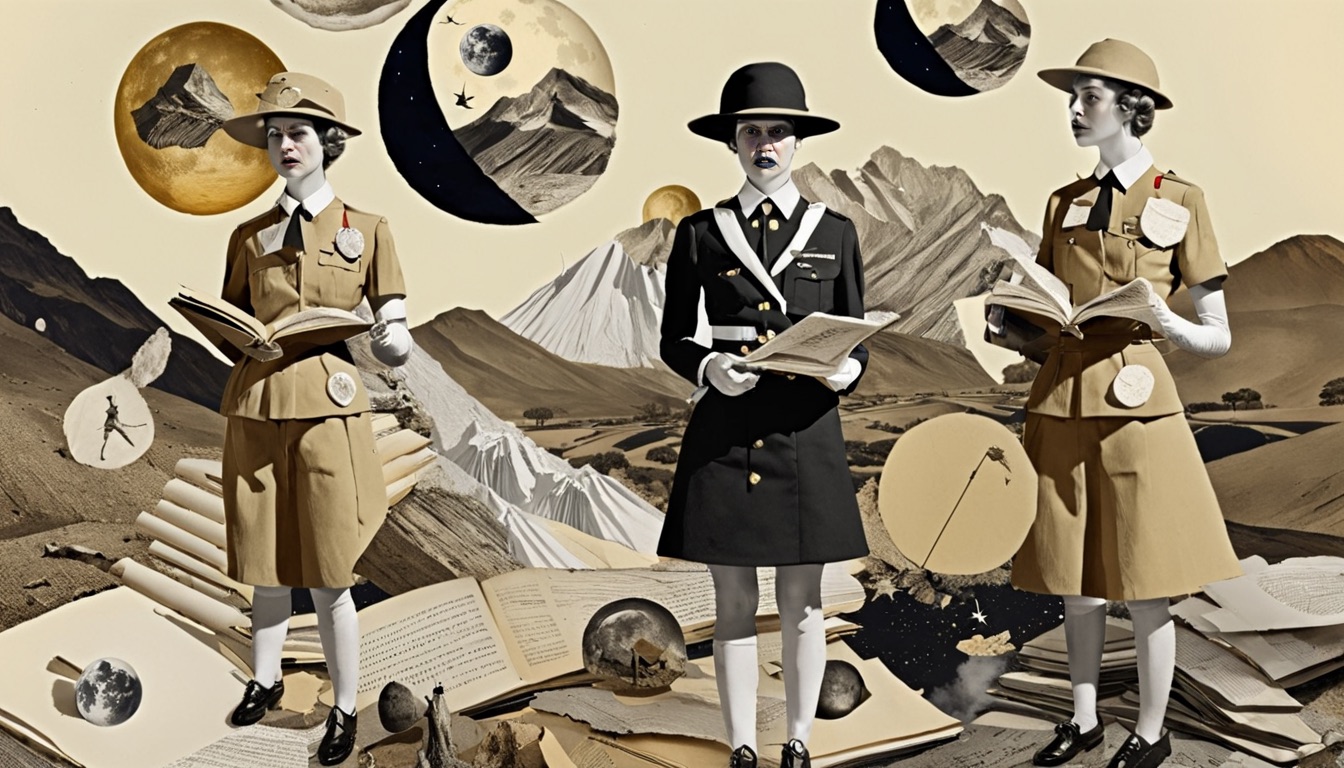
- What’s AI Doing for Companies Like Mine?
- Learn what Bloomberg, Umicore, and Etsy are doing with AI.
- A Chart to Look Smart
- In 2023, Meta hoarded as many NVIDIA GPUs as Microsoft. Probably to train LLaMA 3.
- Most popular AI services on the market collected 24B visitors in less than a year, suggesting an enormous opportunity ahead.
- MedARC compared the medical knowledge of open access and proprietary LLMs, finding surprising results.
- Google DeepMind researchers fine-tuned an LLM that outperforms doctors in differential diagnosis.
- Prompting
- How you format your prompt matters. For some LLMs, it matters a lot.
What we talk about here is not about what it could be, but about what is happening today.
Every organization adopting AI that is mentioned in this section is recorded in the AI Adoption Tracker.
In the Financial Services industry, Bloomberg started to show AI-generated summaries of earnings calls in its famed Bloomberg Terminal.
Ted Merz describes what he saw on LinkedIn:
Clients of the terminal can now create AI summaries of conference call transcripts.
A friend who is a money manager walked me through a demo showing how summaries are accessible via the Document Search function.
The feature is a small, but significant development for financial professionals.
It illustrates both the opportunity and challenge of integrating generative AI into terminals used by more than 325,000 Wall Street traders and investors.
Large legacy data providers such as Bloomberg, FactSet and others are trying to balance the opportunity of incorporating the ChatGPT technology that OpenAI unveiled in late 2022 with the risks that it produces hallucinations.
Bloomberg has taken an incremental approach. The new summaries are delivered from hard-coded prompts on a narrow range of documents. Unlike ChatGPT, the system doesn’t allow users to submit follow up questions.
Here’s how it works: Using the Document Search function clients can select a company and then any of the recent documents that display a summary icon.
The summary has predefined categories including guidance, capital allocation, labor, the macro environment, supply chain, pricing and product development.
It’s easy to use and includes a nice feature: when you click on any of the sections it links back to the original transcript to provide transparency.
There appears to be no way to ask follow-up questions, which is one of the defining features of ChatGPT. It doesn’t tap into the broader Bloomberg database for numbers and news. Nor can the summary handle multiple documents at the same time to identify longer-term trends.
The summaries also don’t include the questions asked by analysts.
So, you cannot ask: “What are the biggest issues Tesla faced in the past quarter? Or what did the analysts ask Apple CEO Tim Cook? Or did Google talk about its business in Europe?
An official press release from Bloomberg confirms the features:
AI-Powered Earnings Call Summaries is designed with a unique approach that combines decades of domain expertise with state-of-the-art, generative AI. Bloomberg Intelligence analysts help train the large language models used in this solution to more accurately understand the nuances of financial language and anticipate what’s most important to investors.
Summary points are also enriched with context links so analysts can discover related information across the Terminal in functions such as Company Financials {MODL
}, Bloomberg Dividend Forecast {BDVD } and Supply Chain Analysis {SPLC }. For more transparency, the solution also makes it possible for users to click on each point in the summary sidebar to easily jump to the corresponding excerpts in the call transcript.
The feature seems similar to one of the many I described in Issue #42 – Say Hi to your future synthetic financial analyst when I reviewed the upcoming OpenBB Pro terminal.
Unfortunately, I don’t have access to a Bloomberg Terminal to compare the two approaches. I’d say that, in both cases, we are looking at a very early implementation of what will likely become a table stake feature in the next few years.
In the Materials industry, Umicore is testing AI to discover and commercialize new battery materials.
Harry Dempsey, reporting for Financial Times:
Mathias Miedreich, chief executive of Belgium’s Umicore, which is working with Microsoft, said that AI would have an “immense impact” on discovering and commercialising new battery materials.
In an interview with the Financial Times, he said: “The ambition is, when you have a certain product that you want to develop, to cut the time to market by half…we are doing now the first concrete projects to see if this is feasible.”
…
For the past two years, Umicore, supplied materials for car batteries used by companies such as Volkswagen, BMW and Stellantis, whose brands include Chrysler, Fiat and Peugeot.He expected AI to help cut the research stage in developing new battery materials from five or six years to two years.
…
AI looked most promising for breakthroughs for solid-state materials and disorder rock salts, which would drastically improve the driving range of an electric car and open up new applications for batteries
…
Battery producers are optimistic of AI revolutionising the discovery of new materials, emulating a shift seen in the pharmaceutical industry where the technology is being used to speed up the development of new drugs.
…
“It’s like a recipe with multiple ingredients and we want to find out if the absolute ingredient is the right one or in the right proportion. This is a massive space to explore. With AI, you can start to expand the comfort zone of where you look.”Earlier this month, Microsoft said that its work with the Pacific Northwest National Laboratory in the US showed that its algorithm was able to narrow 32mn materials down to 18 promising candidates to use in batteries in just 80 hours.
The government-backed laboratory is testing one of those materials, a hybrid of lithium and sodium, that could reduce lithium content by as much as 70 per cent — a material that automakers are worried will be scarce.
…
Miedreich cautioned that there might not be enough available computing power to make all the breakthroughs. And even if suitable materials are identified, researchers must test them in real-world conditions to discover whether they perform as hoped. The safety of batteries produced with new substances was also critical, meaning testing time was difficult to compress, said executives.
The discovery of new materials via molecular simulation and other techniques is as fascinating as the discovery of new drugs.
For now, quantum computing is more of a promise than a reality, but perhaps, one day, we’ll be able to harness its power so that AI can help us discover new materials and new drugs in weeks, and not years.
That would truly start a new golden era for humanity.
In the E-Commerce industry, Etsy is using AI to help shoppers find gift ideas.
Lauren Forristal, reporting for TechCrunch:
Gift Mode is essentially an online quiz that asks about who you’re shopping for (sibling, parent, child), the occasion (birthday, anniversary, get well), and the recipient’s interests. At launch, the feature has 15 interests to choose from, including crafting, fashion, sports, video games, pets, and more. It then generates a series of gift guides inspired by your choices, pulling options from the over 100 million items listed on the platform. The gift guides are centered around more than 200 different personas.
For instance, “The Music Lover,” “The Video Gamer,” “The Adventurer” and “The Pet Parent.” Over time, the company plans to add new interests and personas in response to “emerging trends,” Tim Holley, Etsy’s VP of Product, told TechCrunch.
…
Online retailer UncommonGoods did something similar in 2017 when it released “Sunny,” its AI-powered gift guide feature that suggests products based on inputs like who you’re shopping for, their age group, and hobbies they’re interested in. There’s also a text box to add more specific preferences.
This is a perfect example of gimmick AI.
Going for the low-hanging fruit might seem like a good strategy, but it’s almost never the move that makes a substantial difference for a company in the long run.
There is a difference between solving a ridiculously obvious problem that everybody has, and that shouldn’t exist in the first place, with a difficult-to-implement technological approach, and solving a problem that nobody has with a ridiculously simple technological approach.
How about, instead of this, a way for the users to describe in detail an object that may or may not exist in the Etsy marketplace and the AI finds the closest object for sale on the platform?
You won’t believe that people would fall for it, but they do. Boy, they do.
So this is a section dedicated to making me popular.
In 2023, Meta ordered as many NVIDIA GPUs as Microsoft. This is the sheer size of the shipment, according to the analysts:
Assuming that Microsoft splits its GPUs between OpenAI and the rest of Azure customers, Meta might have significantly more GPUs available to train LLaMA 3 than OpenAI did for the training of GPT-4.
Mark Zuckerberg has been clear that his company has shifted focus, temporarily, I’m sure, from the metaverse to AI.
Should Meta release LLaMA 3 under a real open source license, it would trigger a wave of in-house solutions across thousands of large enterprise organizations across industries that today have no real choice but to adopt OpenAI.
However, it seems an unlikely scenario considering the history of the company, the attitude of the founder, and the recent behavior.
A more likely scenario: Zuckerberg was persuaded that AGI is attainable. And he must have concluded that building “Her” and pushing it to every single user across Facebook, Whatsapp, and Instagram is infinitely more lucrative than what Meta is doing now with traditional advertising.
As I said many times on Synthetic Work, LLMs are the ultimate advertising vehicle. And you don’t even the next generation for that, as Microsoft has already shown with its ad-peppered Bing AI answers.
Speaking of large language models and chatbots (the two things are not equivalent), you might wonder how big is the opportunity out there. The answer is: enormous.
Let’s put aside the unfathomable number of people who visited the ChatGPT website between September 2022 and August 2023.
Character AI, occasionally mentioned here on Synthetic Work, had 3.8 billion visits in the same period.
If you read this week’s Free Edition of this newsletter, you discovered that new research discovered that the users of these chatbots tend to be a little more lonely than the average. So, the question that comes to mind is: how many lonely people are there in the world?
And what’s the opportunity to help and to make money attached to that?
Other notable websites you should pay attention to:
- QuillBot, which is the number one tool used by students worldwide to paraphrase ChatGPT output and evade AI detectors.
- Midjourney, which is considered the state of the art in image generation and it’s still inaccessible to most due to their decision to build on top of Discord.
- Civitai, which is considered the must-visit website for people looking for fine-tuned versions of Stable Diffusion.
- ElevenLabs, often mentioned in this newsletter for their second-most realistic synthetic voices on the market.
- Claude AI which, despite being painted as the most likely competitor to OpenAI, fails to attract an audience even remotely close to the one visiting all the other websites I just mentioned.
For some of these companies, these numbers will go up significantly in the next few months. For example, Midjourney will explode in popularity later this year, when they finally abandon Discord for a more traditional web interface.
For others, their huge audience will only translate into a weight that will crush the company. For example, I have reasons to believe that Civitai is struggling financially due to the growing costs.
Regardless, a huge number of people still have no idea about any of these tools, and the ones that will enter the market later this year.
This is just the beginning of the opportunity.
One of the subsidiary R&D labs of Stability AI, MedARC, compared the medical knowledge of open access and proprietary large language models, finding surprising results.
In order to develop the best open medical LLMs, we need to be able to evaluate our progress. Currently, the evaluation of LLMs on medical tasks is most commonly done on MultiMedQA, a suite of medical question-answering tasks introduced by Google in the Med-PaLM paper. MultiMedQA includes tasks like PubMedQA, MedQA, MMLU clinical knowledge, etc., which evaluate medical knowledge and reasoning. As of January 2024, prompt-engineered GPT-4 is SOTA on all tasks in MultiMedQA.
While closed-source generalist LLMs like PaLM and GPT-4 have been thoroughly evaluated on medical tasks in MultiMedQA and on other benchmarks, there has been minimal evaluation of generalist open LLMs on medical tasks. Benchmarking open source LLMs is the first step toward selecting which models to use and possibly fine-tune for medical applications. Therefore, we evaluate a variety of SOTA generalist open LLMs (Llama-2, Mistral, Yi, etc.) on MultiMedQA to see how well they stack up against proprietary LLMs.
…
We have evaluated a total of 22 models on MultiMedQA. This includes models like Llama-2, Yi, Mistral, StableLM, and Qwen models. This also includes one domain-specific fine-tuned model, Meditron-70b, which is one of the best open-source medical LLMs.
Overall, there is a clear winner: Qwen-72b is the best open medical LLM on medical reasoning benchmarks in the zero-shot setting. It is within 9.7% of Med-PaLM 2’s performance and 13.8% of GPT-4’s performance. On some tasks, open models are closing in on the SOTA (PubMedQA, MMLU college biology). However, current open base models still remain inferior to GPT-4 and Med-PaLM 2 but are better than Med-PaLM on all tasks except MedQA. Note that Med-PaLM, Med-PaLM 2, and GPT-4 use sophisticated prompting techniques, while we only test simple zero- or five-shot prompting.
In other words, Google’s first generation of its multi-million dollar specialized LLM, could barely compete with an open access, generalist AI model developed by the Chinese researchers of Alibaba Cloud. And without the help of any particular prompting technique.
Incidentally, Qwen-72b remains the top pre-trained LLM in the Hugging Face leaderboard. Based on it, the AI community created a host of fine-tuned and chat-focused models.
And, of course, one of these fine-tuned variants of Qwen-72b, could well be one fine-tuned on medical knowledge.
Meditron-70b, mentioned above, for example, is a fine-tuned version of LLaMA 2.
What does all of this mean?
It means that if your company is exploring the idea of building an AI-powered commercial solution for the Healthcare industry, by the end of this year, reliance on OpenAI might not be your only option.
Speaking of LLMs fine-tuned with medical knowledge, Google and DeepMind researchers published new research testing the performance of a version of PaLM-2 fine-tuned to perform differential diagnosis.
From the paper, titled Towards Accurate Differential Diagnosis with Large Language Models:
An accurate differential diagnosis (DDx) is a cornerstone of medical care, often reached through an iterative process of interpretation that combines clinical history, physical examination, investigations and procedures. Interactive interfaces powered by Large Language Models (LLMs) present new opportunities to both assist and automate aspects of this process.
In this study, we introduce an LLM optimized for diagnostic reasoning, and evaluate its ability to generate a DDx alone or as an aid to clinicians.
20 clinicians evaluated 302 challenging, real-world medical cases sourced from the New England Journal of Medicine (NEJM) case reports. Each case report was read by two clinicians, who were randomized to one of two assistive conditions: either assistance from search engines and standard medical resources, or LLM assistance in addition to these tools.
All clinicians provided a baseline, unassisted DDx prior to using the respective assistive tools. Our LLM for DDx exhibited standalone performance that exceeded that of unassisted clinicians (top-10 accuracy 59.1% vs 33.6%, [p = 0.04]).
Comparing the two assisted study arms, the DDx quality score was higher for clinicians assisted by our LLM (top-10 accuracy 51.7%) compared to clinicians without its assistance (36.1%) (McNemar’s Test: 45.7, p < 0.01) and clinicians with search (44.4%) (4.75, p = 0.03). Further, clinicians assisted by our LLM arrived at more comprehensive differential lists than those without its assistance. Our study suggests that our LLM for DDx has potential to improve clinicians' diagnostic reasoning and accuracy in challenging cases, meriting further real-world evaluation for its ability to empower physicians and widen patients' access to specialist-level expertise.
For some reason, the researchers didn’t use Med-PaLM 2 for this test, but fine-tuned an alternative LLM, starting from the PaLM 2 architecture.
The results are impressive and the research, not too dense, is fascinating to read.
The conclusions:
In standalone performance, the LLM generated more appropriate and comprehensive DDx lists than physicians when they were unassisted, with its DDx lists more likely to include the final diagnosis than DDx lists from a board-certified internal medicine physician.
…
Clinicians using the LLM as an assistant produced a DDx with higher top-N accuracy, and DDx with greater quality, appropriateness and comprehensiveness; compared to the status quo for clinical practice (use of Internet search and other
resources).
…
While clinicopathological conferences (CPCs) have long been used as benchmarks for difficult diagnosis, it is also well-known that performance in CPCs in no way reflects a broader measure of competence in a physician’s duties.Furthermore, the act of DDx comprises many other steps that were not scrutinized in this study, including the goal-directed acquisition of information under uncertainty (known to be challenging for AI systems despite recent technical progress in this direction).
While based on real-world cases, the clinical pathology case presentation format and input into the model does differ in important ways from how a clinician would evaluate a patient and generate their DDx at the outset of a clinical encounter.
For example, while the case reports are created as “puzzles” with enough clues that should enable a specialist to reason towards the final diagnosis, it would be challenging to create such a concise, complete and coherent case report at the beginning of a real clinical encounter.
We are therefore very cautious to extrapolate our findings toward any implications about the LLM’s utility as a standalone diagnostic tool.
…
Our work extends prior observations by showing not only that the LLM was more likely to arrive at a correct answer or provide the correct answer in a list, but that its DDx were determined by an independent rater to be of higher appropriateness and comprehensiveness than those produced by board certified physicians with access to references and search.
…
Clinicians frequently expressed excitement about using the LLM but were also aware of the shortcomings of language models and had concerns about confabulations in particular if used by individuals not trained or instructed to avoid such questions. However, our work did not explore many other important aspects of human-AI interaction, which require further study in safety-critical settings such as this.For example, we did not explore the extent to which clinicians trusted the outputs of the model or their understanding of its training and limitations, or undertake focused “onboarding” or training in its use, which are all known to be important modifiers of the benefits derived by clinicians from AI assistants.
…
Qualitative feedback suggested that there remains room for targeted improvement of LLMs as assistive diagnostic tools, with one clinician noting that “It was most helpful for simpler cases that were specific keywords or pathognomonic signs.” but for more complex cases it still tended to draw conclusions from isolated symptoms rather than viewing the case holistically.The assistive effect of these LLMs could potentially ‘upskill’ clinical providers, particularly in enabling them to broaden and enhance the quality of their DDx. As corroborated via our clinician interviews after their experience with the LLM, such upskilling could be relevant for education or training purposes to support providers across a skill continuum ranging from trainees to attending providers.
The upskilling capabilities could also extend to locations where specialist medical training is less common (e.g., in lower and middle income countries [LMIC]).
Assume that LLMs will continue to drastically improve their comprehension and manipulation of the human language with every new generation.
Assume that these LLMs will also gain the capability to infer valuable information from additional senses (sight, hearing – where hearing is not just listening to what the patient says, but hearing the tone of their voice, the pace of their breathing, the sound of the heartbeat, etc.) with every new generation.
When you couple this with the story you read in last week’s Issue #45 – DeathGPT, you really have a feeling that the future of healthcare is going to be drastically different from what we have today.
Before you start reading this section, it's mandatory that you roll your eyes at the word "engineering" in "prompt engineering".
Filed under “You must be kidding me,” new research shows that the way we format our prompts for large language models like GPT-3.5-Turbo and LLaMA 2 matters. In some cases, it matters a lot.
The implications of this is not just that we can get better results from our models if we experiment a bit in the way we express ourselves, but also that the existing benchmarks for these models are probably terribly misleading.
From the paper, titled Quantifying Language Models’ Sensitivity To Spurious Features In Prompt Design Or: How I Learned To Start Worrying About Prompt Formatting
As large language models (LLMs) are adopted as a fundamental component of language technologies, it is crucial to accurately characterize their performance. Because choices in prompt design can strongly influence model behavior, this design process is critical in effectively using any modern pre-trained generative language model. In this work, we focus on LLM sensitivity to a quintessential class of meaning-preserving design choices: prompt formatting. We find that several widely used open-source LLMs are extremely sensitive to subtle changes in prompt formatting in few-shot settings, with performance differences of up to 76 accuracy points when evaluated using LLaMA-2-13B. Sensitivity remains even when increasing model size, the number of few-shot examples, or performing instruction tuning. Our analysis suggests that work evaluating LLMs with prompting-based methods would benefit from reporting a range of performance across plausible prompt formats, instead of the currently-standard practice of reporting performance on a single format. We also show that format performance only weakly correlates between models, which puts into question the methodological validity of comparing models with an arbitrarily chosen, fixed prompt format.
In other words, if you use a space to separate certain portions of your prompt or you go on a new line, it makes a difference. And to find out what combination yields the most accurate answer, these researchers have created an automation script.
The researchers tested LLaMA-2-{7B,13B,70B}, Falcon-7B and Falcon-7B Instruct, and GPT-3.5-Turbo. No GPT-4 yet, but if you have seen recent prompt examples shared by OpenAI, you may have noticed that they, too, format their prompts in multiple ways.
For example, they use all uppercase words for things that they want to remark, like DO NOT, or square brackets to identify roles or users. It’s not by accident.
This research team discovered that the LLM accuracy is influenced by the prompt format depending on the task. The same formatting approach doesn’t necessarily have the same effect on all tasks.
And, depending on the task, sometimes the presence of a deliberate prompt format makes a big difference, while other times it makes a negligible difference.
For example:
As you can see, hopefully, apparently there’s a difference between keeping everything on the same line and putting certain terms on a new line followed by a tab. Who knew?
Look. The paper is very dense, and it’s not exactly easy to understand the formatting they used.
None of us will ever remember the intricacies of prompt formatting for this or that task. That said:
- If you are building a commercial chatbot or an employee-facing one, you might want to dedicate some quality testing time to explore the effect of prompt formatting on the accuracy of your LLM answers.
- If you are just interacting with an LLM inside ChatGPT or LM Studio, try to follow your instinct and give your prompt a little bit of flare, with uppercases, new lines, or brackets where it seems it makes sense.
I started formatting the prompts I use to run certain portions of Synthetic Work (like the Breaking News section) a few months ago and it does make a difference.