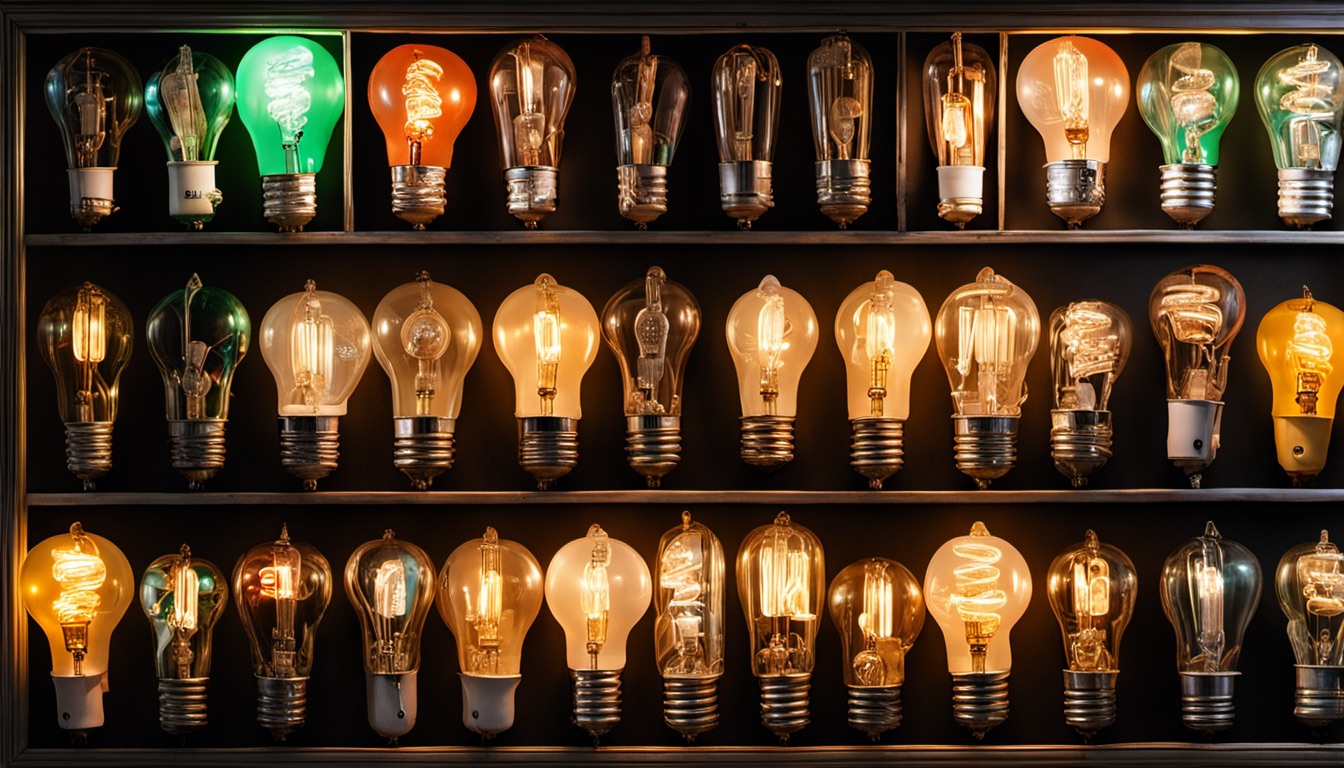
- The ad agency WPP uses generative AI to create job postings, draft communication messages to attract new talent, and more.
- The London Stock Exchange Group is testing generative AI models fine-tuned in collaboration with Microsoft.
- Tinder is using AI to automatically select the best picture from the photo album of a user.
- Australia’s Home Affairs Department used ChatGPT for software development and other tasks for a period.
- In the What Can AI Do for Me? section we see how GPT-4 can be used to generate perfectly legit business ideas. For real.
As I said in this week’s Free Edition, thank you to all of you for supporting Synthetic Work in these first 6 months.
I won’t repeat what I said there. I’ll rather say that nothing is more valuable than your feedback. The newsletter morphed quite a bit from the first issue, mainly based on your input.
So, that feedback remains critical to understand how Synthetic Work can deliver more value to you.
If you have a moment, reply to this email and share your thoughts.
I’m especially interested in what would you need to see to make you say “Oh, finally!”
Thank you and onward.
Alessandro
What we talk about here is not about what it could be, but about what is happening today.
Every organization adopting AI that is mentioned in this section is recorded in the AI Adoption Tracker.
In the Advertising industry, the ad agency WPP uses generative AI to create job postings, draft communication messages to attract new talent, and more.
Patrick Coffee, reporting for The Wall Street Journal:
“I’ve been doing this a long time, and this is probably the most pivotal thing I’ve seen that will disrupt recruitment,” said Shannon Moorman, global head of talent acquisition and executive search at advertising holding company WPP.
…
The most immediate use for tools like ChatGPT and Google’s Bard lies in automating portions of repetitive tasks: writing direct messages to candidates and creating outlines for job listings. In both cases, AI-generated content can then be tailored by humans to better describe the sort of highly specialized and tech-focused roles that are increasingly sought by marketers.ChatGPT “gets you maybe 40% of an outline, and from there you have to really fine-tune it,” said Zach Canfield, associate partner and director of talent at ad agency Goodby Silverstein & Partners.
…
Marketers frequently pursue international candidates for top creative roles. For them, generative AI can summarize the complex legal documents required to navigate U.S. immigration law, as well as help recruiters more fluently communicate with these candidates.
…
“With candidates, it is a two-way street. Sometimes my Spanish is stronger than their English, so I do believe [AI] gives some comfort and credibility and builds rapport both ways,” said Sasha Martens, president of creative recruiting firm Sasha the Mensch, who often uses Bard and other AI tools, such as translator DeepL, to more surely use local slang when recruiting outside the U.S.
As a side note, DeepL has gained enormous popularity in the last few years, surpassing Google Translate accuracy in multiple languages. I’ve recently switched to it to test it out.
More from the article, about the most unexpected use case:
Generative AI’s most compelling use for marketing recruiters, however, may lie in its ability to streamline processes such as the creation of so-called Boolean strings, which are a series of “if, then, and” prompts used to more accurately target candidates, said Moorman.
In the past, WPP recruiters may have spent hours writing hundreds of strings in order to search for, say, software developers in the Bay Area with expertise in the JavaScript coding language, said Moorman. Now, generative AI has automated much of that away, creating Boolean strings that turn up candidates who fit recruiters’ goals, sometimes suggesting more precisely aimed keywords that recruiters hadn’t explicitly asked for.
Recruiters can also tap AI to pluck a certain group of keywords from an existing job description, using those terms to create a new string and further refine the search results, Moorman said.
…
When combined with machine-learning tools such as hireEZ, generative AI can throw open a search to include online circles like Reddit sub-communities, developer forums and alumni groups for historically Black colleges and universities, said Moorman. WPP can then more quickly draw from a far broader group of candidates, she said.“One of the things that excites me about AI is that it’s actually going to open up our aperture for diverse talent pools that we otherwise wouldn’t even be aware of,” Moorman said.
…
When used correctly, generative AI can also reduce instances of bias by, for example, helping to identify the sorts of masculine-leaning terms in a job description that might dissuade women from applying, she said. (Among those words are “driven,” “objective” and “determined,” according to a WPP spokeswoman.) The ability to cull these sorts of cues could be useful to marketing organizations that have often struggled to meet their own diversity hiring goals.
Finally, on the most obvious implication:
All that automation ironically means recruiters are getting a taste of the anxiety over job security that some marketing professionals already feel. That worry echoes the scare over the rise of online job forums and platforms like LinkedIn two decades ago, said Schueneman.
These fears are likely unfounded, he said, because recruiting ultimately relies on human-to-human interaction.
On this, expectedly, I disagree.
In my experience, recruiters add value in a way that is very tangible for a candidate only at the very top. Even within the executive search domain, only a subset of the top head-hunting firms in the world makes a dramatic difference.
So, while it’s true that human-to-human interaction is critically important for the candidate, that’s exactly the area where many candidates feel the most unsatisfied.
And if a candidate feels that he or she is poorly understood, represented, or coached before meeting the hiring manager, then that candidate will not hesitate to prefer an AI to a human being.
It’s my strong belief that AI will replace people in every sort of interaction, both personal and professional, every time we are disappointed and let down by our fellow humans. It will be that disappointment that will popularize AI, not its efficiency and accuracy per se.
In the Financial Services industry, the London Stock Exchange Group is testing generative AI models fine-tuned in collaboration with Microsoft.
Nikou Asgari, reporting for Financial Times:
David Schwimmer, chief executive of LSEG, said the company was working with Microsoft to create “bespoke large language models”.
…
Banks were interested in creating their own generative AI models because they “want to make sure that none of [their] data is being used to inform any other large language models out there”, he added. Schwimmer did not name the companies that LSEG is working with.The UK exchange operator, which is suffering from a dearth of listings in London, regards AI-related products as a potential new business line. LSEG has pushed deep into the financial data business since its $27bn acquisition of Refinitiv.
Once again, fine-tuning models with proprietary data is the key to unlocking AI’s true potential. Every financial organization will want to do the same.
In the Technology industry, Tinder is using AI to automatically select the best picture from the photo album of a user.
Hibaq Farah, reporting for The Guardian:
The tool will look at a user’s photo album and select the five images that best represent them.
Bernard Kim, the chief executive of Tinder’s owner, Match Group, said AI could answer people’s concerns about which picture best represents them and take the stress away from selection.
“I really think AI can help our users build better profiles in a more efficient way that really do showcase their personalities,” Kim said in a call with investors and analysts.
He said Match Group would be launching a number of initiatives that use generative AI to “eliminate awkwardness” and make dating more rewarding.
…
Tinder has more than 75 million active users, according to Match.Mark Van Ryswyk, Tinder’s chief product officer, also hinted last month that the app may adopt generative AI – tools that produce convincing text and image on command – to help users write their bios. The bio feature is still in its early stages and only available in test markets, but it uses an AI system that suggests a personalised text tailored to the “interests” and “relationship goals” sections of users’ profiles.
Van Ryswk said a recent Tinder study had shown that a third of its members would “absolutely” use generative AI to help them build a profile.
…
Crystal Cansdale, the head of communications at the dating app Inner Circle, said the rise in AI on dating apps was the result of “dating fatigue”, or people tired of not getting the right result.She said: “It’s hard to write a bio that is perfect and not sound cringey and desperate, and AI provides an opportunity to optimise the time spent on dating apps.”
The first obvious consequence of this is that, eventually, all users will converge toward publishing the same identical pictures.
At the beginning, the paying Tinder users will enjoy access to this new AI, which will refine their profiles to maximize engagement. Then the free users will use the result of that refinement process to improve their profiles as well.
Keep in mind that people are already using AI fine-tuning to generate synthetic pictures of themselves. Those models can put your face into the most flattering Italian business suit you can imagine, in the most attractive position and lighting ever.
So, all a user has to do is download all the pictures of the most attractive Tinder profiles (which, again, will have been optimized by the Tinder AI), put them in the same folders with his or her headshot, and perform a few hours of fine-tuning to get a customized open source AI model that can churn out an infinite sequence of result-guaranteed pictures.
The cascading effect here is that Tinder users will experience a huge increase in disappointment, as the pictures they see will be increasingly less representative of the actual person they are going to meet. So, contrary to what Crystal Cansdale said in the quoted article, the “dating fatigue” will increase, not decrease.
Once that happens, obviously, the next step will be that Tinder and competitors will start offering virtual partners, just like Replika and Character.ai are already doing.
Why does it matter?
Because this approach will quickly propagate to the professional world, where companies like LinkedIn will soon offer the chance to pick a better picture for your profile and your resume, courtesy of AI classification models.
And the “great convergence” of Tinder will repeat itself.
If you think about this, LinkedIn already shows massive signs of convergence, where the overwhelming majority of business pictures look exactly the same. They all try to emulate the picture of the winners. AI will merely accelerate this process.
As a bonus story, in the Government sector, Australia’s Home Affairs Department used ChatGPT for software development and other tasks for a period.
Ariel Bogle, reporting for The Guardian:
Staff in the home affairs department have said they could not recall what prompts they had entered into ChatGPT during experiments with the AI chatbot, and documents suggest no real-time records were kept.
In May, the department told the Greens senator David Shoebridge it was using the tool in four divisions for “experimentation and learning purposes”.
It said at the time that use was “coordinated and monitored”.
Records obtained by Guardian Australia under freedom of information law suggest no contemporaneous records were kept of all questions or “prompts” entered into ChatGPT or other tools as part of the tests.
Shoebridge said this raised potential “serious security concerns”, given staff appeared to be using ChatGPT to tweak code as part of their work.
…
A Home Affairs spokesperson said the department did not restrict staff from accessing ChatGPT for experimentation until mid-May 2023, but prohibited the unauthorised disclosure of official information.“Access to ChatGPT from departmental systems remains suspended, unless an exception is approved,” they said. “No exceptions have been granted to date.”
No instances of ChatGPT being used for department decision making have been identified.
Many of the prompts staff said they used related to computer programming, such as “debugging generic code errors” or “asking chat gpt [sic] to write scripts for work”.
It doesn’t sound like a real roll-out of a technology that has been later abandoned. It sounds more like a number of government employees who were free to use ChatGPT and they did. Hence, the Australian Home Affairs Department will not be included in the AI Adoption Tracker, for now.
Nonetheless, it’s interesting how the Australian government expects its staff to maintain a full trail of all the prompts they enter into a large language model.
Obviously, doing this in a manual way is completely impractical and would discourage anyone from using the tool. It’s certain that a high-security version of ChatGPT is being developed by OpenAI to address this and many other requirements from all the governments that Sam Altman visited during his recent concert world tour.
This week we attempt something that I genuinely would have not considered worth a minute of my (or your) time just one year ago: we’ll ask the AI to come up with a list of business ideas.
Before you click the unsubscribe button of this newsletter, let me give you some context. If there was no science behind this, I guarantee you that I wouldn’t bother you.
The first paper to support our experiment is titled Ideas are Dimes a Dozen: Large Language Models for Idea Generation in Innovation, published last week by researchers at the Cornell SC Johnson College of Business, The Wharton School, and the University of Pennsylvania.
The premises are intriguing, and remind me of a conversation I recently had with one of your fellow Synthetic Work members on our Discord server (which you can join if you’d like to participate in that conversation):
Despite their remarkable performance, LLMs sometimes produce text that is semantically or syntactically plausible but is, in fact, factually incorrect or nonsensical (i.e., hallucinations). The models are optimized to generate the most statistically likely sequences of words with an injection of randomness. They are not designed to exercise any judgment on the veracity or feasibility of the output. Further, the underlying optimization algorithms provide no performance guarantees and their
output can thus be of inconsistent quality.Hallucinations and inconsistency are critical flaws that limit the use of LLM-based solutions to low-stakes settings or in conjunction with expensive human supervision.
In what applications can we leverage artificial intelligence that is brilliant in many ways yet cannot be trusted to produce reliably accurate results? One possibility is to turn their weaknesses – hallucinations and inconsistent quality – into a strength.
…
In most management settings, we expect to make use of each unit of work produced. As such, consistency is prized and is, therefore, the focus of contemporary performance management. (See, for example, the Six Sigma methodology.) Erratic and inconsistent behavior is to be eliminated. For example, an airline would rather hire a pilot that executes a within-safety-margins landing 10 out of 10 times rather than one that makes a brilliant approach five times and an unsafe approach another five.But, when it comes to creativity and innovation, say finding a new opportunity to improve the air travel experience or launching a new aviation venture, the same airline would prefer an ideator that generates one brilliant idea and nine nonsense ideas over one that generates ten decent ideas. In creative tasks, given that only one or a few ideas will be pursued, only a few extremely positive outcomes matter. Similarly, an ideator that generates 30 ideas is likelier to have one brilliant idea than an ideator that generates just 10. Overall, in creative problem-solving, variability in quality, and
productivity, as reflected in the number of ideas generated, are more valuable than consistency.To achieve high variability in quality and high productivity, most research on ideation and brainstorming recommends enhancing performance by generating many ideas while postponing evaluation or judgment of ideas (Girotra et al., 2010). This is hard for human ideators to do, but LLMs are designed to do exactly this— quickly generate many somewhat plausible solutions without exercising much judgment. Further, the hallucinations and inconsistent behavior of LLMs increase the variability in quality, which, on average, improves the quality of the best ideas. For ideation, an LLM’s lack of judgment and inconsistency could be prized features, not bugs.
At this point, the researchers set up the experiment:
we compare three pools of ideas for new consumer products. The first pool was created by students at an elite university enrolled in a course on product design prior to the availability of LLMs.
The second pool of ideas was generated by OpenAI’s ChatGPT-4 with the same prompt as that given to the students. The third pool of ideas was generated by prompting ChatGPT-4 with the task as well as with a sample of highly rated ideas to enable some in-context learning (i.e., few-shot prompting).
We address three questions. First, how productive is ChatGPT-4? That is, how much time and effort is required to generate ideas and how many can reasonably be generated compared to human efforts?
Second, what is the quality distribution of the ideas generated? We are particularly interested in the extreme values – the quality of the best ideas in the three pools. We measure the quality of the ideas using the standard market research technique of eliciting consumer purchase intent in a survey. Given an estimate of the quality of each idea, we can then compare the distributional characteristics of the quality of the three pools of ideas.
Third, given the performance of ChatGPT-4 in generating new product ideas, how can LLMs be used effectively in practice and what are the implications for the management of innovation?
By the way, these are not random academics:
We have over 20 years of experience teaching product design and innovation courses at Wharton, Cornell Tech, and INSEAD. We have used similar innovation challenges dozens of times with thousands of students.
With the prompt below, which we’ll adapt to our needs later, these researchers generated 100 ideas without providing examples of good ideas and another 100 after providing access to examples of good ideas (a technique that we called Lead by Example in our How to Prompt section of Synthetic Work).
Their first discovery is how quickly a human can generate 100 ideas with the help of GPT-4:
Two hundred ideas can be generated by one human interacting with ChatGPT-4 in about 15 minutes. A human working alone can generate about five ideas in 15 minutes. Humans working in groups do even worse.
…
A professional working with ChatGPT-4 can generate ideas at a rate of about 800 ideas per hour. At a cost of USD 500 per
hour of human effort, a figure representing an estimate of the fully loaded cost of a skilled professional, ideas are generated at a cost of about USD 0.63 each, or USD 7.50 (75 dimes) per dozen. At the time we used ChatGPT-4, the API fee for 800 ideas was about USD 20. For that same USD 500 per hour, a human working alone, without assistance from an LLM, only generates 20 ideas at a cost of roughly USD 25 each, hardly a dime a dozen. For the focused idea generation task itself, a human using ChatGPT-4 is thus about 40 times more productive than a human working alone.
Breathtaking, but only as long as these AI-generated ideas are not complete crap. In fact, per the researchers’ premise, to be useful, an LLM has to generate a few truly exceptional ideas rather than a lot of non-complete crap ones.
So, how did they evaluate the ideas generated by GPT-4?
we used mTurk to evaluate all 400 ideas (200 created by humans, 100 created by ChatGPT without examples and 100 with training examples). The panel comprised college-age individuals in the United States. Ideas were presented in random order. Each
respondent evaluated an average of 40 ideas. On average, each idea was evaluated 20 times.Respondents were asked to express purchase intent using the standard “five-box” options: definitely would not purchase, probably would not purchase, might or might not purchase, probably would purchase, and definitely would purchase
(mTurk stands for Mechanical Turk, the Amazon service)
The results?
The average quality of ideas generated by ChatGPT is higher than the average quality of ideas generated by humans, as measured by purchase intent. The average purchase probability of a humangenerated idea is 40.4%, that of vanilla GPT-4 is 46.8%, and that of GPT-4 seeded with good ideas is 49.3%. The difference in average quality between humans and ChatGPT is statistically significant (p<0.001), but the difference between the two GPT models is not statistically significant (p=0.11).
Then, they used the same evaluation framework to measure how novel the ideas generated by GPT-4 sounded, even if novelty is neither a prerequisite nor a guarantee of success:
OK.
It’s time to try this ourselves, using a slightly modified version of the prompt used by the researchers.
Given that it’s the 6th monthiversary of Synthetic Work, I think it’s the perfect time to try to generate 100 ideas to evolve this project.
Unfortunately, as a UK citizen, I don’t have yet access to the new Custom Instructions feature of ChatGPT, so I’ll have to combine the system prompt and the user prompt into a single user prompt. But if you have access to the feature and want to replicate the experiment, be sure to put the system prompt in the Custom Instructions field.
Another thing that we cannot do with the default interface of ChatGPT is change the so-called temperature of the model, in the same way the researchers have done. But you can do that by accessing the model via the OpenAI API or in the OpenAI sandbox.
The temperature in a model is a measure of how much randomness is allowed in the generation process. The higher the temperature, the more eccentric the answers. So, in theory, it’s possible to tweak the temperature of GPT-4 to generate more creative ideas before losing completely its capability to generate a rational answer.
It’s like driving somebody slightly drunk. Enough to see what he or she says without social inhibitions, but not enough to make him or her completely incoherent.
Enough. Let’s try our prompt with a completely sober GPT-4:
Here’s the first batch of 10 ideas:
Idea #1 is already in motion. As you know that I’ve started offering consulting services to companies that want to adopt AI, and I certainly thought about expanding the service to offer access to fellow experts in adjacent areas (like AI and legal).
I’m not going to comment on idea #2. You can tell me if this is a Synthetic Work service that you would be interested in.
I never thought about idea #3. Is it an exceptional one? Perhaps not.
I think about idea #4 every day, even under the shower. Technology is not there yet, but it’s coming.
Ideas #5, #9, and #10 are very difficult to implement. Idea #9 is not adjacent to Synthetic Work, in my opinion. Also: are they exceptional?
Ideas #6 and #7 are adjacent media projects tailored to provide education and cybersecurity content. As Synthetic Work evolves, you should expect more vertical content. Nothing groundbreaking.
Idea #8 is confusing in its description. It’s a mix of content tailored for the Pharmaceutical industry, similar to ideas #6 and #7, and an actual drug discovery platform, which is what Google DeepMind is doing.
Somehow, I don’t think that people would trust an organization that publishes a newsletter to discover new drugs.
Also, I don’t think I have the resources to develop a drug discovery AI model and compete with DeepMind, but it’s totally secondary.
Remember: we said that the value of using an LLM as a business idea generator is that there’s judgment involved to bias the process.
OK.
I’ll leave you with another two batches of 10 ideas each. If you see anything exceptional in there, that you would be interested in, let me know.
Ideas #11-20:
Ideas #21-30, but this time let’s force the hand of the AI to ask for something that is of general interest to the entire audience (always a terrible idea):
Especially in this last batch, I’m sure you’ll recognize a few things that Synthetic Work already does. So, I wouldn’t say that GPT-4 is doing a job worse than a human (me, in this case) at idea generation.
Of course, to have a chance to stumble on a truly exceptional idea, just like the researchers said, we’ll have to generate thousands of them. 30 is nowhere near enough.
If you try this experiment yourself, in the areas that matter to you, and you stumble on something truly exceptional, please let me know.
Now, to close.
Why is this research so incredibly important?
Because, until now, the general assumption we had was that generative AI guarantees exceptional performance when performing repetitive mechanical tasks, but really poor performance when performing creative tasks.
This assumption has become a self-fulfilling prophecy, constraining the range of things that we try to do in our interaction with ChatGPT, Claude, etc.
But, as this research shows, and our little experiment confirms, generative AI can be used for creative tasks, and it can generate ideas at least as good as the ones of the particular human being that is typing this newsletter.
And that means that we can now go out and be significantly more adventurous in our experiments, possibly unlocking even more value than we thought it was possible with the current generation of AI models.
Earlier this week, the famed venture capitalist Paul Graham, whom I often mention in Synthetic Work, tweeted: