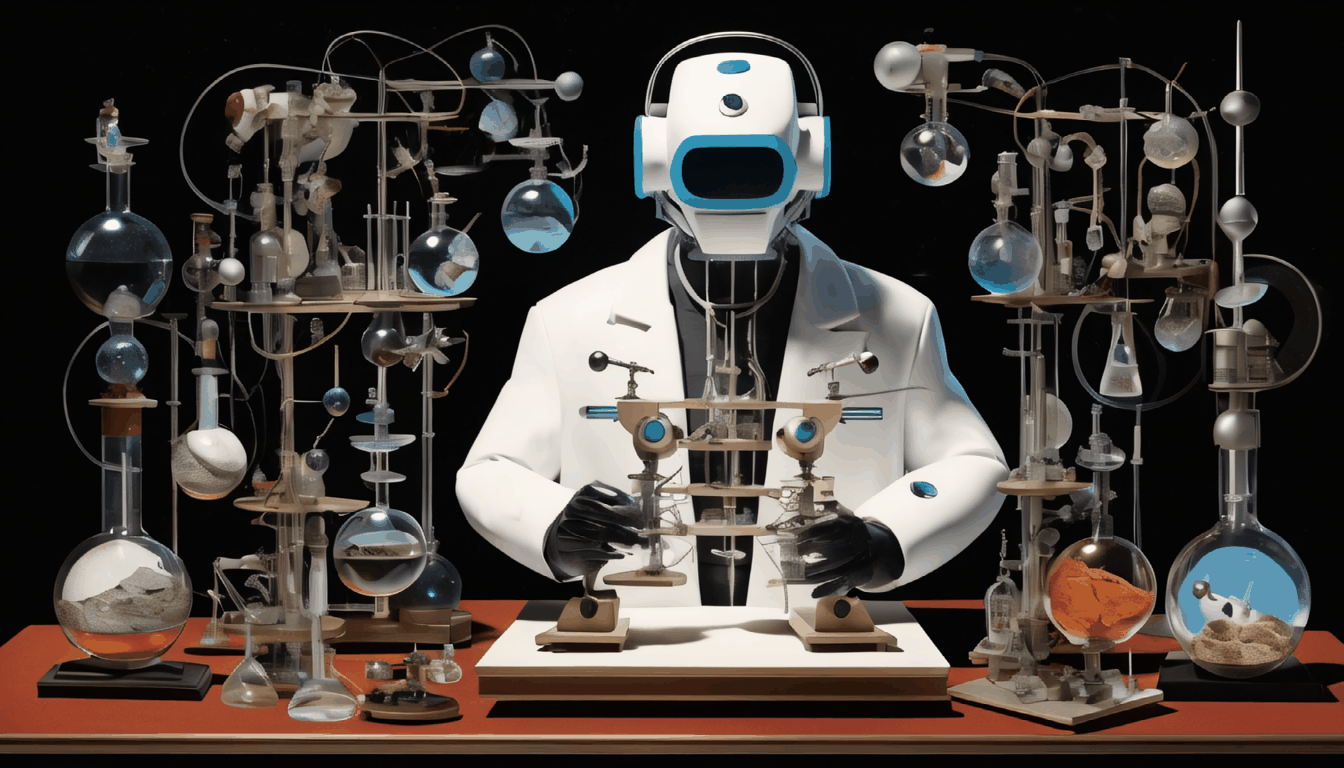
- Intro
- The cultural bias of large language models, all of a sudden, matters.
- What Caught My Attention This Week
- OpenAI is preparing to offer a self-service version of ChatGPT Enterprise, but COO Brad Lightcap doesn’t think AI can transform businesses overnight.
- DARPA is looking to train AI models to behave as synthetic scientists.
- JP Morgan says AI tools are starting to generate revenue for the bank.
- Goldman Sachs is losing the most AI talents to other financial institutions. Is it an attitude problem?
- The UK Department for Education’s Unit for Future Skills puts London finance and insurance professionals on high alert for the expected impact of AI on the job market.
Before we get started, a quick public service announcement.
Next week, I’ll travel to keynote a private event, and to support a hackathon about generative AI for all the employees of a forward-looking consulting company in Italy.
Hence, Synthetic Work takes a break. It will be back for the last Free and Splendid editions of the year on December 29.
If AI was a normal field, this break would also align well with the typical lack of progress during the holidays. But AI is not a normal field. It’s entirely possible that, while the world celebrates things like Christmas and New Year’s Eve, some researchers announce a breakthrough that will change the world forever.
Because. Those. People. Never. Sleep.
If that happens, I’ll send out a This Couldn’t Wait, Could it? Edition.
If it doesn’t happen, and you still want to read something about AI, this is a great time to catch up on the past editions of Synthetic Work, now neatly displayed in a new Archive page.
Also, remember that Synthetic Work offers a Breaking News section on our website, and that will continue to be updated during the holidays.
OK. Now that we got that out of the way, let’s get to what matters.
Long-time readers of this newsletter know that, on multiple occasions, I’ve highlighted how large language models can carry a bias that subtly influences the way they generate text and, therefore, the users that depend on it
These biases can be cultural, ideological, political, racial, etc. And while the racial bias is the most evident and the hardest to tolerate in our age, I’ve expressed much more concern about the others on the list.
These models end up powering consumer-facing AI systems like ChatGPT or Claude, and enterprise-grade alternatives, privately consumed by millions of employees working across all industries.
If they are economically biased, they can manipulate our purchases, or what we think is of high value in our society. This is the most common risk we face with intentionally biased models that promote certain products or services over others in their answers to the users.
If these models are politically biased, they can manipulate the user perception about certain values that are associated with left or right parties and, potentially, influence elections.
If they are ideologically biased, they can manipulate the user perception of what’s right and wrong, potentially influencing the acceptance rate of certain corporate or government behaviors among the workforce or the general population.
Of course, none of this theoretical manipulation happens overnight. It’s a slow process that can take weeks, months, or even years to show its effects. You are probably familiar with the hypothesis that the CIA used modern art as a vehicle to promote American values and slowly influence his opponents during the Cold War.
True or not, that is an example of long-term ideologic manipulation.
Moreover, this theoretical manipulation is predicated on the fact that the users interact so much with these AI systems, anthropomorphizing them, being swayed by their synthetic voices, or their uncanny artificial empathy, that they end up trusting them more than they trust their own judgment.
It’s not unthinkable that an executive in a large company, becoming dependent on one of these AI systems, will eventually see his/her business decision-making influenced by the AI’s bias. And business decision-making can be anything, from the choice of a new supplier to the decision to enter a particular market.
So, the bias of these models matters when it comes to the focus of this newsletter: the way we work.
Of course, for people to trust these models to the point of letting them influence their decision-making, they need to be exceptionally accurate and reliable, and they must be used all the time. These models must become dependable and indispensable.
Almost one year ago, the seasoned CEO of a startup I know told me: “I use ChatGPT every day. For everything. It’s amazing.”
Like him, throughout 2023, Synthetic Work documented how a growing number of people are developing emotional relationships with these AI systems, and how their adoption in the workplace is accelerating.
Today, ChatGPT has about 100 million weekly active users, and more than 92% of Fortune 500 companies are using it.
Its website had 14 billion visits between September 2022 and August 2023.
Plus, ChatGPT is becoming more amazing. And so are open access models that compete with it.
We now have an increasing number of those models that are on par with OpenAI GPT-3.5-Turbo, and we are starting to see the first few models that beat GPT-4 in selected use cases.
But that’s not the only evolution to pay attention to.
If you consult the leaderboard of best performing open access models out there, diligently updated by Hugging Face, you’ll discover that in the top 10, for the first time ever, there are some models trained by Chinese researchers: Qwen-72B and Yi-34B-200K.
You’ll also discover that these models have already been mixed with Western-trained models to create benchmark-beating hybrids. For example: platypus-yi-34b or Yi-34B-Llama.
There is a growing number of them:
Open Access LLMs trained in China: code, LLMs, math, audio, and more. Let's dive into 8 of them:
Qwen
– 1.8B/72B parameters family of models
– Base, Chat and Quantized
– 32k window size (the large one)Yi
– 34B base and chat versions
– Up to 200k context length
– 4 and 8-bit…— Omar Sanseviero (@osanseviero) November 30, 2023
When people see the exceptional performance of these new models, the immediate reaction is the most predictable one: “There you go. China is finally catching up with the West, and soon it will surpass it.”
All I see, instead, is a series of powerful AI models that are as biased as the ones trained in Western countries, but in a different way.
If these models end up showing exceptional performance, not using them will mean losing a competitive edge for a startup or a large organization. Using them, instead, might mean gaining an enormous user base. It’s an easy choice for any commercial entity on the market.
For example, what happens if these models end up powering some parts of TikTok? Or the next Western-based social network that will replace TikTok and has no issues using them instead of the exceptionally expensive alternatives offered by OpenAI and Anthropic?
What happens if these models end up being offered for free even for commercial use?
China, India, and every other country in the world has to train its own AI models not just to not be economically dependent on the West, but also to avoid being exposed to Western biases daily and, potentially, being manipulated by them.
If these models can influence the way people think and conduct business, as I believe, there is a lot at stake.
OK.
Putting intentional adversarial behavior, can’t the solution simply be to remove the bias from AI models?
I’m afraid not.
These models are trained on an immense pile of inherently biased content: the digital output of human beings. To remove the bias from the training dataset, we have to remove the bias from the humans.
We want large language models, or future AI technologies, to be flawless in bias, judgment, and morals. But isn’t that a characteristic of “God” in multiple religions?
Are we asking scientists to give us AI or a digital version of our God?
Happy Holidays
Alessandro
OpenAI is preparing to offer a self-service version of ChatGPT Enterprise, but COO Brad Lightcap doesn’t think AI can transform businesses overnight.
Hayden Field, interviewing him for CNBC:
Q: In August, 80% of Fortune 500 companies had adopted ChatGPT. Now, as of November, you’re at 92%. As far as that remaining 8% of companies that haven’t adopted the tool yet, have you noticed any trends?
A: My guess is it’s probably heavy industry in some senses. … Big, capital-intensive industries like oil and gas, or industries with a lot of heavy machinery, where the work is more about production of a good and a little bit less about being an information business or a services business.
…
Q: In your eyes, what’s the most overhyped and underhyped aspect – specifically – of AI today?A: I think the overhyped aspect is that it, in one fell swoop, can deliver substantive business change. We talk to a lot of companies that come in and they want to kind of hang on us the thing that they’ve wanted to do for a long time – “We want to get revenue growth back to 15% year over year,” or “We want to cut X million dollars of cost out of this cost line.” And there’s almost never a silver bullet answer there – there’s never one thing you can do with AI that solves that problem in full. And I think that’s just a testament to the world being really big and messy, and that these systems are still evolving, they’re still really in their infancy.
The thing that we do see, and I think where they are underhyped, is the level of individual empowerment and enablement that these systems create for their end users. That story is not told, and the things that we hear from our users or customers are about people who now have superpowers because of what the tools allow them to do, that those people couldn’t previously do.
…
Q: We’re now a couple of months into ChatGPT Enterprise. I remember you launched after less than a year of development, with more than 20 beta tester companies like Block and Canva. How, specifically, has usage grown? Who are some of your biggest clients since launch, and how much of a revenue driver is it for OpenAI?A: The enthusiasm has been overwhelming. We’re still a smallish team, so we don’t offer the product self-serve as of today – we will imminently – but we’ve tried to get through as many interested parties as we can get through. …
A lot of the focus of the last two months was really making sure that those first few customers that we implemented and onboarded saw value in the product. … We’re still working through waitlists of many, many, many thousands, and our hope is to get to everyone, and that’s going to be a goal for 2024.
The most consistent thing that comes across from all the interviews OpenAI executives have given so far is that they firmly all believe that this is the early stage of a long journey.
Most people, instead, think about ChatGPT and DAll-E as the peak of how good AI can be.
Your projection of peak performance dramatically influences your business strategy. And your personal commitment.
DARPA is looking to train AI models to behave as synthetic scientist.
Long-time readers of Synthetic Work know that one of my most important research projects I’ve been working on in the last few months is what I call AI Council or board of synthetic advisors.
I showed you how we can already use the technologies we have today to create a synthetic board in Issue #31 – The AI Council.
So I was thrilled when eagle-eyed Synthetic Work reader Matthew sent me a new proposal from the US Defense Advanced Research Projects Agency (DARPA) called Foundation Models for Scientific Discovery (FoundSci).
From the official proposal document:
Goal: The FoundSci AIE aims to explore, develop, and demonstrate an “autonomous scientist” (i.e., an AI agent) capable of skeptical learning and reasoning to act as an aid to human scientists.
This AI must use scientific reasoning to generate creative hypotheses and experiments, and refine said hypotheses to enable scientific discovery at speed and scale.
Background: Current methods in AI for scientific discovery are ad-hoc, with DeepMind leading the charge (e.g., AlphaFold). Separate models need to be developed for different scientific experiments and fine-tuned for different prediction tasks. Furthermore, these methods are still limited to uni-modal data, low-dimensional experiments, and lack physical or scientific reasoning. For example, DeepMind’s AlphaFold is hand-crafted based on the knowledge of multi-disciplinary scientists who encode known inductive biases (e.g., Van der Waals forces, valid protein folding angles, electrochemical assertions) into the machine learning (ML) models.
We argue that such inductive biases, which greatly reduce the search space of hypotheses and experiments, should be identified, or derived automatically, by the AI. If we follow the conventional approaches to foundation models (e.g., large language models (LLMs) and other large pre-trained models, we may not succeed in developing autonomous scientists that can generate novel hypotheses because such novelty would not have been encountered in the data used to train the model. Indeed, using LLMs as a comparison, generating novel experiments requires creativity, whereas generating novel text (e.g., ChatGPT) requires semantic and syntactic conformance, so the latter is well-suited for current methods of next-token prediction using large pre-trained models. How such models can be created to be creative is an open research question that we seek to answer in this AIE.
…
The FoundSci effort seeks research in the area of building new foundation models for scientific discovery that can be the basis of an autonomous scientist. This effort envisions an autonomous scientist possessing the ability to characterize its own uncertainty and skepticism, and use them as drivers to systematically acquire and refine its scientific knowledge bases, in a way that
human scientist partners can trust. The resulting capability would augment DoD research (such as in DoD service labs) and accelerate discovery in any discipline (e.g., the discovery of new materials or the discovery of new computing architectures to robot designs), especially in an era where the volume of experimental data and research papers are increasing exponentially.Proposals should aim to develop the ideas of such an autonomous scientist, and demonstrate a proof-of-concept in any scientific or engineering domain (such as robotics, material science, biology, computer architecture etc.) of their choice.
…
After training, the agent should be able to describe the relevant experiments required to test the generated hypotheses and
analyze the results of said experiments and use them to improve its internal scientific knowledge models.Proposals must argue how the proposed approach (to all three elements described above) can induce creativity when generating hypotheses. As an example of creativity, consider how an autonomous scientist can re-discover a primitive version of AlphaFold by automatically identifying, say, the relevant knowledge of protein physics and geometry as inductive biases to develop a protein folding neural network model that upon getting more experimental data gets refined based on the skepticism of the autonomous scientist. Approaches may be based on (and not limited to) data-less neural network architectures, methods for reducing one problem to another, and altogether new theories.
We discussed the scenario of an AI-powered subsidiary of a traditional company, dedicated to scientific, engineering, or financial research in Issue #40 – I Didn’t See It Coming.
DARPA, always at the forefront of applied research, is showing you that the ideas you read inside Synthetic Work are not just elaborated scenarios conceived by creative minds, but directions actively pursued by some of the most advanced research organizations in the world.
A theoretically infinite (you still need to pay for the GPUs, and there’s a finite number of them) number of synthetic scientists, working 24/7 and accelerating scientific discovery tenfold, would transform the economy in ways that are unpredictable.
This is why one of the most knowledgeable startup investors in the world, Paul Graham, this week said on X:
JP Morgan says AI tools are starting to generate revenue for the bank.
Katherine Doherty, reporting for Bloomberg:
“In addition to efficiency and potential cost avoidance, we are seeing revenue-generating activity, and that’s really encouraging,” Teresa Heitsenrether, the bank’s chief data and analytics officer, said at the Evident AI Symposium on Wednesday.
JPMorgan set a target last year of $1 billion in “business value” generated by AI in 2023, and the firm increased that goal to $1.5 billion at its investor day in May.
…
JPMorgan is using AI to augment work done by humans, rather than seeing it as a way to replace them, Heitsenrether said. The bank can continue to grow using AI without necessarily increasing headcount to support that growth, she said. Still, it’s focused on attracting talent across the globe.“There is a keen desire to be competitive here, to be on the forefront,” she said.
As I always comment: for now. We’ll see what happens to the keyword “necessarily” once GPT-5 and its successors are available. Especially considering that the bank is already framing AI adoption in terms of cost-cutting and efficiency.
More importantly: if JP Morgan can prove that AI tools can generate substantial revenue even for highly regulated companies, it will significantly boost confidence across industries. Which means faster adoption. Which means an accelerated impact (positive or negative) on jobs.
Goldman Sachs is losing the most AI talents to other financial institutions.
As you’ll read in this week’s Splendid Edition, there’s an ongoing war for AI talent among financial institutions, with very interesting flows of people moving from very specific companies to anothers.
The biggest loser reportedly is Goldman Sachs. Possibly because of the attitude captured in the following VentureBeat article by Sharon Goldman:
Marco Argenti, CIO at Goldman Sachs — who told me in a recent interview that the leading global investment banking, securities and investment management firm has, nearly a year after ChatGPT was released, put exactly zero generative AI use cases into production. Instead, the company is “deeply into experimentation” and has a “high bar” of expectation before deployment. Certainly this is a highly-regulated company, so careful deployment must always be the norm. But Goldman Sachs is also far from new to implementing AI-driven tools — but is still treading slowly and carefully.
…
While Argenti told me that he thinks “We’re all anxious to see results right away” in areas like developer and operational productivity, as well as revolutionizing the way knowledge workers work and producing content, when I asked him what it would take to put its experimental use cases of generative AI into production, he said it required “feeling comfortable about the accuracy.” He added that this needs to hit a certain threshold “in which we feel comfortable that the information is correct and the risks are actually well managed.”
…
In addition, he said that Goldman Sachs needs a clear expectation of a return on investment before deploying generative AI into production.
As I said in previous issues of this newsletter, exactly like it happened with cloud computing, ROI calculations can be fatally misguided.
In the early days of cloud computing, over 15 years ago, I personally discussed with the top leadership of hundreds of the largest companies in the world the expectation that cloud adoption would lead to a reduction in IT costs.
It was a misguided expectation, and the ones that waited for that kind of return on the investment fell irremediably behind their competitors, and it took them a decade to catch up.
What cloud computing gave companies was an unprecedented agility in delivering new products and services that nobody knew how to factor in an ROI calculation. And, I’d argue, people still fail at that.
Fast forward to today: if your organization is trying to justify the adoption of AI technologies by calculating the cost saving, you will fall behind.
Just like cloud computing, it might even happen that AI turns out to be more expensive than the way we operate today. Time will tell. But what artificial intelligence gives your organization for sure, unquestionably today, is an unprecedented speed in execution and an unprecedented capability to scale operations.
These are intangibles that nobody knows how to factor in an ROI calculation well.
Putting this aside, there’s an additional, more important aspect to this story that is worth pondering about:
Your company’s openness to experimentation and its willingness to take risks will make or break the capability to attract and retain the most important job profiles of modern times: AI researchers and ML engineers.
People want to see that their work matters.
People want to see that they are not just there to fill slide decks until they are numb.
People want to see that they can make an impact.
If your company cannot offer that, there are plenty of others where talents can go.
When you shape, internally, your AI strategy, remember that how you attract and retain AI talents is a critical part of that strategy.
The UK Department for Education’s Unit for Future Skills puts London finance and insurance professionals on high alert for the expected impact of AI on the job market.
Delphine Strauss, reporting for Financial Times:
High-flying professionals in the City of London will be in the eye of the storm as generative artificial intelligence transforms the UK’s labour market, according to government research published on Tuesday.
The report, billed as one of the first attempts to quantify the impact of AI on the UK jobs market, used methodology developed by US academics to identify the occupations, sectors and areas that would be most affected by the adoption of AI, and by large language models in particular.
The analysis by the Department for Education’s Unit for Future Skills found the finance and insurance sector was more exposed than any other. It used a measure that looked at the abilities needed to perform different jobs and how far they could be aided by 10 common AI applications, including image recognition, language modelling, translation and speech recognition.
Management consulting was the occupation most affected by any type of AI application, followed by financial managers, accountants, psychologists, economists and lawyers.
Call centre workers topped the list of occupations most exposed to large language models, such as the software behind Open AI’s ChatGPT, the study found. Other highly affected jobs include university lecturers, credit controllers, public relations specialists and the clergy.
…
London was five times as exposed as the north-east of England to AI, on the DfE’s measure, because of its concentration of jobs in professional occupations.
The article refers to the new research The impact of AI on UK jobs and training published at the end of last week.
This research uses the same methodology applied in multiple research papers we reviewed almost 9 months ago on Synthetic Work.
This is the full list of the most exposed occupations according to the UFS:
This, instead, is the list of the least exposed occupations:
Now.
How is AI projected to impact the industries in the UK economy?
And, finally, how is AI projected to impact the UK geography?
Plenty of grim news to share with your family during the winter holidays. A perfect conversation starter with your least loved ones.
This week’s Splendid Edition is titled Hieroglyphics Didn’t Matter That Much.
In it:
- Intro
- How to keep busy during the holidays.
- What’s AI Doing for Companies Like Mine?
- Learn what Renaissance Hotels, EY, BlackRock, and Cali Group are doing with AI.
- A Chart to Look Smart
- End-of-year reports from PluralSight, Evident AI, and cnvrg.io give us a shower of charts on AI adoption trends and talent flows.
- Prompting
- Anthropic explains why Claude 2.1 failed the Needle-in-a-Haystack test. To fix the problem just use clever prompt engineering.