January 5, 2024
Free Edition
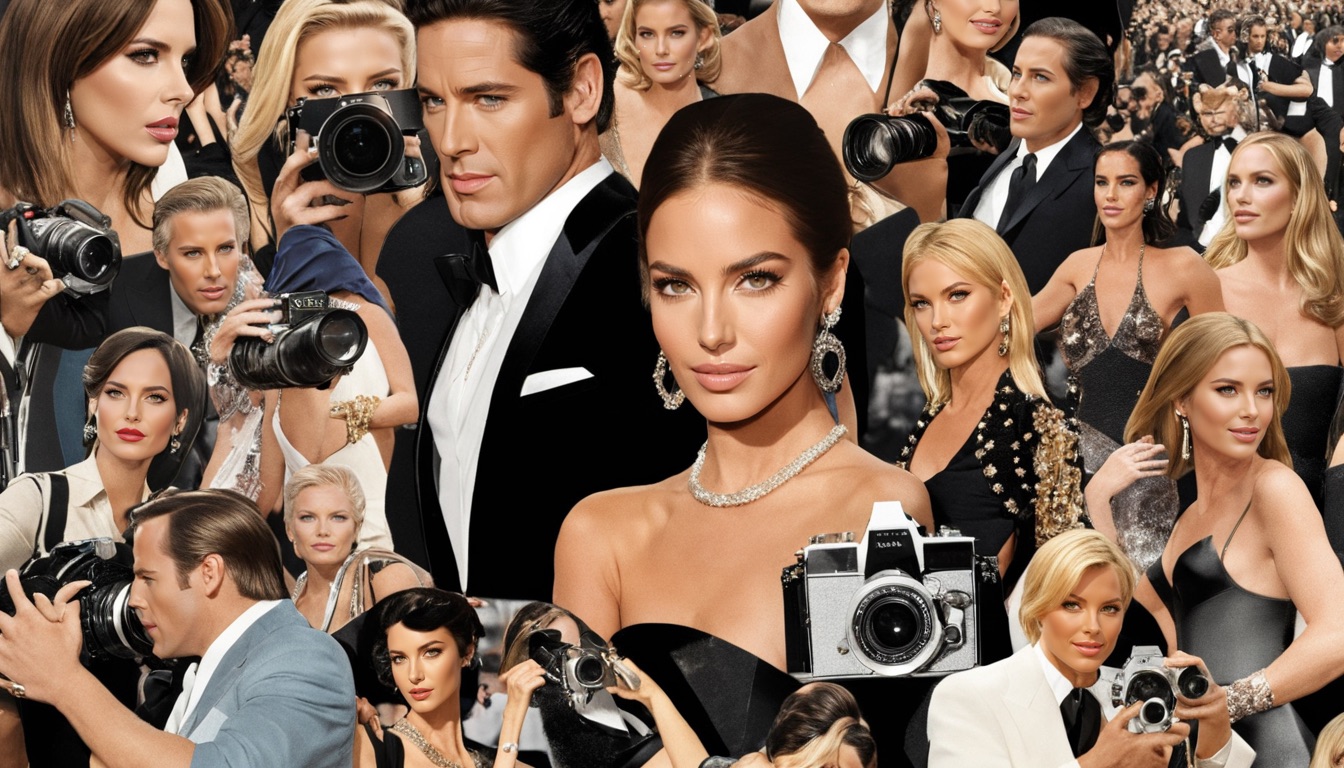
In This Issue
- Intro
- Welcome to the Post-Real Era
- What Caught My Attention This Week
- US Supreme Court Chief Justice devotes his 2023 Year-End Report on the Federal Judiciary to artificial intelligence to ponder if AI will replace judges.
- Reddit CEO reveals that his company is experimenting AI-powered moderation.
- Deepdub, a startup that uses AI to clone voice actors, launches a royalty program.
- The Way We Work Now
- The Prado museum used AI to verify the authenticity of a painting attributed to Raphael.
- How Do You Feel?
- Human supremacists will be upset in discovering that AI models can, under the right conditions, be very creative.
You need a paid membership to read this content.