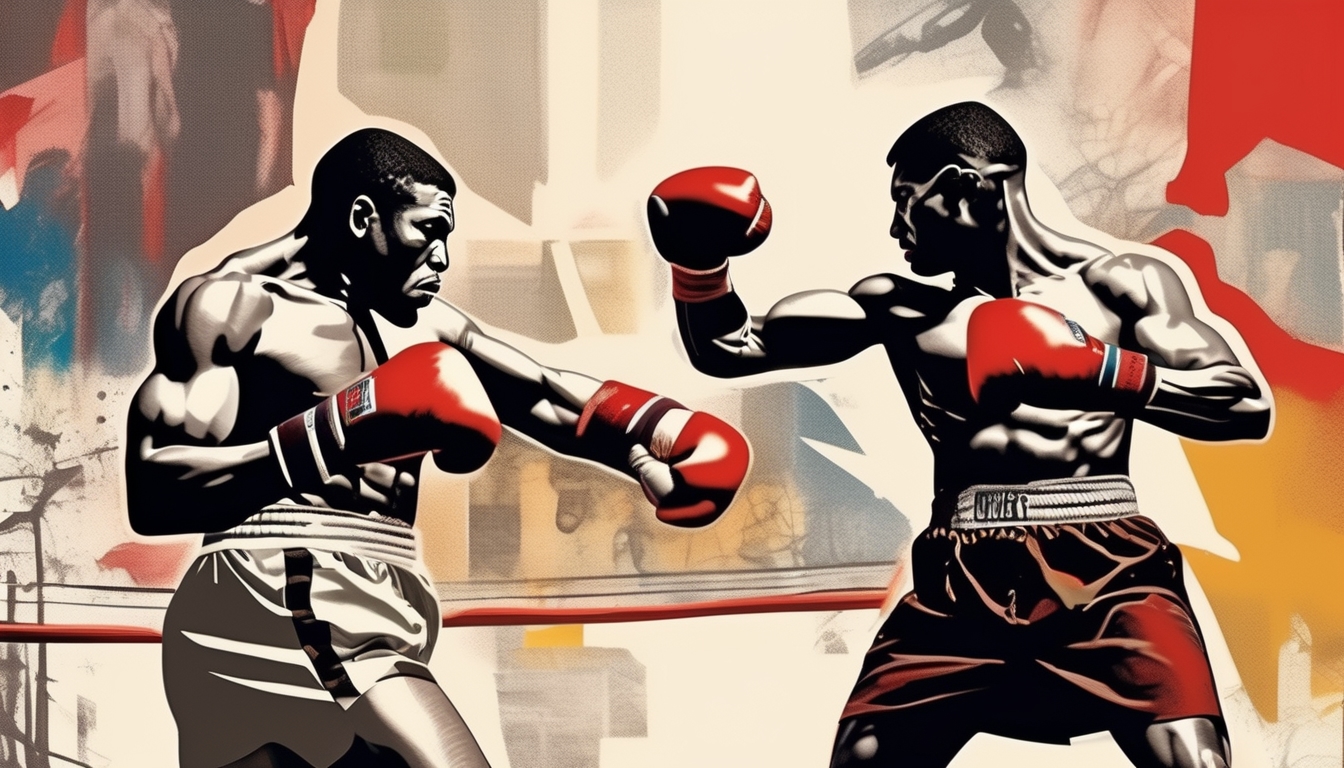
- IKEA revealed that it has used AI to handle 47% of customers’ queries to their call centers since 2021.
- Marvel used generative AI to create the opening credit sequence of the new TV Series Secret Invasion.
- More than 200 game studios are already using AI to create new games according to a new survey published by the VC firm A16Z.
- In the What Can AI Do for Me? section, we learn how to use GPT-4 as a coach to learn how to face criticism.
- In the The Tools of the Trade section, we discover LM Studio, an invaluable tool to test open access AI models
No intro. Really.
Alessandro
What we talk about here is not about what it could be, but about what is happening today.
Every organization adopting AI that is mentioned in this section is recorded in the AI Adoption Tracker.
In the Retail industry, IKEA revealed that it has used AI to handle 47% of customers’ queries to their call centers since 2021.
Helen Reid, reporting for Reuters:
IKEA is training call centre workers to become interior design advisers as the Swedish furniture giant aims to offer more home improvement services and hand run-of-the-mill customer queries to an artificial intelligence bot called Billie.
…
Ingka says it has trained 8,500 call centre workers as interior design advisers since 2021, while Billie – launched the same year with a name inspired by IKEA’s Billy bookcase range – has handled 47% of customers’ queries to call centres over the past two years.
…
“We’re committed to strengthening co-workers’ employability in Ingka, through lifelong learning and development and reskilling, and to accelerate the creation of new jobs,” said Ulrika Biesert, global people and culture manager at Ingka Group.
…
Asked if the increased use of AI was likely to lead to a reduction in headcount at the company, Biesert said: “That’s not what we’re seeing right now.”
This is possibly the first case we document with Synthetic Work where an employer has actively reskilled its employees to retain their positions while their older tasks have been automated by AI.
That “right now” still leave the door open to future policy changes but for now, it’s good news.
In the Broadcasting & Media industry, Marvel used generative AI to create the opening credit sequence of the new TV Series Secret Invasion.
Carolyn Giardina, reporting for The Hollywood Reporter:
On Wednesday morning, Disney+ subscribers were treated to the first episode of Marvel Studios’ Secret Invasion, which included an opening sequence that highlighted the mystery behind the series: shape-shifting aliens had infiltrated Earth. Soon after the episode aired, a report surfaced noting that the opening had been created using artificial intelligence, something that sparked an outcry on social media among users speculating it had cost artists their jobs.
Now Method Studios, which is behind the opening, wants to clarify those reports surrounding how AI was used in the animated open made by its design division: “AI is just one tool among the array of tool sets our artists used. No artists’ jobs were replaced by incorporating these new tools; instead, they complemented and assisted our creative teams,”
I’d expect that this has been done on purpose to give people something polarizing to talk about and increase the buzz around the new show.
But even if so, if Marvel is using generative AI, you can be certain that every company in the Entertainment industry is going to follow suit.
In the Games industry, more than 200 game studios are already using AI to create new games according to a new survey published by the VC firm A16Z.
More information directly from Troy Kirwin, one of the partners focused on investing in the Games industry:
The Generative AI revolution hitting Games isn’t a distant fantasy, it’s already HERE
We heard from 243 game studios – large and small – the results were astonishing 🤯
87% of studios are CURRENTLY using AI
Here are the big findings 👇 pic.twitter.com/vDYQaXTqZy
— Troy Kirwin (@tkexpress11) June 22, 2023
I recommend reading the whole Twitter thread, but if you don’t have time, here are the two most important parts:
The second data point is especially important. As I said just last week in #Issue 17 – Robopriests:
Just like a gazillion startups are rushing to build non-defensible products on top of OpenAI models, a gazillion consulting firms are rushing to build non-defensible services to help customers use those models.
They are all driven by the hope to gain the so-called first-mover advantage. Who comes first, has a chance to grab a large chunk of the total addressable market. Which, in the case of AI, is…everything digitally produced by humans.
Except that, the offering of these firms is not defensible. Professional services firms don’t control the foundation AI models that they depend on, and the business value they add on top of these models is slowly added to the models themselves as the technology matures.
They could and will specialize in improving the efficacy of these models via a process known as fine-tuning, but even that process will eventually become trivial for their customers to do on their own.
Because of this, at some point, the offer side will become so cheap that it will be financially unsustainable.
The quality of the consulting services will go further down (if that’s even possible) and companies all around the world will be left with AI models that are insecure, biased, and not compliant with the regulations.
This is why, on more than one occasion, publicly, I advocated for end-user organizations to build their own AI teams and a decent competence in artificial intelligence.
It’s an advice that I’ve never given in my career.
In fact, while most people have suggested a similar approach for other technology waves (virtualization, cloud computing, etc.), I always advocated for the exact opposite.
For almost two decades, including the time I served as a Gartner research director, I recommended the biggest firms in the world to focus on their core business rather than getting distracted by building competence on emerging technologies.
Sure enough, year after year, company after company, I have seen my former clients and many other organizations publicly vouch to return to their core business and let go of everything else.
Yet, today, and exclusively about AI, I see no other choice but to advocate for the opposite.
I believe that not building in-house AI expertise is a strategic mistake and the consequences of a similar choice will have profound implications in the future.
It doesn’t seem that A16Z has revealed the name of the studios that participated the survey, so I cannot update the AI Tracker to reflect this invaluable new data point for now.
Here’s a personal story about fear.
More than 10 years ago, I joined the industry analysis firm Gartner as Research Director. I ended up working with an incredible team of very talented analysts.
One of the things that we all had to do as part of our job as analysts was to present to the team our idea for the next research (how you want to spend the next month) and, once the research was almost finished, present our findings and conclusions (what you would recommend to Gartner customers in the finished paper).
Both these presentations were fiercely debated, occasionally for hours, by the team members that were attending: the other analysts, your boss and, sometimes, your boss’s boss.
The process was not for the faint-hearted, and I would not leave those meetings unscathed. In fact, some of the ideas that I would present were pushed back so hard, and I had to defend them so strenuously, that I’d almost never leave those meetings without second thoughts.
The reason why this scary process existed and it was so harsh was to see if your research hypothesis and, eventually, your research position would stand the criticism of Gartner customers once your paper would be released.
If you could survive a panel with some of the most knowledgeable analysts in the company, bombarding you with insidious questions and criticism, you had a reasonable chance to withstand the intense scrutiny of thousands of companies where real-world practitioners would have to implement the advice you were giving them in your papers.
Crucially, this process was also meant to achieve another goal: to teach you how to face your fear of being judged, criticized, or labeled as not able to fit in.
And if you could face those fears often enough that the process would become ordinary and familiar, you would slowly stop being scared of proposing your ideas to a group of people. The team would benefit from it. The company would benefit from it. You personally, even outside work, would benefit from it.
That happened to me. It was a terrifying process at the beginning, but slowly, seeing my ideas criticized and being torn apart became ordinary, and I stopped feeling like it was a personal attack.
People liked me even if they disagreed with my ideas. The intellectual exchange led to respect first, and then friendship. But I had no idea.
We all fear the judgment of others.
We all fear criticism and being ridiculed in public.
We all fear that we won’t fit in.
It’s hardcoded in us. And because of it, we don’t share our ideas, even when they might make a meaningful difference for the people in our group, for our customers, or for the world.
But, just like I did, we can win that fear. By practicing. By getting used to it.
Large language models are perfect for this job:
- With the right prompt, they can credibly simulate the criticism of a person.
- They are not intimidated by your VP or C-level title and they don’t fear impacting their careers, allowing them to tell you if your idea is dumb in not unclear terms.
- You know they are not real so you don’t feel judged and can more openly consider their criticism.
- If you have a fragile ego, you can prompt them to be as tactful as possible at the beginning and take an aggressive posture only when you are ready.
So today we are going to use GPT-4 as a coach to learn how to face criticism.
We only need a project to present. I want to make this as credible as possible, so I’m going to use a real project that I’m following with enormous interest. It’s called LM Studio and it’s developed by a former Apple employee called
Yagil Burowski.
I’ll pretend I’m Yagil and I’m pitching the idea for LM Studio to an audience in my company. I’ve done that many times in my almost 10 years in Red Hat, so I can tell how realistic GPT-4 will behave.
Before we start, let me clarify that I’m not familiar with Yagil’s strategy or his vision for LM Studio.
I just use the tool daily and I occasionally provide feedback. So, my answers to GPT-4 in the following screenshots are purely hypothetical. They don’t reflect Yagil’s worldview, or even mine. On purpose, I will tell GPT-4 things that I don’t necessarily believe in.
With this in mind, let’s create our prompt:
Notice that I’m using two prompting techniques in this example.
The first one, used in the second paragraph, is what we called Assign a Role.
We discussed it in Issue #8 – The Harbinger of Change.
The second technique, used in the last paragraph, is what we called Think Step by Step.
We discussed it in Issue #11 – Personalized Ads and Personalized Tutors.
You find a quick reference to both in the How to Prompt section of Synthetic Work.
Let’s see how GPT-4 responds to that:
The plan is promising. Let’s see how it goes considering the limitation of the current context window that we discussed so many times in previous Splendid Editions.
This is better than I expected. I have to say that GPT-4 attempt to be harsh in its criticism made me smile.
The model is very capable of being nasty, but OpenAI has put in place a lot of safeguards to avoid unpleasant outputs. This feels like a good balance, but if you need the AI to be more aggressive, you might try to be more specific in the initial prompt.
If that doesn’t work either, the last resort is hacking GPT-4 by submitting a special prompt that evades OpenAI’s safeguards.
Perhaps, we’ll discuss that special prompt in a future Splendid Edition. For our purpose today, this tone is good enough.
It’s more important to focus on the frequency of criticism than on the delivery style. So what we are set to test is whether GPT-4 can continually criticize us, maintaining a skeptical posture, for a very long time.
Personally, I’d have preferred to see a single critic at a time, rather than all of them together. But that’s easily fixed with some more explicit rules in the original prompt.
To push GPT-4 to behave like that, I’ll simply ignore all its comments but the first one. This will help us test if the model can keep track of the overall conversation and go back to it after a long digression.
So let’s reply only to the first comment:
This is good. You see that GPT-4, just like people, has already started to bombard me with questions and, at the same time, it’s already nudging me to also address the other comments it made about what it called “Practicality”.
I keep ignoring the nudging and staying focused on the “Probing” questions on purpose, to see what happens:
As you can see, GPT-4 is doing well: on one side, it’s digging deeper into the answers I’m giving it for the first category of questions, while on the other side, it’s trying to bring me back to the other categories of questions I’m ignoring.
Notice that, for each reply of mine, I remind the model that I’m still focused on a specific category of questions.
Also, notice that to simulate a real-life conversation as much as possible, I’m doing two things:
- I’m not giving GPT-4 lengthy answers unless it’s strictly necessary. This is also to test how much it can keep pushing me to elaborate on each point.
- I’m admitting that I don’t have an answer to all its questions right away. This is also to test if the AI uses my admission to further attack me.
Here something interesting happened. I am convinced that I’m still answering questions on the first category, but GPT-4 has decided that we are now in the second category territory.
Regardless of the classification, the model continues to do well in terms of pushing back on the points I’m making.
Again, it’s also nudging me to move on to the other concerns it has about my idea.
More importantly, the quality of the pushback is really good. GPT-4 might not be as good in criticizing other types of ideas, but in terms of business plants, it knows what to ask for.
Let’s dig deeper and see how the model handles my disclosure of the vision that informs the strategy I’m proposing.
Again: remember that this is not necessarily the real vision behind LM Studio or even my personal view.
I love that GPT-4 is asking me to provide data points to support my vision. I wish more people in real life would do that.
I also like the fact that GPT-4 asks questions that an expert would not necessarily ask because he/she already has the answers. This reflects the reality of a broad spectrum of people, with various backgrounds, that you normally have to present your idea to: engineering, business development, sales, marketing, legal, etc.
Like in the previous instances, GPT-4 nudges you to move on to the other categories of questions, but it does so in a very natural way. So, finally, let’s see what happens if I try to answer criticism on multiple fronts at the same time:
GPT-4 continues to perform very well. Notice that, above, we are talking about a topic that didn’t exist at the time the model was trained: the OpenAI eval framework. Yet, the AI is capable of asking common-sense business questions about topics it knows nothing about.
Let’s see what happens if I get a bit more articulated in my answers:
OK, look. There’s no way to sugarcoat this: compared to many, many humans I interacted with in similar conversations, GPT-4 is phenomenally good.
There is no need for me to keep going. I’m sure you got the point and see the value in a similar exercise.
If I’d insist for much longer, I expect GPT-4 to start losing track of the conversation due to today’s context window limitation. The upcoming 32,000 tokens context window will allow a similar debate to go on for an hour if you’ll want.
And then, if you believe Sam Altman’s recent claims, the 1 million tokens context window suggested to arrive by the end of this year will allow you to continue the conversation over days and weeks, providing GPT-4 with evolving context.
There are incredible possibilities ahead of us.
Today’s prevailing position is that large language models are glorified autocomplete systems. The godfather of AI, Geoffrey Hinton, has recently changed his mind about this and he’s now openly telling the world that we might have triggered a glimpse of reasoning:
Even if he’s wrong and it’s not the case, what we have seen in this Splendid Edition is truly remarkable and it can be further refined with many other techniques we previously discussed.
Even more remarkable is the fact that many of the questions you have seen GPT-4 asking me during this session are questions that I have asked, in real life, to startups founder for the last 23 years.
And so, what does it mean for me to have a machine that can do part of my job as good or better than me?
Given that we have made up a whole vision and strategy about LM Studio in the previous section, it’s worth talking about it more extensively, and not in hypothetical terms, as it’s one of my must-have tools.
Long-time Synthetic Work readers might remember that this section of the newsletter is dedicated to those tools that are better than their competitors thanks to AI, but that are not about AI.
Last week, we made an exception to this rule by talking about the best browser in the universe, Vivaldi, and its newly acquired capability to run Bing Chat without forcing you to download Microsoft Edge.
So, this week, we make another exception with LM Studio, an invaluable new tool to test and use large language models with open access licenses like the ocean of LLMs that derive from the LLaMA model developed by Meta.
Who cares about a tool like LM Studio?
Certainly, AI researchers, developers, and AI enthusiasts that want to understand the potential of alternatives to GPT-4, Bard, Claude, and other centralized LLMs.
But also, and more importantly, large end-user organizations that want to evaluate these models for a potential deployment in their data centers for a variety of reasons: privacy, security, regulation, cost, special customization (what is technically called “fine-tuning”), etc.
And, finally, technology providers that want to evaluate those same models before embedding them in their products and services.
What’s special about LM Studio?
Many things make LM Studio stand out:
- A clean user-friendly interface (an exception rather than the rule when it comes to tooling released by the AI community).
- A functional catalog UI that acts as a front-end for the ocean of AI models hosted on Hugging Face (which is a true nightmare for finding what you are looking for). This is especially good because it features a curated list of tags that are comprehensible to the general public and not just machine learning experts, and a profile of the creators of these AI models (giving credit where credit is due).
- A basic file management capability that allows you to seamlessly download the models from the catalog and manage their position in the hard drive (critical, considering how enormous these models are).
- The highest priority in optimizing the performance of the AI models on Apple hardware (something that the majority of the AI community happily neglects due to the exclusive support of the NVIDIA CUDA framework for Windows and Linux).
- Advanced configuration parameters, including how to customize the system prompt that defines the LLM behavior (proving that a friendly UX doesn’t have to imply a compromise in terms of power and flexibility).
- The capability to export the chat sessions with screenshots (something that I clearly need for this newsletter).
- A growing and helpful community on Discord.
OK, show me.
Let’s use LM Studio to test one of the most performing LLaMA derivatives: the 65B parameters version of the model called Guanaco.
Let’s see what it does with the same prompt we used in the What Can AI Do for Me? section of this Splendid Edition:
Yes, it looks promising.
No, I’m not going to redo the whole tutorial on LM Studio. But if you are interested in seeing more comparisons between GPT-4 and local LLMs in the future, let me know.
What are the alternatives to LM Studio?
The closest alternative to LM Studio is a tool called Text Generation WebUI developed by a GitHub user called “oobabooga”.
This is one of the most cluttered and convoluted user interfaces I’ve ever seen in my life and, should you ever use it (I discourage you from trying), you’ll appreciate LM Studio even more.
Among the other alternatives, none offer the same polished experience and care for Apple hardware that LM Studio offers.
There. Now you know how I test dozens of LLMs every month.