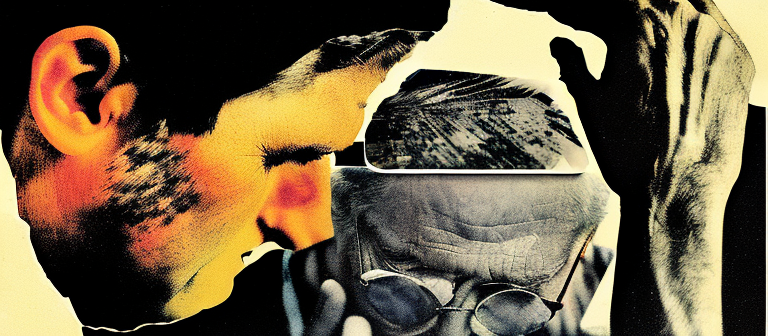
- Coca-Cola formally announces that it doesn’t give a crap about the legal issues surrounding generative AI and it’s using it like there’s no tomorrow
- Walmart is using AI to automate the tedious negotiation with suppliers. No, you can’t do the same with your significant other. Yet.
- Somehow, JPMorgan Chase & Co is trying to decode the language used in Fed meetings to find signals to trade. Politicians next?
- Three powerful techniques to reduce the number of tokens (and your bill) in the interaction with GPT-4: The Power of No, Memory Consolidation, and Alien Language Translation
Last week, I mentioned a new perk for those members of Synthetic Work with a Sage, Innovator, and Amplifier subscription: the Best AI-Centric Tools for X (or Best For) database.
That tool is not finished yet because, first, I had to create another tool: the AI Adoption Tracker.
This is something I wanted to do for 20 years, and not just about AI but about any emerging technology I focused on during my career: a tool that tracks what organizations in what industries are using what type of AI (from what technology provider) for what use case.
Tracking the technology providers that offer a solution in a given market segment is easy. The biggest challenge is keeping up with the number during the market expansion phase, and figuring out a taxonomy that is comprehensive, easy to understand, and not prone to obsolescence after two minutes.
Everybody does that, and I did it, too, 20 years ago when I created another media project called virtualization.info. And maybe we’ll do it again here on Synthetic Work, with the appropriate differentiation, if there’s demand for it.
But what really matters to people is knowing what their peers are doing with new technologies. And if those new technologies actually work.
If you are a law firm, you want to know that other law firms are using GPT-4 to generate draft documents for complex mergers and acquisitions. And you want to know if GPT-4 actually is up to the task from those law firms rather than relying on the word of OpenAI.
This much I learned in 23 years of career, especially as a research director in Gartner and as an executive in Red Hat later on.
Another thing I learned during my 15 years in Gartner and Red Hat is that announcing the intent to use a technology is very different from actually using that technology.
End-user organizations and technology vendors announce these things all the time, as a win-win public relations strategy, to raise awareness about their brands and appear as innovators.
In reality, way too often, the products and services that are supposed to revolutionize this or that company are so mediocre that they don’t even pass the initial tests and end up quietly discarded without anybody noticing.
That’s why, the AI Adoption Tracker will only list companies that went on the record for actually using AI.
For example, last month PwC announced their intent to partner with Harvey AI to use OpenAI GPT-4 to support its 4,000 lawyers in the Legal Business Solutions division. It’s lovely, but we need some confirmation that it’s happening.
Similarly, this month PwC also announced the intent to spend $1B on OpenAI technologies by working with Microsoft. It’s lovely, too, but I’ve seen too much in my career to not be sceptical at the sentence:
Once the models are fully trained and tested, Mr. Kande sees the technology being used to quickly write reports and prepare compliance documents, analyze and evaluate business strategies, identify inefficiencies in operations or create marketing materials and sales campaigns, among many other applications.
Let’s revisit once those models are fully trained and tested and the technology is actually being used as declared, shall we?
For now, the AI Adoption Tracker includes all the companies we’ve mentioned so far in the Screwed Industries section of the Splendid Edition. Going forward, it will include all the ones I didn’t have the chance to mention yet here on Synthetic Work.
The AI Adoption Tracker is available to Synthetic Work members with a Sage, Innovator, and Amplifier membership.
Consider it in beta, as it doesn’t support mobile screens (it’s quite hard to figure out a really nice way to use a tool like this on tiny screens), so be patient as I improve it.
Hopefully, it will be useful to all of you (send me feedback by replying to this email).
The groundwork done for the AI Adoption Tracker tool will become the foundation for the Best For presentation layer and for the third tool that I plan to launch soon (that one is about prompting).
Alessandro
What we talk about here is not about what it could be, but about what is happening today.
Every organization adopting AI that is mentioned in this section is recorded in the AI Adoption Tracker.
In the Food & Beverage industry, Coca-Cola formally described its adoption of generative AI technologies during the 2023 Q1 Earnings Call:
Coca-Cola is the first company to collaborate with OpenAI and Bain & Company to harness the power of ChatGPT and DALL-E to enhance marketing capabilities and business operations and to build capabilities through cuttingedge artificial intelligence (AI). Within one month of announcing this collaboration, the company launched the “Create Real Magic” platform, which allowed consumers to become digital marketeers by leveraging AI to generate original artwork with iconic creative assets from the Coca-Cola archives. The company is also exploring ways to leverage AI to improve customer service and ordering as well as point-of-sale material creation in collaboration with its bottling partners.
Sharon Goldman, writing for VentureBeat, adds some colour:
Pratik Thakar, global head of strategy for the Coca-Cola brand, said these efforts are just getting started.
“This is just a starting point,” he said of the generative AI used in the Create Real Magic campaign. “think it’s going to become mainstream.” His team is also experimenting with video tools like Runway as well as delving into how AI can work in other creative areas such as marketing.
The company’s initial foray into AI-generated art actually began last October, when it was developing its “Masterpiece” short film that aired last month
If haven’t seen it yet, this is the exceptional ad the article is referring to:
Let’s continue with the article:
“Our post-production house said they needed to use AI in order to meet their timelines and get the perfection they needed,” he said. “That’s when we looked at different platforms and used Stable Diffusion for that.”
By January, Thakar said, his team had begun experimenting with ChatGPT, Midjourney and DALL-E and realized that the generative AI space had become very dynamic. This led to the launch of a creative Aincubator, while Coca-Cola’s consulting firm, Bain & Company, brought the idea of an OpenAI partnership into the mix.
…
Artists were able to download and submit their work for the opportunity to be featured on Coca-Cola’s digital billboards in New York City’s Times Square and London’s Piccadilly Circus.“The quality of work was amazing,” said Thakar, who said the platform received 120,000 submissions of AI-generated images…
In mid-July, Coca-Cola will bring 30 AI artists on an all-expenses-paid trip to the company’s headquarters in Atlanta. “We want to work with them and create more ideas,” Thakar explained. “We have our brand licensing, fashion, digital collectibles, billboards — so many different elements can be generated from this art. I think it’s going to be fascinating.”
…
And Coca-Cola continues to experiment with AI, he added — including looking at beta versions of generative AI for music.
To accomplish what has been described so far, Coca-Cola had to fine-tune the Stable Diffusion model.
Like every other foundation model, Stable Diffusion “knows” a lot about the world, thanks to the extensive training phase that Stability AI paid for before releasing it. But it doesn’t know everything about the world.
For example, it’s unlikely that, out-of-the-box, Stable Diffusion knew how to masterfully generate paintings of iconic Coca-Cola concepts, like the original glass bottle, or a certain depiction of Santa Claus (yes, Coca-Cola invented the modern depiction of Christmas that we have today).
So what you do is take Stable Diffusion and its preexisting education and send it to a specialization school. In this case, to specialize in Coca-Cola imagery. You “expose” the AI model to all the images in the Coca-Cola archive for a certain amount of time and, at the end of this process, you get a fine-tuned version of Stable Diffusion that can generate things like this:
(this is not the winner of the competition, just one of the submissions, and a mediocre one. Coca-cola has not announced the winners yet)
I’ve been working with Dall-E 2 and Stable Diffusion since last August and I spent untold nights to master prompting techniques (which we’ll mention in the Prompting section of a future Splendid Edition) and fine-tuning techniques to extend their capability to generate new concepts.
While the complexity is going down, the learning curve necessary to achieve exceptional results remains steep for most people. I can’t tell you how many different techniques have been used, and how many hours have been dedicated to creating the Masterpiece ad you just saw.
Coca-Cola is demonstrating that there’s enormous potential in generative AI for marketing campaigns, and it’s worth it.
–
In the Retail industry, Walmart is using AI to automatically negotiate with suppliers.
Daniela Sirtori-Cortina and Brendan Case, reporting for Bloomberg:
The retail giant uses a chatbot developed by Mountain View, California-based Pactum AI Inc., whose software helps large companies automate vendor negotiations. Walmart tells the software its budgets and needs. Then the AI, rather than a buying team, communicates with human sellers to close each deal.
“We set the requirements and then, at the end, it tells us the outcome,” says Darren Carithers, Walmart’s senior vice president for international operations
Carithers says Pactum’s software—which Walmart so far is using only for equipment such as shopping carts, rather than for goods sold in its stores—has cut the negotiating time for each supplier deal tdays, down from weeks or months when handled solely by the chain’s flesh-and-blood staffers. The AI system has shown positive results, he says. Walmart said it’s successfully reached deals with about 68% of suppliers approached, with an average savings of 3% on contracts handled via computer since introducing the program in early 2021.
…
Walmart—which has more than 100,000 total suppliers—started using Pactum with a pilot for its Canadian unit. The project then expanded to the US, Chile and South Africa.
…Pactum’s software can haggle over a wide range of sticking points, including discounts, payment terms and prices for individual products
When a vendor says it wants to charge more for an item, Pactum’s system compares the request with historical trends, what competitors are estimated to pay and even fluctuations in key commodities that ginto making the item, among other factors. It then tells Walmart the highest price it thinks its buyers should accept, a figure that a human procurement officer can modify if needed.Then the real negotiation starts. Pactum’s chatbot communicates with a flesh-and-blood vendor on the other side, displaying a series of arguments and proposals the supplier can accept or reject…
“There’s so much data, so much back and forth, and so many variables that can be tweaked,” says Pactum CEO Martin Rand. “The AI bot with a human on the other side will find a better combination than two people can over email or on the phone.”
So. One human is already out of the equation. Of course, Pactum is already researching how to conduct bot-to-bot negotiations so that the other human can go home, too.
–
In the Financial Services industry, JPMorgan Chase & Co. has been creative in its use of large language models.
Lu Wang, reporting for Bloomberg:
Based off of Fed statements and central-banker speeches going back 25 years, the firm’s economists including Joseph Lupton employed a ChatGPT-based language model to detect the tenor of policy signals, effectively rating them on a scale from easy to restrictive in what JPMorgan is calling the Hawk-Dove Score.
Plotting the index against a range of asset performances, the economists found that the AI tool can be useful in potentially predicting changes in policy — and give off tradeable signals. For instancethey discovered that when the model shows a rise in hawkishness among Fed speakers between meetings, the next policy statement has gotten more hawkish and yields on one-year government bonds advanced.
…Going by JPMorgan’s model, a 10-point increase in the Fed Hawk-Dove Score now translates to roughly an increase of 10 percentage points in the probability of a 25 basis point hike at the central bank’next policy meeting, or vice versa.
The Hawk-Dove Score, also available for the European Central Bank and the Bank of England, is expected to expand to more than 30 central bankaround the world in the coming months.
JPMorgan Chase & Co. approach by two research papers published between the end of March and the beginning of April:
- Can ChatGPT Forecast Stock Price Movements? Return Predictability and Large Language Models
- Can ChatGPT Decipher Fedspeak?
Justina Lee covers both for Bloomberg:
That process is nothing new on Wall Street, of course, where quants have long used the kind of language models underpinning the chatbot to inform many strategies. But the findings point to the technology developed by OpenAI reaching a new level in terms of parsing nuance and context.
“It’s one of the rare cases where the hype is real,” said Slavi Marinov, head of machine learning at Man AHL, which has been using the technology known as natural language processing to read texts likearnings transcripts and Reddit posts for years.
…In the first paper, titled Can ChatGPT Decipher Fedspeak?, two researchers from the Fed itself found that ChatGPT came closest to humans in figuring out if the central bank’s statements werdovish or hawkish.
…
ChatGPT was even able to explain its classifications of Fed policy statements in a way that resembled the central bank’s own analyst, who also interpreted the language to act as a human benchmark for the study.
…In the second study, Can ChatGPT Forecast Stock Price Movements? Return Predictability and Large Language Models,Alejandro Lopez-Lira and Yuehua Tang at the University of Floridprompted ChatGPT to pretend to be a financial expert and interpret corporate news headlines. They used news after late 2021, a period that wasn’t covered in the chatbot’s training data.
The study found that the answers given by ChatGPT showed a statistical link to the stock’s subsequent moves, a sign that the tech was able to correctly parse the implications of the news…
When Man AHL was first building the models, the quant hedge fund was manually labeling each sentence as positive or negative for an asset to give the machines a blueprint for interpreting the language. The London-based firm then turned the whole process into a game that ranked participants and calculated how much they agreed on each sentence, so that all employees could get involved.The two new papers suggest ChatGPT can pull off similar tasks without even being specifically trained. The Fed research showed that this so-called zero-shot learning already exceeds prior technologiesbut fine-tuning it based on some specific examples made it even better.
This is the time when I remind you that a few weeks ago Bloomberg released BloombergGPT, a large language model fine-tuned with the almost infinite supply of information that the company archives about the financial world.
The models used in the research papers must be infinitely less capable than BloombergGPT.
This is also the time when I remind you that last week, we finally saw Stability AI release the first alpha of their own large language model, StableLM, and that Stability AI is run by former hedge fund managers, and that these former hedge fund managers have expressed the intention of releasing financially-focused large language models multiple times in the last few months.
When Stability AI will release one of those models, let’s hypothetically call it StableLM for Finance, the Finance community will start working on it in mass, just like the Art community worked on Stable Diffusion in mass.
In particular, you should expect one of my favourite open source projects ever, OpenBB, to incorporate the AI model and run with it.
As I said multiple times now, the Finance world will be the one most transformed by generative AI. We’ve seen absolutely nothing yet.
Every company mentioned in this section is also in the new AI Adoption Tracker.
Before you start reading this section, it's mandatory that you roll your eyes at the word "engineering" in "prompt engineering".
It turns out that even the most intelligent person in the world, Terence Tao, is using GPT-4:
So, now, you literally have no excuses to not get better at prompting.
Today we talk about how to squeeze more output out of GPT-4.
If you have read this week’s Free Edition of Synthetic Work, you know that groundbreaking new research is promising to drastically increase the length of our requests to the AI and the length of its responses back to us.
Until that time, we have to deal with the limitations of today’s version of GPT-4 (8,000 tokens or approximately 6,000 words, as 100 tokens ~= 75 words) and tomorrow’s version of GPT-4 (32,000 tokens or approximately 24,000 words).
That is more than enough if you are looking for a short answer, but your intent is to carry a long conversation with the AI, or have it generate for you a long market research like in the example I showed in Issue #8 – The Harbinger of Change, then you’ll start to feel a bit constrained.
Also, interacting with an AI that has a larger short-term memory, as I call the context window), will probably cost more, either in terms of a monthly subscription or in terms of interactions via an application programming interface (API).
For now, there are two main techniques to reduce the waste of tokens which are especially useful if your task of the day requires a lot of back and forth (the so-called turns of dialogue) with the AI.
Going forward, as OpenAI unlocks the capability for GPT-4 to “read” images, I’m sure there will be other creative ways to reduce token consumption.
The Power of No
You know those super polite answers that GPT-4 always gives you with a long preamble of platitudes that become mildly irritating after the first 3 replies?
Those. Well, those are a lot of wasted tokens.
So, the first way to reduce this waste is by teaching the AI how to answer in a different, more succinct way.
Here’s the default answer we know way too well:
Enough nonsense!
Glorious.
Of course, you have to explain this approach to GPT-4 only once, at the very beginning of our chats and never again unless we start a completely new session.
Memory Consolidation
A significantly more advanced technique to save tokens over a long interaction is helping the AI to consolidate the memory of what’s being said.
Let’s say that you have a long conversation with the AI about your business partner and you are asking how to proceed in a certain scenario.
(Notice that in this example, we are not seeking therapy. We are hoping to extract the best business practices that GPT-4 might have learned during the training phase.)
You start by giving a summary of the situation to GPT-4, which replies according to the information you have given:
At this point, in a real-world conversation with a real-world person, you realise that the initial summary you provided was quite incomplete and GPT-4’s answers miss some context to be more useful or accurate.
So, you proceed in providing additional information you deem useful to help the AI:
This is an iterative process that might have many turns of dialogue. And every time, there’s a non insignificant consumption of tokens.
Rather than proceeding in this way until you run out of memory, you can try the following method.
After a few interactions, you ask GPT-4 to summarize what is being said so far:
Then, you take that summary, and use it as a starting point for a new conversation, dramatically reducing the token consumption.
After a few more interactions, you ask GPT-4 to summarize again the conversation you had had so far. This effectively updates the first summary with more details about your interaction with the AI.
You take this new summary and use it to start a new conversation which will be eventually summarized and used to start a new conversation. And so on.
Notice that this technique is not infallible. Along the way, GPT-4 might miss important information from its summary and, as others have noted before me, GPT-4 doesn’t seem to have any way to consider what element of the conversation have more weight than others when it does its summarization.
Nonetheless, I feel like I’m back a million years and I’m watching a bunch of cells coming together to try and form the modern human memory system here. It’s as thrilling as impractical.
Yes, impractical.
I understand this is a lot of friction. This whole process could be easily automated but I don’t want to get into the complexity of third-party tools to achieve prompt acrobatics. But if you want to see some code about this, reply to this email and I’ll point you to something worth exploring.
Eventually, we won’t need to do any of this anymore. But if you need it today, the inconvenience of manually restarting the chat every time helps you a lot.
Some researchers have significantly evolved this approach with a technique called Self-Controlled Memory System, but reviewing how it works is beyond the purposes of this section of the Splendid Edition.
Translating into an Alien Language
This third method is the most powerful, but really weird. You need to be careful if you plan to use it.
Apparently, GPT-4 is capable of translating or, as some have suggested, “compressing” text of any length into a sequence of characters that are understandable by the AI and can be later decompressed.
For example:
Later on, even in a new chat session, you can ask to translate back/decompress and carry on the conversation.
This probably tells you how many words we humans waste to express ourselves. Yes, especially in this newsletter.
This technique is especially useful if you have to re-use a very long template dozens or hundreds of times a day (the latter, probably, in an automated fashion). However, that the AI community is still wrapping its head around this recently discovered capability and nobody knows how reliable it is. So, again, if you plan to use this, do a lot of tests.
Mixing together the summarization technique and the translation/compression technique is not really recommended according to some early experiments I’ve done.