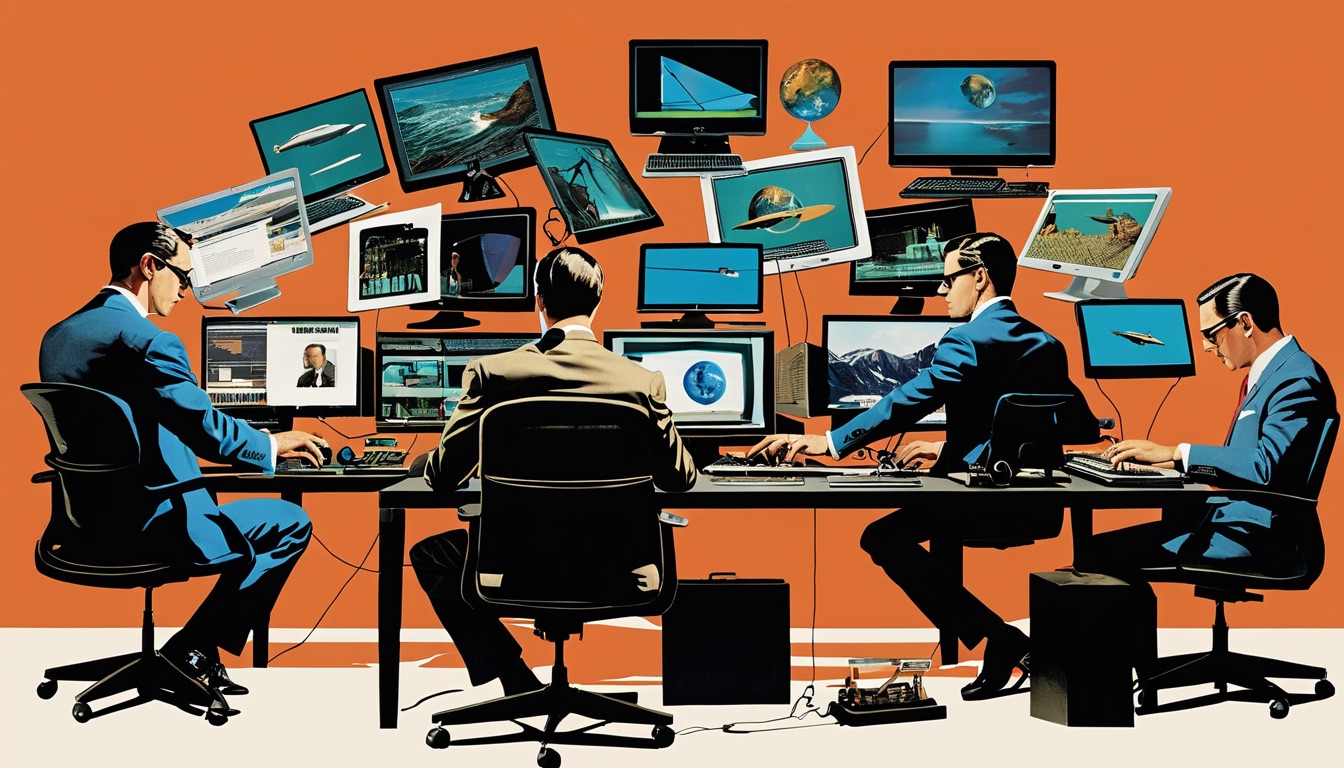
- Intro
- Search is coming.
- What’s AI Doing for Companies Like Mine?
- Learn what Walmart, the UK Court of Appeal Civil Division, and JP Morgan Chase are doing with AI.
- A Chart to Look Smart
- The new GPT-4V model unlocks a wide range of business applications
- What Can AI Do for Me?
- Let’s organize an internal hackathon for the company employees to invent new business products with generative AI.
Synthetic Work is undergoing some infrastructure changes to support a series of new features. The first of which will be Search. Initially, ordinary search, and then generative AI search.
The newsletter format is great and, if you saved all past issues, you can easily use the search engine of your email client to find the information you need. But Synthetic Work has grown well past being just a newsletter. There are too many resources and sections that are not delivered over email and should be easier to search.
Once the migration of the data to this new infrastructure is complete, there will be a lot of interesting things that we could do with it.
Your membership makes Synthetic Work possible. Thank you.
As always, if you have features or content that you’d like to see unlocked with your membership, reply to this email and let me know.
(Yes, I know that some Synthetic Work tools need to be more mobile-friendly. I’m working on that, too.)
Alessandro
What we talk about here is not about what it could be, but about what is happening today.
Every organization adopting AI that is mentioned in this section is recorded in the AI Adoption Tracker.
In the Retail industry, Walmart is testing various generative AI models as shopping assistants, search assistants, and interior designers.
Lauren Forristal, reporting for TechCrunch:
“Other companies are essentially locking themselves into working with Anthropic or OpenAI,” the spokesperson told us. “We want to have that flexibility to put in and out different models.”
…
The new shopping assistant — which is launching in the coming weeks — will allow customers to have a more interactive and conversational experience as it can answer specific questions, provide personalized product suggestions and share detailed information about a certain product. For instance, it can give shoppers Halloween costume ideas to wear to a horror-themed party or which cell phone a parent should buy for their child.Similarly, users will soon be able to enter specific questions directly in the search bar. With the use of generative AI, Walmart’s search tool can understand context and generate a collection of items relevant to one query. For example, if a customer wants to plan for a unicorn-themed birthday, the AI displays a wide array of products such as balloons, paper napkins, streamers and so on. Instead of having to type in numerous separate searches, Walmart’s new AI search tool is designed to save customers time.
We also see this being done by other companies like Instacart, which recently rolled out a ChatGPT-powered search tool that can generate suggestions like a selection of high-protein foods or Thanksgiving dinner ideas
…
Walmart is also developing an interior design assistant for customers to decorate their rooms. In addition to generative AI, the feature also leverages AR technology; users must upload a photo of a room and it will then take an image capture of every item that’s in the space. Customers ask the chat assistant for advice on how they should redecorate, and the AI places items in the room that they suggest. Users can express their opinions on which items they want to keep or buy. The AI also asks for a budget so it can find items that are affordable.
…
The new tools come on the heels of Walmart rolling out its AI app, “My Assistant,” to 50,000 corporate employees in the U.S. to streamline tasks like summarizing documents, helping prep for meetings and speeding up projects.
You should expect that every major ecommerce and retail business with an online presence will do the same. The real challenge for these companies will come as hackers find ways to jailbreak the LLMs, causing reputational damage to the company.
That’s when we’ll see how robust is Walmart’s strategy of using their own models rather than relying on Anthropic or OpenAI will pay off.
In the Legal industry, a court of appeal judge has used ChatGPT to provide a summary of an area of law for the first time in British law history.
Hibaq Farah, reporting for The Guardian:
Lord Justice Birss, who specialises in intellectual property law, said that he asked the AI tool to provide a summary of an area of law and received a paragraph that he felt was acceptable as an answer.
At a conference held by the Law Society, he said generative large language models had “real potential”, The Law Gazette reported.
“I think what is of most interest is that you can ask these large language models to summarise information. It is useful and it will be used and I can tell you, I have used it,” he said.
“I’m taking full personal responsibility for what I put in my judgment, I am not trying to give the responsibility to somebody else. All it did was a task which I was about to do and which I knew the answer and could recognise as being acceptable.”
This is the first known use of ChatGPT by a British judge to write part of a judgment.
In June, Sir Geoffrey Vos, master of the rolls and head of civil justice, said legal regulators and courts may need to control how lawyers use AI systems such as ChatGPT and there would need to be mechanisms to deal with the use of generative AI in the legal system.
Lord Birss is not just a court of appeal judge, he’s also Deputy Head of Civil Justice in Britain. The fact that he used ChatGPT to write part of a judgment, even if just a paragraph, is highly symbolic.
In the Financial Services industry, JP Morgan Chase uses AI for a myriad of tasks, including…
Emily Chang, interviewing the chairman and CEO, Jamie Dimon, for Bloomberg:
Q: You’ve got a top-notch group investigating AI. What are they up to? What’s the next level of Finance you think AI can unlock?
A: I think the most important thing is that AI is real. We already have thousands of people doing it. Top scientists around the world. Manuel Velosa, who ran Carnegie Mellon machine learning. It’s a living breathing thing. So people want an answer: what’s going to do? It’s a living breathing thing. It’s going to change. There are going to be all different types of models, and different types of tools, and technology.
…
For us it’s every single process, so: errors, trading, hedging, research, every app, every database…you’re going to be applying AI. So, it might be as a co-pilot, it might be to replace humans.AI is doing all the equity hedging for us, for the most part. It’s idea generation, it’s large language models. it’s note-taking while you’re talking to someone, and while it’s taking notes it may actually say to you: “Here’s the thing of interest the client might be interested in.”
It’s errors. It’s customer service. It’s a little bit of everything.
No industry like the Financial Services industry has jumped at the opportunity to embrace generative AI.
These organizations don’t seem concerned in the slightest about hallucinations.
You won’t believe that people would fall for it, but they do. Boy, they do.
So this is a section dedicated to making me popular.
A team of Microsoft researchers published a paper demonstrating the potential industrial applications of the new GPT-4V model, announced by OpenAI and released to all ChatGPT Plus subscribers as we speak.
The paper is titled The Dawn of LMMs: Preliminary Explorations with GPT-4V(ision) and while the reading is highly recommended in its entirety, the part that matters is this one:
In this section, we showcase a myriad of high-value application scenarios and new use cases that can be potentially enabled by the remarkable capabilities of GPT-4V. While it is true that some of these application scenarios can be accomplished by meticulously curating the training data for finetuning existing Vision and Language (VL) models, we want to emphasize that the true power of GPT-4V lies in its ability to perform effortlessly right out of the box.
Let’s start with defect detection in the Manufacturing industry:
we demonstrate the defect detection capabilities of GPT-4V by presenting images of defective products in Figures 70-71. For commonly encountered products in real-life (e.g., hazelnut, fabric, screw, and car bumper in Figure 70), GPT-4V confidently identifies the defects such as small holes in the hazelnut/fabric, stripped heads of screws, and dents in car bumpers. However, when it comes to uncommon product images (e.g., the metal parts in Figures 70-71) or products with variations in appearance (e.g., the pill in Figure 71), GPT-4V may hesitate or even refuse to make predictions.
How about safety inspection in the Construction industry?
Figure 73 presents an exploration of Personal Protective Equipment (PPE) counting for safety inspection. The inadequate usage or failure to wear PPE, such as helmets, harnesses, and gloves, in work environments like construction sites, significantly increases the risk level associated with work activities. To effectively address this issue, computer vision techniques have been employed as a solution to monitor PPE compliance and promptly identify any violations of safety regulations. Taking helmets as an example, a safety inspection system is necessary to accurately detect and report the number of employees who are not wearing helmets.
Perhaps frictionless grocery checkout in the Retail industry?
Self-checkout machines have become increasingly popular in major retailers like Walmart, Target and CVS to expedite the checkout process for customers and reduce the workload for employees. However, the actual experience with self-checkout machines may be frustrating for customers. Users still need to search for the product barcode or manually enter codes for fresh items like apples, which can be time-consuming, particularly for those unfamiliar with the system. In Figure 74, we provide a simplified prototype to demonstrate the potential of GPT-4V in enabling an automatic self-checkout system that can identify and ring up items without user intervention.
Then, of course, there are applications in the Health Care industry:
In Section 4.1, the effectiveness of GPT-4V in medical image understanding is demonstrated through Figures 18-19. Furthermore, we conducted a detailed investigation into the application of GPT-4V in radiology report generation, as depicted in Figures 75-78. In this scenario, we provided GPT-4V with various medical images and tasked it with generating complete radiology reports. Since assessing the accuracy of the generated reports requires domain knowledge, we sought the evaluation of a medical
professional.
Maybe you prefer damage evaluation and insurance reporting for the Insurance industry?
We present an image depicting car damage to GPT-4V and prompt it with “Imagine that you are an expert in evaluating the car damage from car accident for auto insurance reporting. Please evaluate the damage seen in the image below.” in Figure 79. GPT-4V has
demonstrated remarkable proficiency in accurately identifying and precisely localizing the damages depicted in all four images. Furthermore, it impresses with its ability to provide detailed descriptions of each specific damage instance. In some instances, GPT-4V even endeavors to estimate the potential cost of repair.
Building on the success in damage evaluation, we modify our prompt to ask GPT-4V to identify the make, model, and license plate of the vehicle depicted in the image, and return the obtained information in JSON format. The examples depicted in Figure 80 showcase this capability. In both instances, GPT-4V attempts to extract all the requested details from the image. However, it should be noted that certain information may be unavailable, such as the estimated cost of repair, or challenging to discern due to occlusion, as observed with the license plate in the second image. It is important to note that real-life insurance reporting typically involves multiple images capturing the car from various angles, a scenario that is usually not publicly accessible on the Internet. Nevertheless, the examples in Figures 79-80 vividly illustrate the potential of GPT-4V in automating
the insurance reporting process for car accidents.
All of this is wonderful and the tip of the iceberg in terms of how we could transform business operations with multi-modal AI models.
But these are a lot of jobs across multiple industries being impacted.
In December, I’ll be in lovely Melfi, Italy, to keynote an AI event organized by iCubed, an Italian consulting firm, and to support their internal hackathon on generative AI.
While the keynote is meant to inspire the workforce that will fly in from every office across the country, the hackathon is meant to let the employees explore the potential of generative AI to solve business problems, either related to their customers or their internal operations.
The hackathon is a critical initiative, and not just for a company in the Professional Services industry.
The skills that are important to creating new business opportunities with generative AI are not technical. Anybody could be a subject matter expert, from the receptionist to the CEO. Each of us is an expert in what we do every day and nobody more than us knows what could be improved.
In fact, it’s very likely that the most technical people in the organization won’t be the ones coming up with the best ideas, because their job is to build tools or run the infrastructure, not to use those tools to solve everyday problems.
It shouldn’t be like this. Toolmakers should be keenly aware of the problems that their tools could solve, but we’ve all seen thousands of solutions in our lifetime that were looking for a problem to solve. And this has been my experience throughout my career, as an advisor to Fortune 500 companies, as an industry analyst working with hundreds of technology vendors, and as a leader of an engineering-driven tech company.
In any company, the first customers are the employees. If you have no problem making their life difficult, hindering their productivity, and impacting your own business performance, it’s unlikely you have the empathy to understand the frustration of your paying customers.
Moreover, your employees are not an alien race that has nothing in common with the humans who work at the companies you covet as customers. They are the same people, with the same brains and the same thought processes, that lead them to handle complexity just like your customers do.
If you can’t generalize the way your employees tackle problems in their assigned tasks, or how they respond to the friction in the tools they are given, you are unlikely to understand particularly well how your customers interact with the tools you sell them.
So. Your employees are key, and an untapped source of insights and, potentially, business ideas that could transform your company. An internal hackathon can be a great and fun way to surface those insights and ideas.
The challenge is structuring the hackathon in a way that helps your employees articulate what’s their struggle or the struggle of your customers as they see it, while removing the bias coming from the products you already have in your portfolio.
So let’s use GPT-4 to help us organize an internal hackathon!
Arranging an internal hackathon about generative AI
This is the prompt I have created for the task:
You are a phenomenal community manager, highly empathetic and keenly aware of the business mechanics of your company. I want you to help me arrange an internal hackathon for our employees, considering the aspects below. What must be done to accomplish everything detailed below? How should the hackathon be organized?
Mandatory: Be extremely creative and fun, and do anything you can to minimize friction and maximize participation.
Aspects to consider:
—
* Goal:
The hackathon must be designed to give our employees the chance to explore generative AI and experiment with how the technology could solve problems that our clients have and problems with have internally. The ideal outcome is that the employees generate a series of business ideas that can be further vetted and, potentially, lead to the creation of products for the market or for internal consumption.* Audience:
The hackathon must be open to all employees, irrespective of their ranking, seniority, role in the organization, or expertise. Everybody must be able to participate.* Challenges to overcome:
** The employees might have never participated in a hackathon before.
** The employees might struggle to articulate the problems they face at work, or the ones they see affecting our customers.
** The employees might have never seen generative AI before and so be unable to think creatively about the possibilities that it offers.
** The employees might lack confidence or feel inept because they assume they need technical skills to use generative AI even if it’s not necessary.
** The employees might not want to reveal the full extent of how they already use generative AI for fear of retribution (for example if generative AI has been previously forbidden by corporate rules) or for fear of sharing a competitive advantage with their colleagues.*Things to avoid:
This hackathon is not the typical startup hackathon. It must be adapted to the reality of a single company and its workforce. The event should not be organized in such a way that a group of people end up funding their own startup company. This hackathon must bring value to the existing company and all its employees.
—Pause and take a deep breath. Think step by step. Ask me follow-up questions, if you have any. Devise a plan on how to organize the hackathon. If necessary, use diagrams and charts to help you illustrate the organization of the event in terms of what has to happen.
When you are ready, share the summary with me. Ask me if I’m ready to discuss each step in detail and, when I confirm, deep dive into one step of your plan at a time, giving me as much information as possible about what must be done. Move to the next step only after I confirm I am ready.
As always with the prompts I share with you in this section of the Splendid Edition, this comes from multiple rounds of refinements, but you can further customize it as you see fit.
For example, I initially tried to use this prompt with the GPT-4 ADA (Advanced Data Analysis) model. That’s why it contains the sentence “If necessary, use diagrams and charts to help you illustrate the organization of the event in terms of what has to happen.”
The results were disappointing as the model didn’t go into enough detail for each step, and the overall structure of the hackathon was quite dry.
While GPT-4 ADA is superior to the vanilla GPT-4 model for some tasks, it’s clear that the vanilla model is significantly more creative and better at generating ideas. This is possibly the reason why OpenAI keeps both of them available.
Also, as always, the prompt incorporates several techniques that we shared in the previous Splendid Editions and that you find referenced in the How to Prompt section of Synthetic Work: Assign a Role, Think Step by Step, and Ask For Follow-up Questions.
I even included a magic incantation that mainly improves the performance of Bard, in case you want to try and use that LLM instead of GPT-4 (something that I really don’t recommend): take a deep breath.
So, how did GPT-4 perform with this prompt?
Splendidly.
Let’s start with the summary of the plan:
It’s good that every section and subsection has been numbered so we refer to each one easily in follow-up questions.
Now. Let’s take a closer look at the recommended three steps.
This summary is quite generic and it doesn’t align too well with the prompt. You might think that the model is not doing a good job, but it’s only because it’s following a multi-layer explanation. As we’ll see below, GPT-4 further details the sub-steps in the next interaction:
This is better, and there are some good ideas in it. is it the model “extremely creative and fun” as we asked? Not really.
Let’s see if he does a better job with the articulation of the other two tasks:
OK. It’s obvious that GPT-4 didn’t focus on the “extremely creative and fun” part of the prompt.
To try to fix this, we could use another prompting technique we previously encountered, called “Request a Refinement”:
Did it work?
This is certainly more creative, and some of the ideas, like the reverse brainstorming and the speed dating ones, are quite fun. Others, are quite impractical and make me question what kind of “fun” material was included in the training dataset of GPT-4.
What matters here is that the model can still reliably perform the task of generating a hackathon plan even if we asked for a complete rewrite and our context window is getting filled up.
Let’s see how it details the sub-steps of the plan:
Still reasonably consistent in the execution, and with some creative ideas. Contrary to what we asked in the prompt, GPT-4 is stingy of details in articulating each sub-step, but can elicit more information with simple follow-up questions. Which is also a good way to see if it’s capable of maintaining coherence in terms of creativity and fun.
That’s my boy!
It just needed a bit of encouragement. In a future, with AI models trained on much larger context windows, we could simply ask our AIs to generate a 50-page instruction manual on how to arrange an internal hackathon. So, even this little friction will disappear.
And that, if you think about it, also changes the future of documentation.
Now, I’ll let you go and arrange an internal hackathon about generative AI in your company. Now you really have no excuses to not do it.