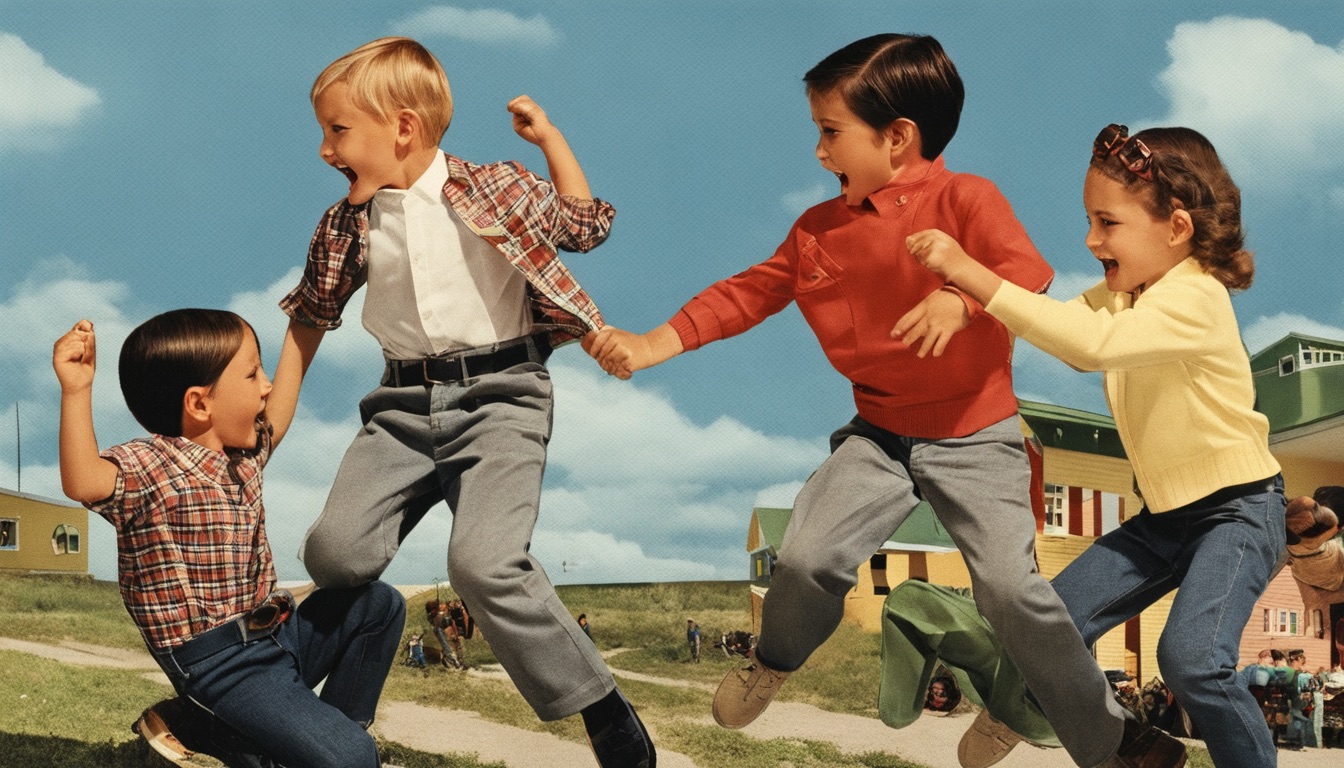
- What’s AI Doing for Companies Like Mine?
- Learn what Golborne Medical Centre, The Clueless, British Airways, and the China Ministry of State Security are doing with AI.
- A Chart to Look Smart
- How the Financial Services industry is fine-tuning specialized LLMs for asset management and document analysis.
- The Tools of the Trade
- A free online service to compare the answers of multiple LLMs to your prompts in edge use cases.
What we talk about here is not about what it could be, but about what is happening today.
Every organization adopting AI that is mentioned in this section is recorded in the AI Adoption Tracker.
In the Health Care industry, the Golborne Medical Centre is using an AI-powered stethoscope to detect three types of heart disease before the patient needs emergency care.
Madhumita Murgia, reporting for the Financial Times:
The Golborne practice, which lies over the railway track from London’s affluent Notting Hill neighbourhood, is one of 200 GP surgeries in north-west London and Wales to receive an AI stethoscope in the UK’s first deployment of the technology in primary care. Nearly half of Golborne’s patients come from non-white minority ethnic groups, who tend to face a higher risk of death from heart disease.
The tool has been licensed by medical regulators for use by general physicians and will be the first AI product that can be relied on to prescribe life-saving medication without the need for a specialist review first.
AI diagnostics promise to be a game-changer for the UK’s National Health Service and its staff, who are working under enormous strain. As the health service heads into what is expected to be one of its toughest winters, figures in October showed that people were waiting for a near record 7.7mn non-emergency appointments.
…
The goal of the AI stethoscope, designed by US company Eko Health, is to close these gaps and save the lives of heart patients who end up needing emergency care in hospital. “This is a route to treating patients early while they wait,” said Kelshiker.For every patient picked up in primary care, before emergency admission, the NHS saves £2,500. “Scaling that across just one sector in north-west London . . . will immediately unlock around £1mn per year for the system,” said Kelshiker.
The traditional procedure is often flawed. GPs carry out the first diagnosis, using regular stethoscopes and their clinical judgment. However, common symptoms of heart failure, such as fatigue and abdominal bloating, are very general and often missed during routine 10-minute appointments, which can result in patients becoming very sick.
Any heart condition diagnosis needs a blood test for confirmation as well as a referral to a specialist to perform an electrocardiogram, or a scan. Patients cannot be treated without confirmation from both those tests.
…
“There are around 30,000 excess deaths per year, while they are waiting for these sorts of tests. So that’s where the bottleneck is,” said Kelshiker. “People are dying needlessly.”
…
In 15 seconds, the Eko stethoscope can detect three types of heart disease: heart failure, which accounts for up to 4 per cent of the annual budget of the NHS; atrial fibrillation or irregular heartbeat, which is the biggest cause of stroke; and valvular heart disease.
…
Doctors will need to confirm the AI diagnosis with a blood test, which usually takes a couple of weeks to complete locally. The drugs can be prescribed straightaway.
…
The AI stethoscope though is no longer at a trial stage and needs no form filling or paper consent requests, Kelshiker told the clinical staff at Golborne
…
The Eko in clinical studies was able to detect about 85 per cent of treatable heart failure in patients. It had a 93 per cent specificity against the blood test, which means patients with an AI diagnosis almost always have an abnormal blood test as well.Razak, who has been using the Eko stethoscope for a few weeks, said the AI version feels less traditional in its design, compared with the others she has used, which might give older physicians reason to pause. It also requires a mobile device to pair with, and regular charging — extra steps she and others will have to get used to.
We are just getting started applying AI to health care. There are infinite possibilities to increase quality of care and people’s lifespan.
In the Advertising industry, the agency The Clueless perfected a series of synthetic influencers that are paid up to $1,000 a post.
Cristina Criddle, reporting for the Financial Times:
Pink-haired Aitana Lopez is followed by more than 200,000 people on social media. She posts selfies from concerts and her bedroom, while tagging brands such as haircare line Olaplex and lingerie giant Victoria’s Secret.
Brands have paid about $1,000 a post for her to promote their products on social media — despite the fact that she is entirely fictional.
Aitana is a “virtual influencer” created using artificial intelligence tools, one of the hundreds of digital avatars that have broken into the growing $21bn content creator economy.
…
Their emergence has led to worry from human influencers their income is being cannibalised and under threat from digital rivals. That concern is shared by people in more established professions that their livelihoods are under threat from generative AI — technology that can spew out humanlike text, images and code in seconds.But those behind the hyper-realistic AI creations argue they are merely disrupting an overinflated market.
“We were taken aback by the skyrocketing rates influencers charge nowadays. That got us thinking, ‘What if we just create our own influencer?’” said Diana Núñez, co-founder of the Barcelona-based agency The Clueless, which created Aitana. “The rest is history. We unintentionally created a monster. A beautiful one, though.”
Or, as Jeff Bezos famously said: “Your margin is my opportunity.”
Continuing:
Instagram analysis of an H&M advert featuring virtual influencer Kuki found that it reached 11 times more people and resulted in a 91 per cent decrease in cost per person remembering the advert, compared with a traditional ad.
…
“Influencers themselves have a lot of negative associations related to being fake or superficial, which makes people feel less concerned about the concept of that being replaced with AI or virtual influencers,” said Rebecca McGrath, associate director for media and technology at Mintel.“For a brand, they have total control versus a real person who comes with potential controversy, their own demands, their own opinions,” McGrath added.
…
The UK’s Advertising Standards Agency said it was “keenly aware of the rise of virtual influencers within this space” but said there was no rule where they must declare they are generated by AI.
Even if there were rules requiring disclosure, the new generation, exposed to synthetic content from a young age, wouldn’t care.
In Issue #22 – The Dawn of the Virtual YouTuber, we have seen in the first YouTube Culture & Trends Report that people are open to watching videos produced by synthetic avatars.
Continuing:
One of the first virtual influencers, Lil Miquela, charges up to hundreds of thousands of dollars for any given deal and has worked with Burberry, Prada and Givenchy.
Although AI is used to generate content for Lil Miquela, the team behind the creation “strongly believe [the storytelling behind virtual creators] cannot be fully replicated by generative AI”, said Ridhima Kahn, vice-president of business development at Dapper Labs, who oversees Lil Miquela’s partnerships.
Of course, this is only true for now. If you haven’t already, I suggest you read the “How Do You Feel?” section of this week’s Free Edition to learn about the latest research about large language models and creativity.
Continuing:
Lil Miquela is considered by many to be mixed race, and her audience of nearly 3mn followers ranges from the US to Asia and Latin America. Meanwhile, The Clueless now has another creation in development, which it calls a “curvy Mexican” named Laila.
…
Dapper Labs emphasised that the team behind Lil Miquela is diverse and reflects her audience. The Clueless said its creations were designed to “foster inclusivity and provide opportunities to collectives that have faced exclusion for an extended period”.The Clueless’s creations, among other virtual influencers, have also been criticised for being overly sexualised, with Aitana regularly appearing in underwear. The agency said sexualisation is “prevalent with real models and influencers” and that its creations “merely mirror these established practices without deviating from the current norms in the industry”.
The most phenomenal aspect of this is that, differently from The Clueless realistic synthetic influencers, Dapper Labs’ Lil Miquela is evidently synthetic:
and yet, this virtual influencer has more X followers than me. And I never worked with Burberry, Prada, and Givenchy.
I know. I’m doing everything wrong.
The key point here is that virtual influencers not only give brands full control over the content being promoted without risking PR disasters due to the uncontainable personality of a 20-something-year-old human who suddenly becomes rich. They also allow brands to check all the boxes of diversity and inclusion at a fraction of the cost (and the drama) of hiring real humans. And they allow to surgically craft personalities that resonate with any niche of the target audience, casting a net that is much wider than what a human influencer could ever reach.
If the market accepts synthetic influencers, the future for their human counterparts is bleak.
And if that happens, there’s no reason why synthetic influencers couldn’t start to proliferate in TV commercials, too.
In the Transportation industry, British Airways is using AI to predict when a plane will suffer a fault and anticipate maintenance.
Philip Georgiadis, reporting for the Financial Times:
Among new technologies is an AI tool that predicts when a plane is likely to develop a fault, allowing BA to pre-emptively carry out fixes rather than wait for failures to be spotted close to take off with passengers aboard.
This has already led to some operational improvements, one of the people said.
…
Senior executives accept BA is reliant on antiquated technologies, from a creaking IT network to using paper based records to log defects on flights.
…
BA has turned to older AI technologies before, including trying to speed up aircraft turnaround times on the ground by spotting potential problems on cameras fitted across the airfield at Heathrow airport.
According to LinkedIn comments from people directly involved in the project, this operation is funded by a £7 billion investment:
In the Defense Industry, the Chinese Ministry of State Security (MSS) is using AI to automatically identify and generate reports about counter-intelligence agents.
Edward Wong, Julian E. Barnes, Muyi Xiao and Chris Buckley, reporting for the New York Times:
The spies asked for an artificial intelligence program that would create instant dossiers on every person of interest in the area and analyze their behavior patterns. They proposed feeding the A.I. program information from databases and scores of cameras that would include car license plates, cellphone data, contacts and more.
The A.I.-generated profiles would allow the Chinese spies to select targets and pinpoint their networks and vulnerabilities, according to internal meeting memos obtained by The New York Times.
…
Today the Chinese agents in Beijing have what they asked for: an A.I. system that tracks American spies and others, said U.S. officials and a person with knowledge of the transaction, who shared the information on the condition that The Times not disclose the names of the contracting firms involved.
…
The M.S.S. has intensified its intelligence collection on American companies developing technology with both military and civilian uses, while the C.I.A., in a change from even a few years ago, is pouring resources into collecting data on Chinese companies developing A.I., quantum computing and other such tools.
As I said on social media at the very end of the 2023:
You won’t believe that people would fall for it, but they do. Boy, they do.
So this is a section dedicated to making me popular.
Companies adopting AI seek an edge by fine-tuning large language models to be exceptionally good at a few specific tasks.
You might remember BloombergGPT, possibly the first attempt by an end-user organization to train an LLM on proprietary data. We mentioned it in Issue #9 – The Tools of the Trade.
Months later, we start to see more deliberate efforts, especially in the Financial Services industry.
The first one of those efforts to pay attention to is called Shai, a large language model trained by a Chinese asset management company to perform tasks relevant to the firm.
From the research paper describing the project:
Our endeavor for building an AM LLM are as follows:
- First, we pick up and define several NLP tasks for a typical asset management company, and build the corresponding task dataset for training and evaluation.
- Second, we conduct continuous pretraining and supervised finetuning on a 10B-level base LLM model, providing an optimal balance between performance and inference cost.
- Third, we conduct evaluation covering our proposed AM tasks. These evaluations include financial professional examination questions, open Q&As based on real-world scenarios, specific tasks designed for asset management scenarios, and safety assessments, providing a comprehensive and objective evaluation. To gain valuable insights into the relative performance of these models in the specific context of asset management, we notably bring Shai into direct comparison with mainstream 10B-level open-source models, such as baichuan2[18], Qwen[19], InterLM[20], and Xverse[21], on our proprietary dataset.
So, what are the tasks that Shai is trained to focus on?
In investment research, for instance, LLMs can assist asset management firms in quickly and accurately extracting key information from a vast array of market data, financial reports, and macroeconomic indicators. They can analyze and summarize this complex information, enabling faster data collation and reducing errors that can occur due to human intervention.
In the realm of risk management, LLMs can aid asset management companies in predicting and evaluating various types of risks via sophisticated data analysis and pattern recognition. For example, when it comes to assessing the market volatility of a particular asset class, LLMs can swiftly analyze historical trends and relevant news reports, providing both quantitative and qualitative support to the risk assessment process.
In customer service and consultation, the application of LLMs has significantly improved the user interaction experience. They can comprehend the specific needs and situations of customers, providing targeted responses or recommendations, which greatly enhances customer satisfaction.
In the context of regulatory compliance, LLMs can interpret complex regulatory documents, assisting asset management companies in ensuring that their business operations meet a variety of legal requirements. For instance, when new financial regulations are introduced, LLMs can quickly summarize the main changes and potential impacts, helping the company adapt swiftly to changes in the legal environment.
Arguably, all generalist LLMS, like GPT-4, are already trained to reach the state of the art in performing all of the above tasks but the second. And GPT-5 might set a new bar that no specialized LLM can reach.
Caution must be exercised in deciding where to focus the training and fine-tuning efforts.
Once that is done, the next step is figuring out how to choose the right data to train the LLM on. Here, the paper offers precious advice:
In our process, our primary goal was to feed our model high-quality data from the asset management sector. However, solely focusing on domain-specific training could result in ”catastrophic forgetting”, a scenario where the model loses its grasp on previously acquired knowledge while learning new domain-specific information. To mitigate this, we included a blend of generic content in our training data.
…
During the pre-training phase, we selected a diverse range of data sources for model training, including textbooks from the financial and economic sector, research reports, interview records of fund managers, articles from official Chinese media outlets, and content from encyclopedias, books from various fields, and corpose from online forums.
…
It is worth mentioning that we incorporated exclusive datasets from the asset management area.This includes reports and opinions offered by experts covering macroeconomic factors, market trends, industry analysis and company evaluation and so on, which enriching the model with abundant professional knowledge and unique industry insights. Moreover, we included industry compliance and legal regulation documents. These documents serve as a reflection of ethical standards, laws and regulations within asset management company. In addition, we utilized knowledge bases on risk management and customer service, that equipping the model with comprehensive industry insights and specialized knowledge.However, we must acknowledge the potential errors during data processing, as both data parsing abilities and OCR systems may make mistakes. Moreover, online information can contain low-value content. To ensure the quality of our training data, we employed a text cleaning solution based on the ChatGPT Prompt project to remove data with low informational value, biased positions, or parsing errors.
…
Our data for Supervised Fine-tuning was divided into four parts: general dialogue, financial vertical Q&A, asset management tasks, and proprietary industry data.
OK. How did Shai perform compared to GPT-4 and other LLMs?
In this case, the answer is: not good enough, even against models with the same amount of parameters (which is much less than GPT-4), to justify the effort.
Also remember that scoring a LLM is very sensitive to the prompts used during the evaluation. We have many cases where, during an attempt to reproduce research findings, a poor GPT-4 performance was instantaneously improved with a different prompt.
There is no guarantee that a research team is great at prompt engineering.
The second effort comes from JP Morgan. It’s called DocLLM and it’s focused on a completely different challenge: a better understanding of corporate documents that include visual elements.
Documents with rich layouts, including invoices, receipts, contracts, orders, and forms, constitute a significant portion of enterprise corpora. The automatic interpretation and analysis of these documents offer considerable advantages, which has spurred the development of AI-driven solutions. These visually rich documents feature complex layouts, bespoke type-setting, and often exhibit variations in templates, formats and quality.
Although Document AI (DocAI) has made tremendous progress in various tasks including extraction, classification and question answering, there remains a significant performance gap in real-world applications. In particular, accuracy, reliability, contextual understanding and generalization to previously unseen domains continues to be a challenge.
Document intelligence is inherently a multi-modal problem with both the text content and visual layout cues being critical to understanding the documents. It requires solutions distinct from conventional large language models such as GPT-3.5, Llama, Falcon, or PaLM that primarily accept text-only inputs and assume that the documents exhibit simple layouts and uniform formatting, which may not be suitable for handling visual documents.
Numerous vision-language frameworks that can process documents as images and capture the interactions between textual and visual modalities are available. However, these frameworks necessitate the use of complex vision backbone architectures [9] to encode image information, and they often make use of spatial information as an auxiliary contextual signal.
In this paper we present DocLLM, a light-weight extension to standard LLMs that excels in several visually rich form
understanding tasks. Unlike traditional LLMs, it models both spatial layouts and text semantics, and therefore is intrinsically multi-modal. The spatial layout information is incorporated through bounding box coordinates of the text tokens obtained typically using optical character recognition (OCR), and does not rely on any vision encoder component. Consequently, our solution preserves the causal decoder architecture, introduces only a marginal increase in the model size, and has reduced processing times, as it does not rely on a complex vision encoder.We demonstrate that merely including the spatial layout structure is sufficient for various document intelligence tasks such as form
understanding, table alignment and visual question answering.
Just like for Shai, this focus area is at risk of being disrupted by GPT-4V and the future GPT-5V. We have a growing body of research demonstrating that GPT-4V can be trained to perform well on visual tasks, including OCR, and there’s no question that OpenAI and its competitors have a keen interest in using those types of models to increase semantic understanding and search of enterprise documents.
So, what are the tasks that DocLLM is trained to focus on?
We employ a total of 16 datasets with their corresponding OCRs, spanning four DocAI tasks: visual question answering (VQA), natural language inference (NLI), key information extraction (KIE), and document classification (CLS).
…
An example prompt derived from DocVQA would read: “{document} What is the deadline for scientific abstract submission for ACOG – 51st annual clinical meeting?”
…
An example prompt derived from TabFact would read: “{document} \”The UN commission on Korea include 2 Australians.\”, Yes or No?”
…
An example extraction instruction derived from KLC would read: “{document} What is the value for the \”charity number\”?”
…
An example MCQ instruction derived from RVL-CDIP would read: “{document} What type of document is this? Possible answers: [budget, form, file folder, questionnaire].”
And now, some information about the dataset used for training, to give you a sense of the scale of the effort:
We gather data for pre-training from two primary sources: IIT-CDIP Test Collection 1.0 and DocBank. IIT-CDIP Test Collection 1.0 encompasses a vast repository of over 5 million documents, comprising more than 16 million document pages. This dataset is derived from documents related to legal proceedings against the tobacco industry during the 1990s. DocBank consists of 500K documents, each featuring distinct layouts and a single page per document. The relevant statistics for the datasets utilized in the pre-training are detailed in Table 2. We obtain a collection of 16.7 million pages comprising a total of 3.8 billion tokens.
Interestingly, the JPMorgan team created two variants of DocLLM: one adopting the Falcon architecture and one adopting the LLaMA-2 architecture.
How did they perform against GPT-4 and other LLMs?
Even here, the performance is inconsistently better and, in some cases, not so much better to justify the enormous effort of training a specialized LLM.
More importantly, this evaluation doesn’t test the performance of GPT-4V, leaving doubts about how long the advantage of DocLLM will last in those tasks where it currently shows a lead.
If your company is trying to reinvent the wheel to gain an edge, keep in mind that incremental improvements to make the wheel run smoother are not meant to guarantee a long-term competitive advantage.
If you or your company are evaluating the adoption of a large language model for a specific use case, you might find useful doing some preliminary research on an online free service called Chatbot Arena.
The intended purpose of this tool, developed by the research lab LMSYS Org, is to benchmark the performance of commercial, open access, and open source large language models in a way that takes into account human preferences much more than the traditional metrics used by benchmarks like GSM8K.
By default, the user sends a prompt and the system returns the output of two LLMs, without revealing which one is which. Only after the user has indicated which output he/she prefers, the name of the LLMs is revealed:
The user can continue rating the outputs until he/she is satisfied and can clearly identify which LLM is the best for the intended use case.
Research teams all around the world compete to have their LLMs reach the top of the Arena leaderboard, a bit like a group of parents arguing about whose child is the smartest in the class. And, so far, the whole AI community happily fomented this competition with over 100,000 votes.
But if you put aside the original intent of the project, you’ll see that this tool can also be used to assess how promising contestants respond to user prompts in edge use cases compared to generalist LLMs like GPT-4 or LLaMA-2.
While Chatbot Arena doesn’t offer the possibility to compare every single LLM released by the AI community (there are hundreds), it gives you access to the most important and capable ones. The same ones you’d probably consider for a production project:
To make things easier in this particular scenario, LMSYS Org offers an alternative modality (called Arena side-by-side) where you can pick which LLMs to compare in a non-blind test:
This capability of comparing the answers of multiple LLMs is something I already praised in a very powerful AI system called h2oGPT, which we reviewed in Issue #35 – X-Rays for Vans.
But h2oGPT is very complex to set up and use, and adding new models to test requires a lot of technical expertise.
Conversely, Chatbot Arena is effortless even for a non-technical person like a Line of Business manager.
The only downside of Chatbot Arena when used for testing edge prompts and use cases is that, differently from an AI system like h2oGPT, it doesn’t allow you to have a full conversation with the LLMs under evaluation. You can only send a single prompt and get a single response, but you can’t continue that session.
Due to this limitation, think about Chatbot Arena as a tool to quickly triage the possible directions of your evaluation before you commit to a more expensive and time-consuming analysis.
Happy experimenting!