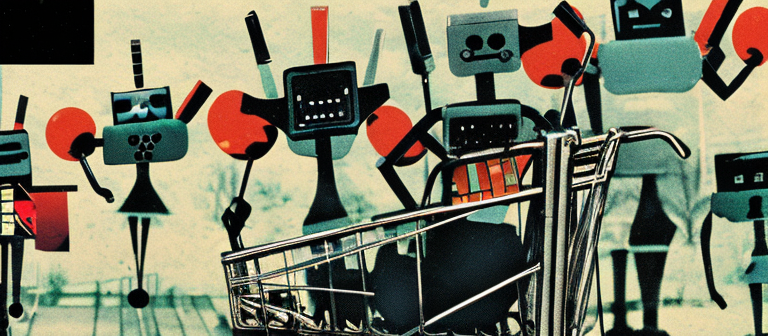
- Wells Fargo is using large language models to help determine what information clients must report to regulators and how they can improve their business processes.
- Carvana used generative AI to produce 1.3 million personalized video ads for its customers to celebrate 10 years in business.
- Deutsche Bank is using AI to scan the portfolios of its clients and suggest new investments.
- Amazon is using computer vision AI models to screen items for damage before orders are shipped to customers.
- JP Morgan Chase is preparing to launch a generative AI service like GPT-4 to offer investment advice to its clients.
- In the What Can AI Do for Me section, let’s see how GPT-4 can help us fully document a corporate procedure as mortally boring as opening a bank account.
The AI Adoption Tracker now includes almost 50 companies across 23 industries, using AI for 41 different use cases. Not bad if you think that I launched the tool just one month ago.
If your company is not a first-mover and you want to know what your industry peers are doing with AI, or if you are a technology provider and you are looking for customers, take a look at the tool: https://synthetic.work/aitracker
What we talk about here is not about what it could be, but about what is happening today.
Every organization adopting AI that is mentioned in this section is recorded in the AI Adoption Tracker.
In the Financial Services industry, Wells Fargo is using large language models to help determine what information clients must report to regulators and how they can improve their business processes.
William Shaw and Aisha S Gani, reporting for Bloomberg:
“It takes away some of the repetitive grunt work and at the same time we are faster on compliance,” said Chintan Mehta, the firm’s chief information officer and head of digital technology and innovation. The bank has also built a chatbot-based customer assistant using Google Cloud’s conversational AI platform, Dialogflow.
—
In the Retail industry, Carvana used generative AI to produce 1.3 million personalized video ads for its customers to celebrate 10 years in business.
Remember the day you met your Carvana car? We do. Using Artificial Intelligence (AI) and Generative Adversarial Networks (GAN), we’re taking each and every customer on a joyride of the day they bought their Carvana car with an AI-generated video tailored to their car-buying journey.
…
The first step was deciding what data points we wanted to incorporate into our AI-generated videos. We landed on a few — the name of the customer, the kind of car they bought, and where and when the purchase took place.Once we knew what the “pillars” of each video would be, we dug deeper, thinking about what images in a video or words in a script could represent a purchase. What time of year was it? What was going on culturally at that time? What could a customer in that area use a car for? From animating customer names to making flags for national holidays and beyond, we spent months brainstorming ways to create an all-encompassing snapshot that could help customers re-live the moment they met their car.
Then, came the art. Photographing the vehicles on our website from every angle does more than help us show them off — it allows us to make 3D models of the vehicles we offer. Leaning on a network of talented 3D modelers, animators, AI experts, and visual artists, we created a system to turn our vehicle models, AI-generated art, and 3D assets into seamlessly-animated videos with a signature style.
…
The visual component was only half the battle. We knew we wanted a narrator — but we also knew that asking a voice actor to record millions of lines to cover every possible combination of “name, vehicle, place, season, and cultural events” wasn’t exactly feasible.Fortunately, AI hasn’t only made strides in the art world. After hiring a voice actor to record a variety of lines, we used two emergent technologies — text-to-speech and re-voicing — to turn scripts into audio bites that sounded close to our actor. After applying a filter to blend the two, we ended up with the best of both worlds — AI voiceover, fluidly combined with our voice actor’s original work, to create a unique narration for every video.
…
When all was said and done, we were left with a cloud-based system capable of rendering up to 300,000 videos per hour, that’s already produced around 45,000 hours of personalized content (that’s over five years’ worth of videos watched back-to-back!).
You know what I do all day: research related to topics you read on Synthetic Work. But if you are wondering what I do all night, this is it: I test and use all the AI models that Carvana is mentioning in this blog post for different use cases, from personalized ads to financial analysis and pattern recognition to mass disinformation.
Let’s take a look at one of the generated videos:
Worth watching this whole video, pretty wild showcase of real world generative video.
Carvana created 1.3 million hyper-personalized videos.
Script, voice *and* video personalized to the specific user and their vehicle. pic.twitter.com/AMsBsd8cII
— Chris Frantz (@frantzfries) May 24, 2023
From the distinctive style of the images they generated, I’m inclined to believe that Carvana used OpenAI Dall-E 2 for the image generation portion of the work.
On this topic, it’s worth remembering that we already saw another example of personalized ads via generative AI in Issue #11 – Personalized Ads and Personalized Tutors, when the ad agency WPP deepfaked the face and voice of the Bollywood actor Shah Rukh Khan to help Cardbury celebrate Diwali with thousands of small businesses in India.
You are very likely seeing the future of advertising. Not just the online one, but also the TV one. There is a way:
So. @emroth08 tell us that free TV appliances are coming, courtesy of @ilyaNeverSleeps. The question in my mind is: what can you do with generative AI applied to the concept to revolutionize the business model (and advertising)?
First, my initial reaction: as I started my career…
— Alessandro Perilli ✍️ Synthetic Work 🇺🇦 (@giano) May 15, 2023
—
In the Financial Services industry, Deutsche Bank is using AI to scan the portfolios of its clients and suggest new investments.
With “Next best offer”, for example, algorithms continuously analyse the portfolios of Wealth Management Clients customers for risks. If, for example, a bond is downgraded, analysts issue a sell recommendation, or a region is particularly heavily overweighted, then the algorithm shows the advisor a warning.
The warning comes with a product suggestion to minimise the customer’s risk. The algorithm takes this product suggestion from the portfolios of comparable customers. “At this point, we thought about it for a long time and tried it out a lot,” says Kirsten Bremke, who came up with the original idea for “Next best offer” and now manages it. “In fact, customers prefer products that other comparable people already have. That’s when they’re most likely to switch.”
There is only a recommendation for a switch if this switch delivers a high added value to the customers. After all, switching also costs money. “Our algorithm checks that the expected benefits of switching exceed the costs,” says Bremke. “After that, the advisors then decide whether to actually pass the proposal on to the customer – after all, they’re the ones who know our customers best.”
…
At the moment, “Next best offer” is being used in Germany. The next step will be to roll it out in Italy, Spain and Asia. The model is constantly learning with Bremke and Mindt closely monitoring how customers react to suggestions and using their findings to train the model, which then improves its suggestions.
The same blog post also describes how Deutsche Bank is using AI for payment anomaly detection:
the AI model “Black Forest” analyses transactions and records suspicious cases. For each movement of capital, for example, various criteria are examined, such as the amount, the currency, the country to which it is going and the type of transaction: was it made online or over the counter?
If a criterion does not match the typical patterns, “Black Forest” reports the anomaly to the account manager. If he also finds the transaction suspicious, he forwards it to the Anti-Financial Crime department. As the feedback increases, the AI learns to classify transactions correctly and only report those where there is a real threat of a crime.
The “Black Forest” model has been in use since 2019 and has already uncovered various cases including one related to organised crime, money laundering and tax evasion.
The blog post finally mentions another future use case that is highly interesting: automated classification of environmentally sustainable deals. But it doesn’t seem the bank is using AI for that yet.
—
In the Logistics industry, Amazon is using computer vision AI models to screen items for damage before orders are shipped to customers.
Liz Young, reporting for The Wall Street Journal:
The e-commerce giant said it expects the technology to cut the number of damaged items sent out, speed up picking and packing, and eventually play a critical role in the company’s efforts to automate more of its fulfillment operations.
…
Amazon estimates that fewer than one in 1,000 items it handles is damaged, though the total number is significant for the retailer, which handles about 8 billion packages annually.
…
Amazon so far has implemented the AI at two fulfillment centers and plans to roll out the system at 10 more sites in North America and Europe. The company has found the AI is three times as effective at identifying damage as a warehouse worker, said Christoph Schwerdtfeger, a software development manager at Amazon.
…
The AI checks items during the picking and packing process. Goods are picked for individual orders and placed into bins that move through an imaging station, where they are checked to confirm the right products have been selected. That imaging station will now also evaluate whether any items are damaged. If something is broken, the bin will move to a worker who will take a closer look. If everything looks fine, the order will be moved along to be packed and shipped to the customer.Amazon trained the AI using photos of undamaged items compared with damaged items, teaching the technology the difference so it can flag a product when it doesn’t look perfect, Schwerdtfeger said.
—
Bonus story, not yet included in the AI Adoption Tracker because it’s not a real product yet:
In the Financial Services industry, JP Morgan Chase is preparing to launch a generative AI service like GPT-4 to offer investment advice to its clients.
Hugh Son, reporting for CNBC:
The company applied to trademark a product called IndexGPT this month, according to a filing from the New York-based bank.
…
JPMorgan may be the first financial incumbent aiming to release a GPT-like product directly to its customers, according to Washington D.C.-based trademark attorney Josh Gerben.“This is a real indication they might have a potential product to launch in the near future,” Gerben said.
“Companies like JPMorgan don’t just file trademarks for the fun of it,” he said. The filing includes “a sworn statement from a corporate officer essentially saying, ‘Yes, we plan on using this trademark.’”
JPMorgan must launch IndexGPT within about three years of approval to secure the trademark, according to the lawyer. Trademarks typically take nearly a year to be approved, thanks to backlogs at the U.S. Patent and Trademark Office, he said.
…
“It’s an A.I. program to select financial securities,” Gerben said. “This sounds to me like they’re trying to put my financial advisor out of business.”
If you are interested in reviewing the trademark application, you can find it here.
Way more important than that, it’s how many AI talents JP Morgan Chase is hiring.
Again, William Shaw and Aisha S Gani, reporting for Bloomberg:
The biggest US bank advertised globally for 3,651 AI-related roles from February through April, almost double its closest rivals Citigroup Inc. and Deutsche Bank, Evident’s data showed. Eigen Technologies Ltd., which helps firms including Goldman Sachs Group Inc. and ING with AI, said enquiries from banks jumped five-fold in the first quarter of 2023 compared to the same period a year ago.
Perhaps it’s time for financial advisors to consider a career change and start learning how to train AI models.
After the overwhelming success of last week’s tutorial on how to use GPT-4 to create a presentation (and improve your narrative in the process), this week we try again with a completely different use case.
I thought “What is the thing that people hate doing the most after writing presentations?” and, of course, the answer is attending meetings. But we can’t send an AI to attend meetings on our behalf, yet.
Another detested but much-needed activity in a business environment is writing a procedure.
Procedures are the documentation of business processes. Critical to avoid single points of failure when key employees leave the company, they are also the foundation of a company’s ability to scale its operations.
But writing procedures is a tedious and time-consuming activity. The aforementioned key employee has to make explicit all the knowledge accumulated over the years. And they have to do it in a way that is clear and understandable to the people who will read the procedure in the future: new hires joining the team, colleagues that will replace the key employee after he/she leaves, auditors, etc.
And this, dear reader, is a surprisingly difficult task.
Even if, somehow, you find the strength to write a procedure, it’s hard to detail the steps necessary to do your job because so many of them have become second nature and you don’t even think about them anymore. To succeed, you really need to put yourself in the shoes of the reader and imagine what they need to know to do your job.
Humans are not exactly famous for their empathy, so AI can do something amazing here: force us to make explicit the implicit.
Even if you don’t have to write procedures, or you don’t care much about them, I still recommend you to take a look at this tutorial because you can see how some of the techniques we discussed in other Splendid Edition issues are used to get the most out of our interaction with the AI.
Let’s write a procedure on how to open a corporate bank account.
Goosebumps, I know.
I simply supposed that most companies need to open multiple bank accounts throughout their lifecycle and, surprisingly, this seems to be a phenomenally complicated process in large organizations.
Let’s get what you need
Differently from the how to write a presentation tutorial, in this case, I’m using the vanilla GPT-4 model, available as part of the ChatGPT Plus subscription.
Just go to chat.openai.com and log in, or sign up and pay your $20 ticket to the future.
OpenAI has also released an official iOS app but (1) for now, the app is available only in a few countries, (2) a smartphone is really not ideal for what we are about to do today.
If you don’t want to pay for the ChatGPT Plus subscription, the next best thing is using the GPT-4 model that powers Bing Creative Mode inside the Microsoft Edge browser. Which is free.
If you are a Windows user, you already have Microsoft Edge and access to Bing.
If you are not a Windows user, download Microsoft Edge. Then, roll your eyes at the million popups and ads and surveys that Microsoft considers a great user experience.
Now, click on the huge Bing button in the top right corner. A sidebar will open and you’ll be able to choose a conversation style. Be sure to pick Creative Mode.
At this point, you are good to go.
Let’s ask GPT-4 to help us write this procedure
Critical for this job, is a technique we called Ask For Follow-up Questions. We discussed it in Issue #8 – The Harbinger of Change and, of course, it’s referenced in the How to Prompt section of Synthetic Work.
This is a really strong start.
Ask for follow-up questions is the mechanism that will allow GPT-4 to push us to make explicit the implicit. To be sure that GPT-4 uses it to its full potential, we further clarify how the interaction will happen, implicitly suggesting that GPT-4 can ask follow-up questions on the follow-up questions:
Now comes an important trick. In the previous tutorial on how to write a presentation, you have learned that the very limited short-term memory (what’s called context window in technical jargon) of these AI models is our major constraint.
If you have read this week’s Free Edition, you know that there’s a possibility that OpenAI will release a version of GPT-4 with a huge context window, able to store up to one million tokens (approx 750K words). But, for now, we are stuck with the 2048 tokens (approx 1500 words). So, it’s good practice to keep reminding GPT-4 what was said before.
Hence, rather than just reply to the first question, as the AI suggests, we repeat the question as originally stated (a simple copy and paste will do) and then we reply:
As you can see from the screenshot, GPT-4 is following the rule we defined and asks a couple of follow-up questions.
Now, let’s see what happens if I try to dodge one of those follow-up questions. The value of the AI is that it pushes me to answer everything and so, to be truly useful, it should keep asking until I reply to everything.
Exceptional job. Not only, GPT-4 didn’t let go, but it also further expanded its original question so that I’d have more context to answer.
I didn’t do it in this tutorial, but at any point in time, you can ask GPT-4 to clarify what it means with a specific question.
You can also decide to not answer certain questions at the moment and postpone it for later. Here’s an example:
In this particular case, perhaps, there was not much to explain about the reason why each step is necessary. But, in other procedures, it might be a critical context for new owners.
I would have preferred if GPT-4 had asked me to explain the reason why I need to do each step, question by question, rather than all at the end, but it’s easy to fix with a slightly different prompt.
OK. At the end of this interaction, GPT-4 is ready to write the procedure for me. I just need to be a bit pushy for it to do it:
As you can see, the description of the procedure is not much different from the interaction we just had. What matters is not much the output, but the fact that the interaction forced me to think about and clarify the steps necessary to execute the process and, perhaps, go and find the information I was missing.
This is the enormous value.
But if you want a more impressive output, GPT-4 still has in memory all the knowledge you have shared with it, plus all the knowledge accumulated during its training phase. You can ask it to make the document more elaborate, more formal, divided into sections, etc.
I’d rather ask to create a table out of it, for easier consultation:
And that’s it.
Now you can force every employee in your company to use this approach and download their knowledge into a procedure before you mass fire them and replace them with AI models. Super useful.
And…are you seated?…you can also use this approach to force a really good cook to write down a detailed recipe that normal people can actually follow.
If this use case was excruciatingly boring, feel free to reply to this email and suggest something else you’d like to see in a future tutorial.
If I don’t hear from you, the next one might be about how to use AI to brush your teeth. You’ve been warned.