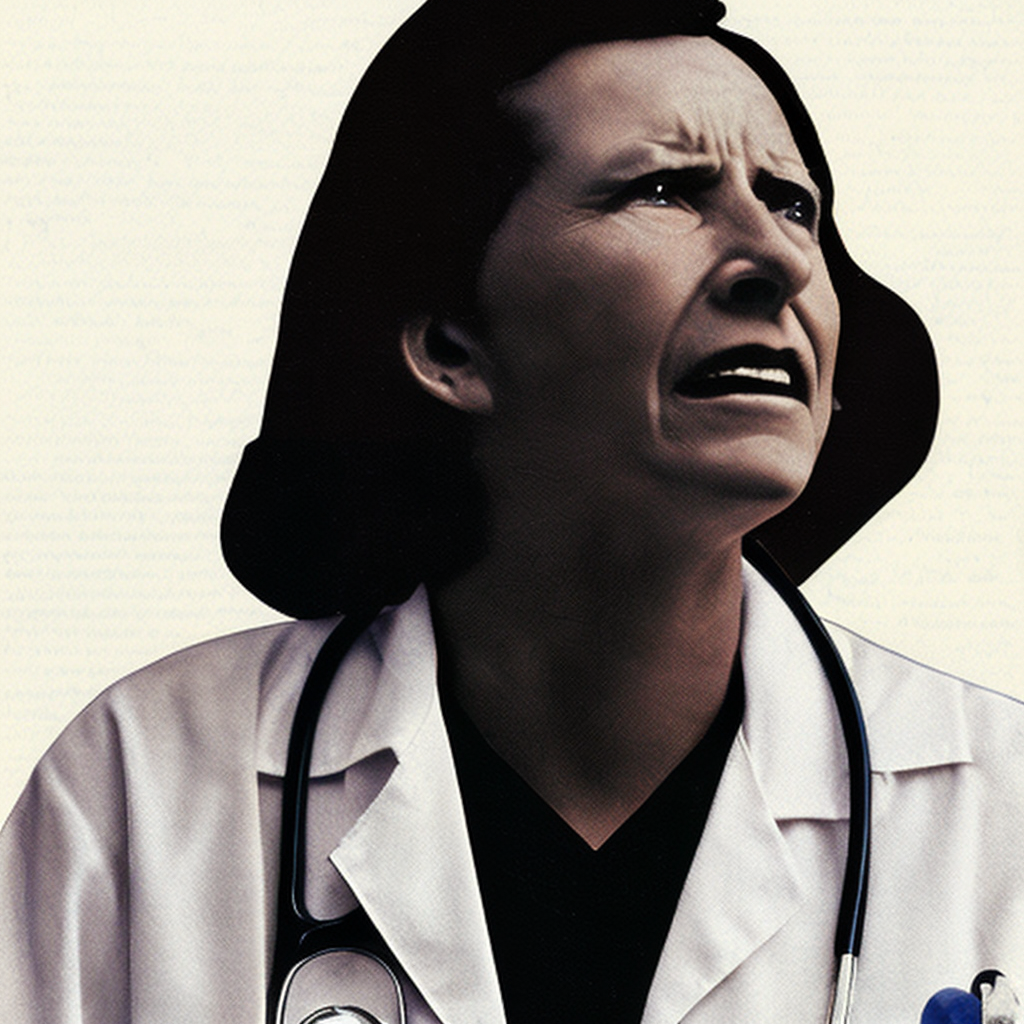
- Why not use Stable Diffusion to generate the image of a tooth growing in a person’s brain, so young doctors learn how disgusting their profession can be?
- OK. Let’s just generate chest X-rays for edge use cases. Somebody has a lovely AI model for you to download
- Somebody else is analyzing the voice of patients to spot signs of anxiety
- Google Med-PaLM 2 consistently performs at an “expert” doctor level on medical exam questions
- DeepMind predicts the 3D structures of the over 200 million known proteins to boost drug discovery
- Microsoft wants to play, too, and fine-tunes BioGPT on 15M PubMed abstracts
- Stability AI launches yet another satellite organization: MedARC
- Meanwhile, in Hungary, AI spots 22 undiagnosed cases of breast cancer
- In the US, another AI is used to identify atrial fibrillation, diabetic retinopathy, and sepsis
- The UK Medicines and Healthcare products Regulatory Agency (MHRA) says “party is over”
Last week we spend some time looking at how AI is impacting the job market across industries: Issue #3 – I will edit and humanize your AI content.
This week, we go back to our usual programming and we deep dive into the Health Care industry to understand what AI is doing there.
This is a huge topic and what I’ll say below is a drop in the ocean compared to everything that’s happening on daily basis in the Health Care industry because of AI. Also, keep in mind that this week’s release of GPT-4 will bring even more changes. But we have to start somewhere.
Take a deep breath, this is going to be intense.
Alessandro
What we talk about here is not about what it could be, but about what is happening today.
Every organization adopting AI that is mentioned in this section is recorded in the AI Adoption Tracker.
I want to start with the one thing that captured my imagination the most in the months before launching Synthetic Work: medical training augmented by generative AI.
One of the biggest challenges in any profession, but especially in the medical field, is preparing students for edge cases. Situations they will encounter rarely, if ever, during their career.
It’s harder to train a surgeon on how to remove a tooth that is growing in a person’s brain when he/she has never studied the situation.
I hope you were not eating lunch. Sorry about that.
So, to better prepare medical students to edge cases, a month ago, a group of radiologists and AI graduate students at Stanford decided to use Stable Diffusion in a novel way.
Remember that Stable Diffusion is an open generative AI system that produces images from a prompt submitted by the user in plain English. You can see some examples in this new section of Synthetic Work: photography.synthetic.work
So, these two took tens of thousands of existing images of chest x-rays, and the corresponding radiology reports, and used them to teach Stable Diffusion how to generate novel, and accurate, images of chest x-rays. A process called fine-tuning in technical jargon.
Then, they sent to Stable Diffusion novel prompts using medical terms, generating novel images of chest x-rays for arbitrary situations.
The fine-tuned model they created is called RoentGen and you can get it here.
Of course, this approach can be extended to many other branches of the medical profession and has the potential to transform the way students learn. Think, for example, what this approach could do for the students of psychology around the world:
“AI, create a clinically-realistic dialogue for my class where a doctor interviews a hypothetical patient affected by multiple personalities disorder who believes to be:
- Marileen Monroe
- Marie Antoinette
- Crudelia De Mon
- Mata Hari
- Eve
- Rita Levi-Montalcini
- Stalin
Class, cure the patient.”
You are bound to fail without AI.
Speaking of which, there is some promising work that is being done to detect signs of mental health issues by analyzing patients’ voices with AI.
Ingrid K. Williams last year reported for the New York Times:
Psychologists have long known that certain mental health issues can be detected by listening not only to what a person says but how they say it, said Maria Espinola, a psychologist and assistant professor at the University of Cincinnati College of Medicine.
With depressed patients, Dr. Espinola said, “their speech is generally more monotone, flatter and softer. They also have a reduced pitch range and lower volume. They take more pauses. They stop more often.”
Patients with anxiety feel more tension in their bodies, which can also change the way their voice sounds, she said. “They tend to speak faster. They have more difficulty breathing.”
Today, these types of vocal features are being leveraged by machine learning researchers to predict depression and anxiety, as well as other mental illnesses like schizophrenia and post-traumatic stress disorder. The use of deep-learning algorithms can uncover additional patterns and characteristics, as captured in short voice recordings, that might not be evident even to trained experts.
…
“The technology that we’re using now can extract features that can be meaningful that even the human ear can’t pick up on,” said Kate Bentley, an assistant professor at Harvard Medical School and a clinical psychologist at Massachusetts General Hospital.
This technology (which, for a change, is not generative AI) is already being tested in multiple ways. Here, for example, an implementation offered by Cigna, the American health care and insurance provider, and developed by the AI startup Ellipsis Health:
Now, tell me. Aren’t you happy at the idea that when you’ll call your doctor to book an appointment next time, your phone call will be automatically scanned for signs of stress?
No? Do you prefer Amazon (which is building its online pharmacy), Apple (which is turning its every gadget into a medical device), Google, or Facebook to do the same when you call their customer services and automatically start serving you ads about anti-depressant?
Let’s stay on this topic for a moment longer, and talk about how these technology providers are on a collision course with traditional health care providers and how many medical professions could be impacted.
This week, the AI community released a wave of new AI models. You know that one of them is GPT-4, but you might have missed Google’s announcement of Med-PaLM2.
PaLM is Google’s most powerful large language model (the same AI technology that powers ChatGPT and GPT-4), and, from this week, you’ll start to see it integrated into Gmail, Google Docs, and a number of third-party applications and services.
Google has a special version of PaLM called Med-PaLM. I’m quoting from the official announcement:
Last year we built Med-PaLM, a version of PaLM tuned for the medical domain. Med-PaLM was the first to obtain a “passing score” (>60%) on U.S. medical licensing-style questions. This model not only answered multiple choice and open-ended questions accurately, but also provided rationale and evaluated its own responses.
Recently, our next iteration, Med-PaLM 2, consistently performed at an “expert” doctor level on medical exam questions, scoring 85%. This is an 18% improvement from Med-PaLM’s previous performance and far surpasses similar AI models.
While this is exciting progress, there’s still a lot of work to be done to make sure this technology can work in real-world settings. Our models were tested against 14 criteria — including scientific factuality, precision, medical consensus, reasoning, bias and harm — and evaluated by clinicians and non-clinicians from a range of backgrounds and countries
This is not just the biased enthusiasm of a technology vendor.
At the beginning of this year, Eric Topol, an American cardiologist, professor of molecular medicine, and one of the most respected doctors in the world, wrote about the previous version of PaLM:
From the above graphic, you can see the PaLM catapulting from 50% accuracy to 67.6%, an absolute jump of 17% (a relative increase of 33%!). Importantly, the parity in medical question answering was demonstrated by 92.6% of doctors saying the MED-PaLM chatbot was right as compared with 92.9% of other doctors being correct. Furthermore, for potential harm of the answers, there was only a small gap: the extent of possible harm was 5.9% for Med-PaLM and 5.7% for clinicians; the likelihood of possible harm was 2.3% and 1.3%, respectively.
…
the opportunity to get to machine-powered, advanced medical reasoning skills, that would come in handy (an understatement) with so many tasks in medical research (above Figure), and patient care, such as generating high-quality reports and notes, providing clinical decision support for doctors or patients, synthesizing all of a patient’s data from multiple sources, dealing with payors for pre-authorization, and so many routine and often burdensome tasks, is more than alluring.
…
It’s very early for LLMs/generative AI/foundation models in medicine, but I hope you can see from this overview that there has been substantial progress in answering medical questions—that AI is starting to pass the tests that approach the level of doctors, and it’s no longer just about image interpretation, but starting to incorporate medical reasoning skills. That doesn’t have anything to do with licensing machines to practice medicine, but it’s a reflection that a force is in the works to help clinicians and patients process their multimodal health data for various purposes. The key concept here is augment; I can’t emphasize enough that machine doctors won’t replace clinicians. Ironically, it’s about technology enhancing the quintessential humanity in medicine.
And Med-PaLM2 is not the only AI model that might have a critical impact on the Health Care industry.
There is also AlphaFold 2, an AI model that enormously accelerates drug discovery by predicting the 3D structures of the over 200 million known proteins that the scientific community hasn’t investigated yet.
AlphaFold was developed by Deepmind, the AI startup that Google acquired in 2014 for more than $500 million. The company taught the AI model the structure of 100,000 proteins. As result, now AlphaFold can predict with atomic accuracy the structure of most known proteins.
The AI model is so powerful that, in collaboration with the European Bioinformatics Institute (EMBL-EBI), DeepMind was able to publish an entire database of predicted 3D protein structures online.
Look at me searching for the dopamine receptor D4!
If you are interested in understanding exactly what AlphaFold does and its implications on drug discovery (and more), here a super-nerdy video about it:
Let’s continue with our list of AI models that are or might subvert the order of the Health Care universe.
Next up is Microsoft, which announced BioGPT, a large language model that is completely trained on medical literature (15M PubMed abstracts) instead of being a general-purpose model which learns medical literature afterwards.
BioGPT is really good at answering medical questions.
But BioGPT is not just good at answering questions. It can be used to dig deep into the medical literature learned by the AI model to find quickly the relevant research about any question. Kadir Nar, a Computer Vision Engineer, has already put together a prototype on Hugging Face, a platform for AI applications, if you want to try it:
And this AI model is based on GPT-2. Two!!! And developed by Microsoft Research, not OpenAI itself.
Don’t think for a second that OpenAI has not worked on a special version of GPT-4 for the Health Care industry.
In the second issue of Synthetic Work, we saw how GPT-4 (at that time not disclosed yet) was adopted in the second largest law firm in UK: Law firms’ morale at an all-time high now that they can use AI to generate evil plans to charge customers more money.
There is no reason to believe that OpenAI will not do the same for every other industry.
Finally, in our long review of AI models for the Health Care industry, there is Stability AI, the company that funded and promoted the release of Stable Diffusion. They are also nurturing the training of an open source model through the affiliate company MedARC that was announced last month.
If you want to know more about what they are doing, the two-hour launch video will be enlightening:
In reality, there is another dozen models that would be worth mentioning but this is a newsletter, not a research paper, and Synthetic Work is about the practical impact of AI on human labour, the economy, and society, not about academic models that might or might not find a commercial application.
So let’s talk about that.
Earlier this month, Adam Satariano and Cade Metz, two reporters for the New York Times, wrote about AI detecting cancer that human doctors couldn’t see:
Inside a dark room at Bács-Kiskun County Hospital outside Budapest, Dr. Éva Ambrózay, a radiologist with more than two decades of experience, peered at a computer monitor showing a patient’s mammogram.
Two radiologists had previously said the X-ray did not show any signs that the patient had breast cancer. But Dr. Ambrózay was looking closely at several areas of the scan circled in red, which artificial intelligence software had flagged as potentially cancerous.
“This is something,” she said.
…
Hungary, which has a robust breast cancer screening program, is one of the largest testing grounds for the technology on real patients. At five hospitals and clinics that perform more than 35,000 screenings a year, A.I. systems were rolled out starting in 2021 and now help to check for signs of cancer that a radiologist may have overlooked. Clinics and hospitals in the United States, Britain and the European Union are also beginning to test or provide data to help develop the systems.
More on AI finding cancer that radiologists miss, from the same article:
Mr. Kecskemethy, along with Kheiron’s co-founder, Tobias Rijken, an expert in machine learning, said A.I. should assist doctors. To train their A.I. systems, they collected more than five million historical mammograms of patients whose diagnoses were already known, provided by clinics in Hungary and Argentina, as well as academic institutions, such as Emory University. The company, which is in London, also pays 12 radiologists to label images using special software that teaches the A.I. to spot a cancerous growth by its shape, density, location and other factors.
From the millions of cases the system is fed, the technology creates a mathematical representation of normal mammograms and those with cancers. With the ability to look at each image in a more granular way than the human eye, it then compares that baseline to find abnormalities in each mammogram.
Last year, after a test on more than 275,000 breast cancer cases, Kheiron reported that its A.I. software matched the performance of human radiologists when acting as the second reader of mammography scans. It also cut down on radiologists’ workloads by at least 30 percent because it reduced the number of X-rays they needed to read.
…
Kheiron’s technology was first used on patients in 2021 in a small clinic in Budapest called MaMMa Klinika. After a mammogram is completed, two radiologists review it for signs of cancer. Then the A.I. either agrees with the doctors or flags areas to check again.Across five MaMMa Klinika sites in Hungary, 22 cases have been documented since 2021 in which the A.I. identified a cancer missed by radiologists, with about 40 more under review.
It’s a huge breakthrough,” said Dr. András Vadászy, the director of MaMMa Klinika, who was introduced to Kheiron through Dr. Karpati, Mr. Kecskemethy’s mother. “If this process will save one or two lives, it will be worth it.”
Meanwhile, in the US, artificial intelligence is used in many other ways.
Sumathi Reddy reports for the Wall Street Journal:
At Mayo cardiology, an AI tool has helped doctors diagnose new cases of heart failure and cases of irregular heart rhythms, which are called atrial fibrillation, potentially years before they might otherwise have been detected, said Dr. Paul Friedman, chair of the clinic’s cardiology department in Rochester, Minn.
Doctors can’t tell on their own whether someone with a normal electrocardiogram, or ECG, might have atrial fibrillation outside of the test. The AI, however, can detect red-flag patterns in the ECGs that are too subtle for humans to identify.
…
Cano Health, a group of primary-care physicians in eight states and Puerto Rico, did a pilot last year using AI to analyze images from a special eye camera to identify diabetic retinopathy, a leading cause of blindness that can afflict people with diabetes. The test in four Chicago-area offices went well enough that the group now is looking to expand its use, said Robert Emmet Kenney, senior medical director at Cano Health.
…
Sinai Hospital in Baltimore is one hospital that uses an algorithm to identify hospitalized patients who are most at-risk for sepsis, a fast-moving response to an infection which is a main cause of death in hospitals.The algorithm examines more than 250 factors, including vital signs, demographic data, health history and labs, said Suchi Saria, a professor of AI at Johns Hopkins and chief executive of the health AI company Bayesian Health, which developed the program.
The system alerts doctors if it determines a patient is septic or deteriorating. Doctors then evaluate the patient and start antibiotic treatment if they agree with the assessment. The system adjusts over time based on the doctors’ feedback, said Esti Schabelman, the hospital’s chief medical officer.
OK. Let’s take a deep breath.
What does all of this mean?
Let’s start with some considerations about the Health Care industry overall.
Well. We have a handful of technology providers that need to keep growing and are looking at the Health Care industry to find new profits. And these technology providers now have a once-in-a-lifetime technology, large multi-modal models, that can examine medical data and are equally or more accurate than a human doctor.
Two things can happen.
One is that these technology providers will offer their AI systems to traditional health care providers through a consumption model (pay-per-use) or another type of licensing agreement (we’ll talk about the business models of AI in a future Splendid Edition of Synthetic Work).
The other is that these technology providers will decide to bypass traditional health care providers and replace them by building a direct relationship with the patients.
If you think that the latter scenario is improbable, think about how Apple is slowly establishing itself as a financial services provider via Apple Wallet, Pay, Pay Later, Card, etc. For now, they work with Goldman Sachs, but it’s clear that this relationship is doomed.
If the latter scenario happens, technology providers will have every interest in placing their increasingly capable AIs in front of their customers.
Why let the users book an appointment with a fallible human doctor when they can have an instant medical consultation with ChatGPT, Siri or Alexa, or search for their conditions on Google?
It’s way more convenient. It addresses the anxiety of a patient by being instantaneously ready (did you ever spend sleepless nights while waiting to see your doctor two days after?). And it gives you the illusion of being mode discreet (raise your hand if you love to be seen in the waiting room while you wait for a sexual health exam).
Of course, I’m not the only one anticipating these scenarios. Which means that regulators are preparing to constrain technology vendors in every way they can. You know, just to try and avoid the advent of an artificial Elizabeth Holmes.
In the UK, for example, the Medicines and Healthcare products Regulatory Agency (MHRA)is already saying that:
LLMs that are developed for, or adapted, modified or directed toward specifically medical purposes are likely to qualify as medical devices.
Additionally, where a developer makes claims that their LLM can be used for a medical purpose, this again is likely to mean the product qualifies as a medical device.
…
The MHRA remains open-minded about how best to assure LLMs but any medical device must have evidence that it is safe under normal conditions of use and performs as intended, as well as comply with other applicable requirements of medical device regulation.
By the time technology providers will have obtained regulatory compliance for LLMs, we’ll have reached artificial general intelligence (AGI) and we’ll have much bigger problems to solve.
And now, let’s close with a consideration about all the doctors out there.
In 2017, Geoffrey Hinton, the British-Canadian cognitive psychologist and AI luminary, a man that has won more awards than you and I will ever see on TV, told the New Yorker:
I think that if you work as a radiologist you are like Wile E. Coyote in the cartoon. You’re already over the edge of the cliff, but you haven’t yet looked down. There’s no ground underneath.
…
It’s just completely obvious that in five years deep learning is going to do better than radiologists. It might be ten years. I said this at a hospital. It did not go down too well.
Six years have passed and now we have AI spotting breast cancer, atrial fibrillation, and diabetic retinopathy that specialists struggle to see.